Open Access
ARTICLE
Deep Pyramidal Residual Network for Indoor-Outdoor Activity Recognition Based on Wearable Sensor
1 Department of Computer Engineering, School of Information and Communication Technology University of Phayao, Phayao, 56000, Thailand
2 Image Information and Intelligence Laboratory, Department of Computer Engineering, Faculty of Engineering, Mahidol University, Nakhon Pathom, 73170, Thailand
3 Department of Mathematics, Faculty of Applied Science, King Mongkut’s University of Technology North Bangkok, Bangkok, 10800, Thailand
4 Intelligent and Nonlinear Dynamic Innovations Research Center, Science and Technology Research Institute King Mongkut’s University of Technology North Bangkok, Bangkok, 10800, Thailand
* Corresponding Author: Anuchit Jitpattanakul. Email:
Intelligent Automation & Soft Computing 2023, 37(3), 2669-2686. https://doi.org/10.32604/iasc.2023.038549
Received 17 December 2022; Accepted 12 May 2023; Issue published 11 September 2023
Abstract
Recognition of human activity is one of the most exciting aspects of time-series classification, with substantial practical and theoretical implications. Recent evidence indicates that activity recognition from wearable sensors is an effective technique for tracking elderly adults and children in indoor and outdoor environments. Consequently, researchers have demonstrated considerable passion for developing cutting-edge deep learning systems capable of exploiting unprocessed sensor data from wearable devices and generating practical decision assistance in many contexts. This study provides a deep learning-based approach for recognizing indoor and outdoor movement utilizing an enhanced deep pyramidal residual model called SenPyramidNet and motion information from wearable sensors (accelerometer and gyroscope). The suggested technique develops a residual unit based on a deep pyramidal residual network and introduces the concept of a pyramidal residual unit to increase detection capability. The proposed deep learning-based model was assessed using the publicly available 19Nonsens dataset, which gathered motion signals from various indoor and outdoor activities, including practicing various body parts. The experimental findings demonstrate that the proposed approach can efficiently reuse characteristics and has achieved an identification accuracy of 96.37% for indoor and 97.25% for outdoor activity. Moreover, comparison experiments demonstrate that the SenPyramidNet surpasses other cutting-edge deep learning models in terms of accuracy and F1-score. Furthermore, this study explores the influence of several wearable sensors on indoor and outdoor action recognition ability.Keywords
Cite This Article
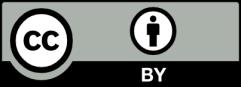