Open Access
ARTICLE
Automatic Detection of Outliers in Multi-Channel EMG Signals Using MFCC and SVM
1 Electrical Engineering Department, College of Engineering, Najran University Saudi Arabia, Najran, 61441, Saudi Arabia
2 Department of Software Engineering, University of Malakand, Dir Lower, Pakistan
3 Department of Electrical Engineering, University of Engineering and Technology, Mardan, 23200, Pakistan
4 Faculty of Maritime Studies, King Abdulaziz University, Jeddah, 21589, Saudi Arabia
5 Department of Computer Science, University of Engineering and Technology, Mardan, 23200, Pakistan
* Corresponding Author: Fazal Muhammad. Email:
Intelligent Automation & Soft Computing 2023, 36(1), 169-181. https://doi.org/10.32604/iasc.2023.032337
Received 14 May 2022; Accepted 15 June 2022; Issue published 29 September 2022
Abstract
The automatic detection of noisy channels in surface Electromyogram (sEMG) signals, at the time of recording, is very critical in making a noise-free EMG dataset. If an EMG signal contaminated by high-level noise is recorded, then it will be useless and can’t be used for any healthcare application. In this research work, a new machine learning-based paradigm is proposed to automate the detection of low-level and high-level noises occurring in different channels of high density and multi-channel sEMG signals. A modified version of mel frequency cepstral coefficients (mMFCC) is proposed for the extraction of features from sEMG channels along with other statistical parameters i-e complexity coefficient, hurst exponent, and root mean square. Several state-of-the-art classifiers such as Support Vector Machine (SVM), Ensemble Bagged Trees, Ensemble Subspace Discriminant, and Logistic Regression are used to automatically identify an EMG channel either bad or good based on these extracted features. Comparison-based analyses of these classifiers have also been considered based on total classification accuracy, prediction speed (observations/sec), and processing time. The proposed method is tested on 320 simulated EMG channels as well as 640 experimental EMG channels. SVM is used as our main classifier for the detection of noisy channels which gives a total classification accuracy of 99.4% for simulated EMG channels whereas accuracy of 98.9% is achieved for experimental EMG channels.Keywords
Cite This Article
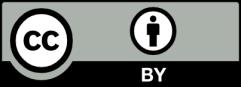