Open Access
ARTICLE
Short-Term Stock Price Forecasting Based on an SVD-LSTM Model
1 Department of Finance and Taxation, Shandong University of Finance and Economics, Jinan, 250014, China
2 Department of Computer science and Technology, Shandong University of Finance and Economics, Jinan, 250014, China
* Corresponding Author: Peiguang Lin. Email:
Intelligent Automation & Soft Computing 2021, 28(2), 369-378. https://doi.org/10.32604/iasc.2021.014962
Received 29 October 2020; Accepted 04 February 2021; Issue published 01 April 2021
Abstract
Stocks are the key components of most investment portfolios. The accurate forecasting of stock prices can help investors and investment brokerage firms make profits or reduce losses. However, stock forecasting is complex because of the intrinsic features of stock data, such as nonlinearity, long-term dependency, and volatility. Moreover, stock prices are affected by multiple factors. Various studies in this field have proposed ways to improve prediction accuracy. However, not all of the proposed features are valid, and there is often noise in the features—such as political, economic, and legal factors—which can lead to poor prediction results. To overcome such limitations, this study proposes a forecasting model for predicting stock prices in a short-term time series. First, we use singular value decomposition (SVD) to reconstruct the features of stock data, eliminate data noise, retain the most effective data features, and improve the accuracy of prediction. We then model the time-series stock data based on a long short-term memory (LSTM) model. We compare our proposed SVD-LSTM model with four state-of-the-art methods using real-world stock datasets from two Chinese banks: Ping an Bank and Shanghai Pudong Development Bank. The experimental results show that the proposed method can improve the accuracy of stock price predictions.Keywords
Cite This Article
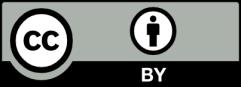