Open Access
PROCEEDINGS
A Four-Site Water Model for Liquid and Supercooled Water Based on Machine Learning: TIP4P-BGWT
1 International Research Center for Computational Mechanics, State Key Laboratory of Structural Analysis, Optimization and CAE Software for Industrial Equipment, Department of Engineering Mechanics, Faculty of Vehicle Engineering and Mechanics, Dalian University of Technology, Dalian, 116024, China
* Corresponding Author: Jian Wang. Email:
The International Conference on Computational & Experimental Engineering and Sciences 2023, 27(3), 1-1. https://doi.org/10.32604/icces.2023.09761
Abstract
Water is the most ubiquitous fluid in nature and widely exists in the micro/nanoconfinement of leafstalks, shale, bones, etc. The complex relation of the properties and behaviours of water to the temperature, pressure and confinement size enhances the difficulty in the accurate simulation, such as the supercooled state of pure water below the freezing point. As a powerful tool, molecular dynamics simulation is adequate for investigating the relevant properties and behaviours. However, accurately calculating the physical properties of liquid and supercooled water is quite challenging by molecular simulations owing to limited model parameters. Machine learning (ML) techniques and temperature-dependent parameters provide a path to efficiently reparametrize TIP4P model. Here, 7000 molecular dynamics (MD) samples for liquid and supercooled water from 253 K to 373 K are generated to train the back-propagation neural network with better generalization ability. This network could rapidly and accurately provide adequate data for genetic algorithm to reparametrize the molecular model without extra time-consuming molecular simulations. Based on the proposed optimized approach, a water model TIP4P-BGWT with temperature-dependent model parameters is established. It exhibits excellent predictive performance with comprehensive balance for the four crucial physical properties (density, vaporization enthalpy, self-diffusion coefficient and viscosity) of water. The corresponding mean absolute percentage error is 3.26%, which is lower than the existing water models. Furthermore, the calculated results of the temperature of maximum density, thermal expansion coefficient, isothermal compressibility, surface tension, radial distribution function and the average number of hydrogen bonds per molecule are also in good consistent with experiments. It is notable that the established water model exhibits excellent performance for the supercooled water. The present study offers an accurate numerical model of liquid and supercooled water for the molecular simulationbased researches on the nanoflow, nanodroplet and interfacial fluids, etc.Keywords
Cite This Article
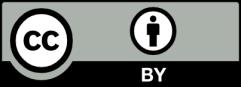