Open Access
ARTICLE
A Study of Unmanned Path Planning Based on a Double-Twin RBM-BP Deep Neural Network
1 Zhejiang Industry Polytechnic College, Shaoxing, 312000, China
2 Kunsan National University, Jeollabuk-do, 54150, Korea
* Corresponding Author: Xuan Chen. Email:
Intelligent Automation & Soft Computing 2020, 26(6), 1531-1548. https://doi.org/10.32604/iasc.2020.011723
Received 26 May 2020; Accepted 27 August 2020; Issue published 24 December 2020
Abstract
Addressing the shortcomings of unmanned path planning, such as significant error and low precision, a path-planning algorithm based on the whale optimization algorithm (WOA)-optimized double-blinking restricted Boltzmann machine-back propagation (RBM-BP) deep neural network model is proposed. The model consists mainly of two twin RBMs and one BP neural network. One twin RBM is used for feature extraction of the unmanned path location, and the other RBM is used for the path similarity calculation. The model uses the WOA algorithm to optimize parameters, which reduces the number of training sessions, shortens the training time, and reduces the training errors of the neural network. In the MATLAB simulation experiment, the proposed algorithm is superior to several other neural network algorithms in terms of training errors. The comparison of the optimal path under the simulation of complex road conditions shows the superior performance of this algorithm. To further test the performance algorithm introduced in this paper, a flower bed, computer room and other actual scenarios were chosen to conduct path-planning experiments for unmanned paths. The results show that the proposed algorithm has obvious advantages in path selection, reducing the running time and improving the running efficiency. Therefore, it has definitive practical value in unmanned driving.Keywords
Cite This Article
X. Chen, Z. Wan and J. Wang, "A study of unmanned path planning based on a double-twin rbm-bp deep neural network," Intelligent Automation & Soft Computing, vol. 26, no.6, pp. 1531–1548, 2020. https://doi.org/10.32604/iasc.2020.011723Citations
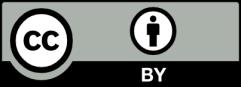