Open Access
ARTICLE
Intelligent Intrusion Detection System for the Internet of Medical Things Based on Data-Driven Techniques
1 Faculty of Computers and Information Technology, University of Tabuk, Tabuk, 71491, Saudi Arabia
2 National Engineering School of Monastir, University of Monastir, Monastir, 5000, Tunisia
3 Applied College, University of Tabuk, Tabuk, 71491, Saudi Arabia
4 Department of Electronics, Faculty of Engineering Annaba, Badji Mokhtar BP. 12, Annaba, 23000, Algeria
* Corresponding Author: Okba Taouali. Email:
Computer Systems Science and Engineering 2023, 47(2), 1593-1609. https://doi.org/10.32604/csse.2023.039984
Received 27 February 2023; Accepted 27 April 2023; Issue published 28 July 2023
Abstract
Introducing IoT devices to healthcare fields has made it possible to remotely monitor patients’ information and provide a proper diagnosis as needed, resulting in the Internet of Medical Things (IoMT). However, obtaining good security features that ensure the integrity and confidentiality of patient’s information is a significant challenge. However, due to the computational resources being limited, an edge device may struggle to handle heavy detection tasks such as complex machine learning algorithms. Therefore, designing and developing a lightweight detection mechanism is crucial. To address the aforementioned challenges, a new lightweight IDS approach is developed to effectively combat a diverse range of cyberattacks in IoMT networks. The proposed anomaly-based IDS is divided into three steps: pre-processing, feature selection, and decision. In the pre-processing phase, data cleaning and normalization are performed. In the feature selection step, the proposed approach uses two data-driven kernel techniques: kernel principal component analysis and kernel partial least square techniques to reduce the dimension of extracted features and to ameliorate the detection results. Therefore, in decision step, in order to classify whether the traffic flow is normal or malicious the kernel extreme learning machine is used. To check the efficiency of the developed detection scheme, a modern IoMT dataset named WUSTL-EHMS-2020 is considered to evaluate and discuss the achieved results. The proposed method achieved 99.9% accuracy, 99.8% specificity, 100% Sensitivity, 99.9 F-score.Keywords
Cite This Article
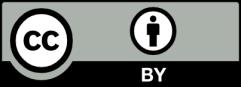