Open Access
ARTICLE
Time-Efficient Fire Detection Convolutional Neural Network Coupled with Transfer Learning
Department of Computer Sciences, College of Computer and Information Sciences, Princess Nourah bint Abdulrahman University, Riyadh, 11047, KSA
* Corresponding Author: Norah S. Alghamdi. Email:
Intelligent Automation & Soft Computing 2022, 31(3), 1393-1403. https://doi.org/10.32604/iasc.2022.020629
Received 01 June 2021; Accepted 15 July 2021; Issue published 09 October 2021
Abstract
The detection of fires in surveillance videos are usually done by utilizing deep learning. In Spite of the advances in processing power, deep learning methods usually need extensive computations and require high memory resources. This leads to restriction in real time fire detection. In this research, we present a time-efficient fire detection convolutional neural network coupled with transfer learning for surveillance systems. The model utilizes CNN architecture with reasonable computational time that is deemed possible for real time applications. At the same time, the model will not compromise accuracy for time efficiency by tuning the model with respect to fire data. Extensive experiments are carried out on real fire data from benchmarks datasets. The experiments prove the accuracy and time efficiency of the proposed model. Also, validation of the model in fire detection in surveillance videos is proved and the performance of the model is compared to state-of-the-art fire detection models.Keywords
Cite This Article
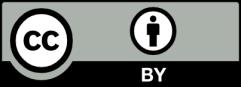