Open Access
ARTICLE
Cayley Picture Fuzzy Graphs and Interconnected Networks
1 Department of Mathematics, University of Education Lahore, Attock campus, Pakistan
2 ETS-Maths and NS Engineering Division, HCT, University City, Sharjah, United Arab Emirates
* Corresponding Author: Waheed Ahmad Khan. Email:
Intelligent Automation & Soft Computing 2023, 35(3), 3317-3330. https://doi.org/10.32604/iasc.2023.024484
Received 19 October 2021; Accepted 20 December 2021; Issue published 17 August 2022
Abstract
Theory of the Cayley graphs is directly linked with the group theory. However, if there are uncertainties on the vertices or edges or both then fuzzy graphs have an extraordinary importance. In this perspective, numbers of generalizations of fuzzy graphs have been explored in the literature. Among the others, picture fuzzy graph (PFG) has its own importance. A picture fuzzy graph (PFG) is a pair defined on a = , where = is a picture fuzzy set on and = is a picture fuzzy set over the set such that for any edge with , and In this manuscript, we introduce the notion of the Cayley picture fuzzy graphs on groups which is the generalization of the picture fuzzy graphs. Firstly, we discuss few important characteristics of the Cayley picture fuzzy graphs. We show that Cayley picture fuzzy graphs are vertex transitive and hence regular. Then, we investigate different types of Cayley graphs induced by the Cayley picture fuzzy graphs by using different types of cuts. We extensively discuss the term connectivity of the Cayley picture fuzzy graphs. Vertex connectivity and edge connectivity of the Cayley picture fuzzy graphs are also addressed. We also investigate the linkage between these two. Throughout, we provide the extensions of some characteristics of both the PFGs and Cayley fuzzy graphs in the setting of Cayley picture fuzzy graphs. Finally, we provide the model of interconnected networks based on the Cayley picture fuzzy graphs.Keywords
A classical (crisp) set involves exactly two truth values ‘True (1)’ and ‘False (0)’ which is unable to handle the raw data or the data with uncertainties. To overcome such circumstances, Zadeh [1] explored the concepts of fuzzy sets (FSs) which is proved more effective to solve the problems containing uncertainties. The fuzzy sets (FSs) is the generalized form of a classical (crisp) set in which the members of the set are allocated different degrees of membership values lying in the interval
The idea of fuzzy graphs (FGs) was proposed by Rosenfeld [11], a decade after the Zadeh’s incredible article on fuzzy sets. Fuzzy graphs are proven an effective tool to handle the problems containing unclear data. Fuzzy graph also provides us more compatible models for the solution of real world problems as compared to the classic graphs. Subsequently, Mordeson and Nair [12] introduced the concept of the complement fuzzy graph and in continuation Sunitha and Kumar explored it further in [13]. In the same perspective, Parvathi et al. [14] introduced the term intuitionistic fuzzy graphs (IFGs) at the base of intuitionistic fuzzy relation and Shannon and Atanossov [15] provided several generalized forms of IFGs. Different operations on IFGs were presented in [16,17]. Moreover, Rashmanlou et al. [18] discussed various exclusive properties of IFGs and different types of products of IFGs were discussed in [19]. The structure of IFGs has more diversity than that of FGs and hence several applications of IFGs were explored towards radio coverage networking [20], shortest path problems [21] and social networks [22]. Moreover, the term Cayley intuitionistic fuzzy graphs were discussed in [23]. Currently, the generalization of intuitionistic fuzzy graphs termed picture fuzzy graphs (PFGs) is introduced by Zua et al. [24]. They introduced some special types of picture fuzzy graphs (PFGs) like strong, complete etc. They also introduced and applied the terms weak and co-weak isomorphisms to the picture fuzzy graphs (PFGs). Few applications of PFGs towards social networking were also explored by them. Subsequently, picture fuzzy multigraphs (PFMGs) has been introduced in literature [25]. Recently, the further extensions of PFGs like constant picture fuzzy graphs [26] and balanced picture fuzzy graphs [27] are also introduced. First and third authors (with Babir Ali) have introduced the notion of bipolar picture fuzzy graphs and bipolar picture fuzzy acquaintanceship graph in [28]. Similarly, the term interval-valued picture fuzzy graphs is explored by both the authors in [29].
Numerous generalizations of the fuzzy graphs are explored in the literature to deal with the uncertainties existing in daily life complex problems. We know that the uncertainties are well explained by using PFSs and hence the Cayley picture fuzzy graphs (CPFGs) would be the excellent research area for dealing the problems having uncertainties. In this study, we suggest the definition of CPFGs at the base of picture fuzzy relation described on the group and its generators. We discuss few main characteristics of the Cayley picture fuzzy graphs. We show that the CPFGs are vertex transitive (node symmetric) and hence are regular. Consequently, we provide the linkage between Cayley picture fuzzy graphs and classical Cayley graphs as well. We discuss in detail the term connectivity of the CPFGs. Overall, we provide the generalizations of some characteristics of both the PFGs and Cayley fuzzy graphs (CFGs) in the setting of CPFGs. Finally, we provide an application of CPFGs towards interconnected networks.
Definition 1. [1] A pair
Definition 2. [1] A fuzzy subset of
Definition 3. [30] An object of the form of
Definition 4. [6] A picture fuzzy set (PFS)
Definition 5. [6] Let
Definition 6. [11] Let
Definition 7. [23] Cayley intuitionistic fuzzy graph
Definition 8. [24] A pair
Let
For further discussions on the fuzzy edge cuts and connectivity etc of FGs, we refer
3 Cayley Picture Fuzzy Graphs (CPFGs)
Throughout by a Cayley picture fuzzy graph we mean a Cayley picture fuzzy graph on a group
Definition 9. Let
Example 1. Let us take a group
Figure 1: Cayley picture fuzzy graph
Definition 10. Let
Remark 1. A Cayley picture fuzzy directed graph having equal out (resp., in) degree
Example 2. The graph given in Fig. 1 is a regular CPFG.
Theorem 1. Every CPFG is vertex transitive.
Proof. Let
From
Theorem 2. Every vertex transitive CPFG is regular.
Proof. Let
Similarly,
Thus,
4 Cayley Graphs Induced by Cayley Picture Fuzzy Graphs
In this section, first we introduce
Definition 11. Let
A level set of
where
Definition 12. Let
Theorem 3. Let
Proof. Let
Theorem 4. Given a CPFG
Proof. Since
Theorem 5. Let
Proof. Since
5 Vertex and Edge Connectivity in CPFGs
The terms vertex connectivity and edge connectivity of a fuzzy graphs have been introduced in [31]. The notion of strong edges (arcs) in fuzzy graphs was initiated in [33]. These terms were further generalized in [32]. Throughout this section, we shift these terminologies towards PFGs and CPFGs. We divide this section into three subsections. In Section 1, we discuss the notion of the vertex connectivity of a CPFGs. Section 2 is devoted for discussion on the edge connectivity of the CPFGs. In the third subsection, we explore the relationship between vertex and edge connectivity of the CPFGs.
5.1 Vertex Connectivity in CPFGs
We present the definitions of disconnection and vertex connectivity of PFGs (resp., CPFGs) which are due to the work presented in [31–33] for fuzzy graphs.
Definition 13. A disconnection of a PFG
Remark 2. In the above definition, if
Definition 14. The vertex connectivity of a CFG (resp., CPFG)
Let
Definition 15. Let
Example 3. Let
Then
Picture fuzzy vertex cuts of
5.2 Edge Connectivity in CPFGs
Definition 16. Let
Definition 17. The minimum weight of cut-sets of a PFG (resp., CPFG)
Definition 18. An edge of a PFG (resp., CPFG)
Definition 19. Let
If there are
Definition 20. A 1-CPFGEC is a CPFGEC bond (CPFGEC-bond).
Remark 3. If
Proposition 1. In a CPFG-bond of a CPFG there is at least one of the end nodes of a CPFG-bond is a CPFG-cut vertex.
Proof. Let
Example 4. Let
5.3 Relationship between Vertex Connectivity and Edge Connectivity in CPFGs
Definition 21. A CPFG
Theorem 6. In a CPF tree of a graph
such that
Proof. Let
Now every internal vertex of
However, in a common CPFG, Theorem 13 does not hold true as we observe in Example 5.
Example 5. From Examples (3 & 4), we have observed the followings.
Which implies
Due to the current progress of parallel and distributed computing, the design and analysis of diverse interconnected networks have been a significant area of research for the last few years and is still triggered by the new technologies introduced in the field of communication networking like optic fibers. In this regard, various classes of Cayley graphs are used in the modeling of interconnected networks and routings. Since the signals in such systems within the range can either be connected, disconnected or fluctuate between the positions of connected and disconnected. It is well known that in any network environment the data transferred across the network in the shape of packets. However, these packets are interrupted during their journey due to network congestion, overtaxed devices, software bugs and so on. Generally, the wireless networks face more issues with packet loss than the wired networks as the weaker signals, radio frequency interference, physical barriers and distance are the main reasons of wireless networks to drop packets. Hence the modelling of interconnected networks in the setting of fuzzy graphs is more practical. Specifically, as CPFGs is a vertex transitive so it is most suitable to describe interconnected networks. We present such model through a CPFG shown in Fig. 2.
Figure 2: Cayley picture fuzzy graph defined on
From Fig. 2 and its description, it is evident that the level of communication between and
Similarly, we may describe the levels of the vertex and edge connectivity of the interconnected networks given in Fig. 2. For this, let
Finally, the vertex and edge connectivity of path
the vertex and edge connectivity of path
and the vertex and edge connectivity of path
At the base of the above calculations, one can easily observe the strength of the network flow through these paths.
Description of Fig. 2:
Here, the values of vertices of CPFG are:
Fuzzy graphs theory plays a considerable role in modeling many real world problems containing uncertainties in different fields like computer science, decision making theory, optimization theory, data analysis, routing in networking etc. In this view, a number of generalizations of fuzzy graphs have been introduced to handle the difficult and complex real life problems. Since the picture fuzzy set is the extension of both the fuzzy sets and intuitionistic fuzzy sets. Similarly, picture fuzzy graphs are the generalization of both the fuzzy graphs and intuitionistics fuzzy graphs. In this work, we have provided the generalized form of picture fuzzy graphs named Cayley picture fuzzy graphs on the groups. We have also provided its numerous characterizations and application towards interconnected networks. We have suggested the definition of CPFGs based on the picture fuzzy relation defined on the group. Firstly, we have discussed few main characteristics of the CPFGs. Then, we have shown that the CPFGs are vertex transitive (node symmetric) and hence are regular. Subsequently, we have also provided the linkage between Cayley picture fuzzy graphs and classical Cayley graphs. In our discussion, we have also included the discussions on the Cayley graphs induced by different types of CPFGs. Afterwards, we have provided the detailed discussion on the connectivity of the CPFGs. In general, we have provided the generalizations of some characteristics of both the PFGs and Cayley fuzzy graphs (CFGs) in the setting of CPFGs. The notion of cut sets and different types of cuts of CPFGs have also addressed. In this regard, we have extensively discussed the notions of the vertex connectivity and edge connectivity of the CPFGs. Moreover, we have also inter-related these two terms. Since the fluctuations and hence uncertainties exist in interconnected networks so we have provided the model for such networks based on the CPFGs. By doing simple calculations we have investigated the strength of the network flow through different paths. On the same patterns, one could express computer networking, social networking, web graphs in the frame of CPFGs. Since the numbers of applications of Cayley graphs and PFGs have been explored in different fields of sciences, CPFGs would be an important tool to solve many others real world problems containing uncertainties. Finally, one may extend this study by initiating the concept of constant Cayley picture fuzzy graphs, Interval-valued Cayley picture fuzzy graphs etc.
Funding Statement: The authors received no specific funding for this study.
Conflicts of Interest: The authors declare that they have no conflicts of interest to report regarding the present study.
References
1. L. Zadeh, “Fuzzy sets,” Information and Control, vol. 8, no. 3, pp. 338–353, 1965. [Google Scholar]
2. L. Zadeh, “The concept of a linguistic variable and its application to approximate reasoning—I,” Information Science, vol. 8, no. 3, pp. 199–249, 1975. [Google Scholar]
3. K. T. Atanassov, “Intuitionistic fuzzy sets,” Fuzzy Sets and Systems, vol. 20, pp. 87–96, 1986. [Google Scholar]
4. T. Chaira and A. K. Ray, “A new measure using intuitionistic fuzzy set theory and its application to edge detection,” Applied Soft Computing, vol. 8, no. 2, pp. 919–927, 2008. [Google Scholar]
5. D. F. Li, “Multiattribute decision making models and methods using intuitionistic fuzzy sets,” Journal of Computer and System Sciences, vol. 70, no. 1, pp. 73–85, 2005. [Google Scholar]
6. B. C. Cuong and V. Kreinovich, “Picture fuzzy sets,” Journal of Computer Science and Cybernetics, vol. 30, no. 4, pp. 409–420, 2014. [Google Scholar]
7. P. H. Phong, D. T. Hieu, R. Ngan and P. T. Them, “Some compositions of picture fuzzy relations,” in Proc. of the 7th National Conf. on Fundamental and Applied Information Technology Research (FAIR’7), Thai Nguyen, Vietnam, 19–20 June 2014, pp. 19–20, 2014. [Google Scholar]
8. B. C. Cuong and V. H. Pham, “Some fuzzy logic operators for picture fuzzy sets,” in Proc. of the 2015 Seventh Int. Conf. on Knowledge and Systems Engineering (KSE), Ho Chi Minh City, Vietnam, 8–10 2015, pp. 132–137, 2015. [Google Scholar]
9. G. Wei, “Some cosine similarity measures for picture fuzzy sets and their applications to strategic decision making,” Informatica, vol. 28, no. 3, pp. 547–564, 2017. [Google Scholar]
10. A. M. Khalil, S. G. Li, H. Garg, H. Li and S. Ma, “New operations on interval-valued picture fuzzy set, interval-valued picture fuzzy soft set and their applications,” IEEE Access, vol. 7, pp. 51236–51253, 2019. [Google Scholar]
11. A. Rosenfeld, “Fuzzy graphs,” in Fuzzy Sets and Their Applications to Cognitive and Decision Processes, L. A. Zadeh, K. S. Fu, K. Tanaka, M. Shimura (eds.New York: Academic Press, pp. 77–95, 1975. [Google Scholar]
12. J. N. Mordeson and P. S. Nair, “Fuzzy graphs and fuzzy hypergraphs,” in Studies in Fuzziness and Soft Computing. Springer-Verlag Berlin GmbH, 2000. [Google Scholar]
13. M. Sunitha and A. Vijayakumar, “Complement of a fuzzy graph,” Indian Journal of Pure and Applied Mathematics, vol. 33, no. 9, pp. 1451–1464, 2002. [Google Scholar]
14. R. Parvathi and M. Karunambigai, “Intuitionistic fuzzy graphs,” in Journal of Computational Intelligence: Theory and Applications. Berlin, Heidelberg: Springer, pp. 139–150, 2006. [Google Scholar]
15. A. Shannon and K. T. Atanassov, “On a generalization of intuitionistic fuzzy graphs,” Notes on Intuitionistic Fuzzy Sets, vol. 12, no. 1, pp. 24–29, 2006. [Google Scholar]
16. S. Thilagavathi, R. Parvathi and M. Karunambigai, “Operations on intuitionistic fuzzy graphs II,” In: International conference in International Journal of Computer Applications, vol. 51, 2009. [Google Scholar]
17. M. Akram and R. Akmal, “Operations on intuitionistic fuzzy graph structures,” Fuzzy Information and Engineering, vol. 8, no. 4, pp. 389–410, 2016. [Google Scholar]
18. H. Rashmanlou, S. Samanta, M. Pal and R. A. Borzooei, “Intuitionistic fuzzy graphs with categorical properties,” Fuzzy Information and Engineering, vol. 7, no. 3, pp. 317–334, 2015. [Google Scholar]
19. S. Sahoo and M. Pal, “Different types of products on intuitionistic fuzzy graphs,” Pacific Science Review A: Natural Science and Engineering, vol. 17, no. 3, pp. 87–96, 2015. [Google Scholar]
20. M. Akram and W. A. Dudek, “Intuitionistic fuzzy hypergraphs with applications,” Information Sciences, vol. 218, pp. 182–193, 2013. [Google Scholar]
21. M. Karunambigai, P. Rangasamy, K. T. Atanassov and N. Palaniappan, “An intuitionistic fuzzy graph method for finding the shortest paths in networks,” in Theoretical Advances and Applications of Fuzzy Logic and Soft Computing. Berlin, Germany: Springer, pp. 3–10, 2007. [Google Scholar]
22. D. Yu and S. Shi, “Researching the development of Atanassov intuitionistic fuzzy set: Using a citation network analysis,” Applied Soft Computing, vol. 32, no. 1, pp. 189–198, 2015. [Google Scholar]
23. M. Akram, M. Karunambigai and O. Kalaivani, “Cayley intuitionistic fuzzy graphs,” Journal of Applied Mathematics and Informatics, vol. 32, no. 5–6, pp. 827–842, 2014. [Google Scholar]
24. C. Zuo, A. Pal and A. Dey, “New concepts of picture fuzzy graphs with application,” Mathematics, vol. 7, no. 5, pp. 470, 2019. [Google Scholar]
25. S. Das and G. Ghorai, “Analysis of road map design based on multigraph with picture fuzzy information,” International Journal of Applied and Computational Mathematics, vol. 6, no. 3, pp. 1–17, 2020. [Google Scholar]
26. R. Anjum, A. Gumaei and A. Ghaffar, “Certain notions of picture fuzzy information with applications,” Journal of Mathematics, vol. 2021, no. 3, pp. 1–8, 2021. [Google Scholar]
27. S. Amanathulla, B. Bera and M. Pal, “Balanced picture fuzzy graph with application,” Artificial Intelligence Review, vol. 54, no. 7, pp. 5255–5281, 2021. [Google Scholar]
28. W. A. Khan, B. Ali and A. Taouti, “Bipolar picture fuzzy graphs with application,” Symmetry, vol. 13, no. 8, pp. 1427, 2021. [Google Scholar]
29. W. A. Khan, K. Faiz and A. Taouti, “Interval-valued picture fuzzy graphs,” in Demonstratio Mathematica, 2021. [Google Scholar]
30. K. T. Atanassov, “Intuitionistic fuzzy sets,” Fuzzy Sets and Systems, vol. 20, no. 1, pp. 87–96, 1986. [Google Scholar]
31. R. T. Yeh and S. Bang, “Fuzzy relations, fuzzy graphs, and their applications to clustering analysis,” in Fuzzy Sets and Their Applications to Cognitive and Decision Process, L. A. Zadeh, K. S. Fu, M. Shimura (eds.New York, NY, USA: Academic Press, pp. 338–353, 1975. [Google Scholar]
32. S. Mathew and M. Sunitha, “Node connectivity and arc connectivity of a fuzzy graph,” Information Sciences, vol. 180, no. 4, pp. 519–531, 2010. [Google Scholar]
33. K. R. Bhutani and A. Rosenfeld, “Strong arcs in fuzzy graphs,” Information Sciences, vol. 152, pp. 319–322, 2003. [Google Scholar]
Cite This Article
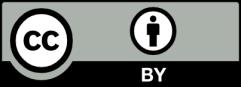