Open Access
ARTICLE
Aspect-Based Sentiment Classification Using Deep Learning and Hybrid of Word Embedding and Contextual Position
1 Department of Computer Science, COMSATS University Islamabad, Wah Campus, Wah, 47040, Pakistan
2 Department of Computer Science, Namal University, Mianwali, 42201, Pakistan
3 Department of Management Information Systems (MIS), College of Business Administration, King Faisal University (KFU), Al-Ahsa, 31982, Saudi Arabia
4 Department of Software Engineering, Al Ain University, Al Ain, 64141, United Arab Emirates
* Corresponding Authors: Hikmat Ullah Khan. Email: ; Fawaz Khaled Alarfaj. Email:
Intelligent Automation & Soft Computing 2023, 37(3), 3101-3124. https://doi.org/10.32604/iasc.2023.040614
Received 25 March 2023; Accepted 06 June 2023; Issue published 11 September 2023
Abstract
Aspect-based sentiment analysis aims to detect and classify the sentiment polarities as negative, positive, or neutral while associating them with their identified aspects from the corresponding context. In this regard, prior methodologies widely utilize either word embedding or tree-based representations. Meanwhile, the separate use of those deep features such as word embedding and tree-based dependencies has become a significant cause of information loss. Generally, word embedding preserves the syntactic and semantic relations between a couple of terms lying in a sentence. Besides, the tree-based structure conserves the grammatical and logical dependencies of context. In addition, the sentence-oriented word position describes a critical factor that influences the contextual information of a targeted sentence. Therefore, knowledge of the position-oriented information of words in a sentence has been considered significant. In this study, we propose to use word embedding, tree-based representation, and contextual position information in combination to evaluate whether their combination will improve the result’s effectiveness or not. In the meantime, their joint utilization enhances the accurate identification and extraction of targeted aspect terms, which also influences their classification process. In this research paper, we propose a method named Attention Based Multi-Channel Convolutional Neural Network (Att-MC-CNN) that jointly utilizes these three deep features such as word embedding with tree-based structure and contextual position information. These three parameters deliver to Multi-Channel Convolutional Neural Network (MC-CNN) that identifies and extracts the potential terms and classifies their polarities. In addition, these terms have been further filtered with the attention mechanism, which determines the most significant words. The empirical analysis proves the proposed approach’s effectiveness compared to existing techniques when evaluated on standard datasets. The experimental results represent our approach outperforms in the F1 measure with an overall achievement of 94% in identifying aspects and 92% in the task of sentiment classification.Keywords
Cite This Article
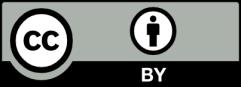