Open Access
ARTICLE
Diagnosis of Disc Space Variation Fault Degree of Transformer Winding Based on K-Nearest Neighbor Algorithm
1 Department of Power and Electrical Engineering, Northwest A&F University, Yangling, 712100, China
2 NARI Group, Beijing Kedong Electric Power Control System Co., Ltd., Beijing, 100000, China
3 Huangling Mining Group Co., Ltd., Shanxi Coal and Chemical Industry Group, Huangling, 716000, China
* Corresponding Author: Song Wang. Email:
(This article belongs to the Special Issue: Advances in Modern Electric Power and Energy Systems)
Energy Engineering 2023, 120(10), 2273-2285. https://doi.org/10.32604/ee.2023.030107
Received 22 March 2023; Accepted 01 June 2023; Issue published 28 September 2023
Abstract
Winding is one of the most important components in power transformers. Ensuring the health state of the winding is of great importance to the stable operation of the power system. To efficiently and accurately diagnose the disc space variation (DSV) fault degree of transformer winding, this paper presents a diagnostic method of winding fault based on the K-Nearest Neighbor (KNN) algorithm and the frequency response analysis (FRA) method. First, a laboratory winding model is used, and DSV faults with four different degrees are achieved by changing disc space of the discs in the winding. Then, a series of FRA tests are conducted to obtain the FRA results and set up the FRA dataset. Second, ten different numerical indices are utilized to obtain features of FRA curves of faulted winding. Third, the 10-fold cross-validation method is employed to determine the optimal k-value of KNN. In addition, to improve the accuracy of the KNN model, a comparative analysis is made between the accuracy of the KNN algorithm and k-value under four distance functions. After getting the most appropriate distance metric and k-value, the fault classification model based on the KNN and FRA is constructed and it is used to classify the degrees of DSV faults. The identification accuracy rate of the proposed model is up to 98.30%. Finally, the performance of the model is presented by comparing with the support vector machine (SVM), SVM optimized by the particle swarm optimization (PSO-SVM) method, and random forest (RF). The results show that the diagnosis accuracy of the proposed model is the highest and the model can be used to accurately diagnose the DSV fault degrees of the winding.Graphical Abstract
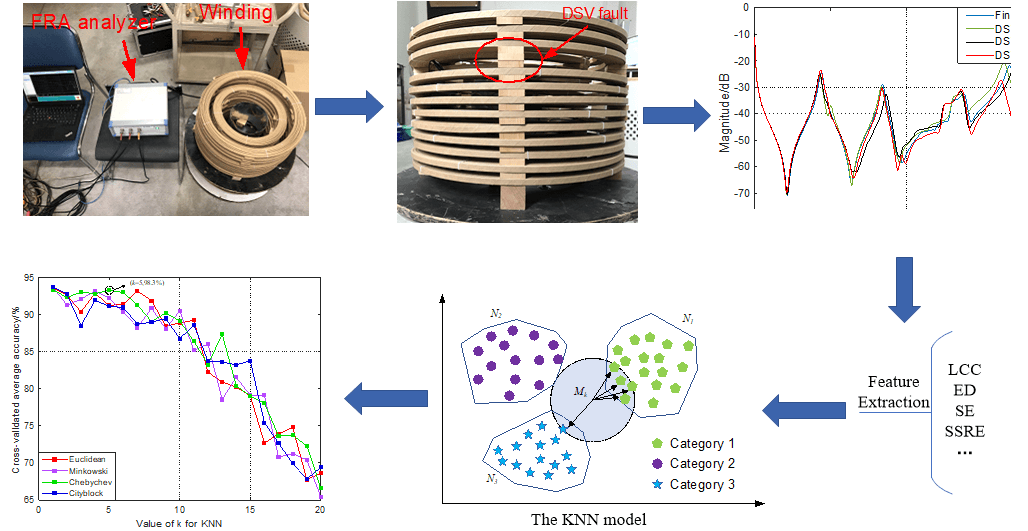
Keywords
Cite This Article
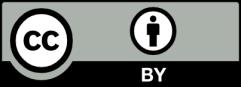