Open Access
ARTICLE
A Survey on Machine Learning Algorithms in Little-Labeled Data for Motor Imagery-Based Brain-Computer Interfaces
School of Computer and Communication Engineering, Changsha University of Science and Technology, Changsha, 410114, China.
Hunan Provincial Key Laboratory of Intelligent Processing of Big Data on Transportation, Changsha University of Science and Technology, Changsha, 410114, China.
School of Mechanical and Aerospace Engineering, Nanyang Technological University, Singapore , 639798, Singapore.
*Corresponding Author: Fei Wang. Email: .
Journal of Information Hiding and Privacy Protection 2019, 1(1), 11-21. https://doi.org/10.32604/jihpp.2019.05979
Abstract
The Brain-Computer Interfaces (BCIs) had been proposed and used in therapeutics for decades. However, the need of time-consuming calibration phase and the lack of robustness, which are caused by little-labeled data, are restricting the advance and application of BCI, especially for the BCI based on motor imagery (MI). In this paper, we reviewed the recent development in the machine learning algorithm used in the MI-based BCI, which may provide potential solutions for addressing the issue. We classified these algorithms into two categories, namely, and enhancing the representation and expanding the training set. Specifically, these methods of enhancing the representation of features collected from few EEG trials are based on extracting features of multiple bands, regularization, and so on. The methods of expanding the training dataset include approaches of transfer learning (session to session transfer, subject to subject transfer) and generating artificial EEG data. The result of these techniques showed the resolution of the challenges to some extent. As a developing research area, the study of BCI algorithms in little-labeled data is increasingly requiring the advancement of human brain physiological structure research and more transfer learning algorithms research.Keywords
Cite This Article
Y. Jia, F. Li, F. Wang and Y. Gui, "A survey on machine learning algorithms in little-labeled data for motor imagery-based brain-computer interfaces," Journal of Information Hiding and Privacy Protection, vol. 1, no.1, pp. 11–21, 2019. https://doi.org/10.32604/jihpp.2019.05979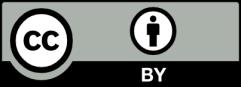