Open Access
ARTICLE
Sentiment Analysis Based on Performance of Linear Support Vector Machine and Multinomial Naïve Bayes Using Movie Reviews with Baseline Techniques
1 Department of Computer Science, City University of Science & Technology, Peshawar, 25000, Pakistan
2 Department of Computer Science and Software Technology, University of Swat, Swat, 19200, Pakistan
3 Department of Computer Software Engineering, University of Engineering & Technology Mardan, Mardan, 23200, Pakistan
4 Department of Computer Science, IQRA National University, Swat, 19200, Pakistan
* Corresponding Author: Muzammil Khan. Email:
Journal on Big Data 2023, 5, 1-18. https://doi.org/10.32604/jbd.2023.041319
Received 18 April 2023; Accepted 05 June 2023; Issue published 29 September 2023
Abstract
Movies are the better source of entertainment. Every year, a great percentage of movies are released. People comment on movies in the form of reviews after watching them. Since it is difficult to read all of the reviews for a movie, summarizing all of the reviews will help make this decision without wasting time in reading all of the reviews. Opinion mining also known as sentiment analysis is the process of extracting subjective information from textual data. Opinion mining involves identifying and extracting the opinions of individuals, which can be positive, neutral, or negative. The task of opinion mining also called sentiment analysis is performed to understand people’s emotions and attitudes in movie reviews. Movie reviews are an important source of opinion data because they provide insight into the general public’s opinions about a particular movie. The summary of all reviews can give a general idea about the movie. This study compares baseline techniques, Logistic Regression, Random Forest Classifier, Decision Tree, K-Nearest Neighbor, Gradient Boosting Classifier, and Passive Aggressive Classifier with Linear Support Vector Machines and Multinomial Naïve Bayes on the IMDB Dataset of 50K reviews and Sentiment Polarity Dataset Version 2.0. Before applying these classifiers, in pre-processing both datasets are cleaned, duplicate data is dropped and chat words are treated for better results. On the IMDB Dataset of 50K reviews, Linear Support Vector Machines achieve the highest accuracy of 89.48%, and after hyperparameter tuning, the Passive Aggressive Classifier achieves the highest accuracy of 90.27%, while Multinomial Nave Bayes achieves the highest accuracy of 70.69% and 71.04% after hyperparameter tuning on the Sentiment Polarity Dataset Version 2.0. This study highlights the importance of sentiment analysis as a tool for understanding the emotions and attitudes in movie reviews and predicts the performance of a movie based on the average sentiment of all the reviews.Keywords
Cite This Article
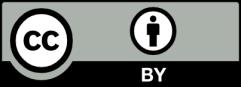