Open Access
ARTICLE
Towards Generating a Practical SUNBURST Attack Dataset for Network Attack Detection
1 Princess Summaya University for Technology, Amman, Jordan
2 College of Computing and IT, Shaqra University, Shaqra, Saudi Arabia
* Corresponding Author: Amjad Aldweesh. Email:
Computer Systems Science and Engineering 2023, 47(2), 2643-2669. https://doi.org/10.32604/csse.2023.040626
Received 25 March 2023; Accepted 13 June 2023; Issue published 28 July 2023
Abstract
Supply chain attacks, exemplified by the SUNBURST attack utilizing SolarWinds Orion updates, pose a growing cybersecurity threat to entities worldwide. However, the need for suitable datasets for detecting and anticipating SUNBURST attacks is a significant challenge. We present a novel dataset collected using a unique network traffic data collection methodology to address this gap. Our study aims to enhance intrusion detection and prevention systems by understanding SUNBURST attack features. We construct realistic attack scenarios by combining relevant data and attack indicators. The dataset is validated with the J48 machine learning algorithm, achieving an average F-Measure of 87.7%. Our significant contribution is the practical SUNBURST attack dataset, enabling better prevention and mitigation strategies. It is a valuable resource for researchers and practitioners to enhance supply chain attack defenses. In conclusion, our research provides a concise and focused SUNBURST attack dataset, facilitating improved intrusion detection and prevention systems.Keywords
Cite This Article
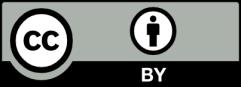