Open Access
ARTICLE
Extensive prediction of drug response in mutation-subtype-specific LUAD with machine learning approach
1 Department of Thoracic Surgery, Sichuan Provincial People’s Hospital, University of Electronic Science and Technology of China, Chengdu, China
2 School of Medicine, University of Electronic Science and Technology of China, Chengdu, China
3 Department of Assisted Reproductive Medicine, Sichuan Provincial Academy of Medical Sciences & Sichuan Provincial People’s Hospital, Chengdu, China
* Corresponding Authors: QI CAO. Email: ; YOUYU WANG. Email:
# These authors contributed equally to this work
(This article belongs to the Special Issue: Multi-Omics Approaches for Precision Medicine)
Oncology Research 2024, 32(2), 409-419. https://doi.org/10.32604/or.2023.042863
Received 14 June 2023; Accepted 25 September 2023; Issue published 28 December 2023
Abstract
Background: Lung cancer is the most prevalent cancer diagnosis and the leading cause of cancer death worldwide. Therapeutic failure in lung cancer (LUAD) is heavily influenced by drug resistance. This challenge stems from the diverse cell populations within the tumor, each having unique genetic, epigenetic, and phenotypic profiles. Such variations lead to varied therapeutic responses, thereby contributing to tumor relapse and disease progression. Methods: The Genomics of Drug Sensitivity in Cancer (GDSC) database was used in this investigation to obtain the mRNA expression dataset, genomic mutation profile, and drug sensitivity information of NSCLS. Machine Learning (ML) methods, including Random Forest (RF), Artificial Neurol Network (ANN), and Support Vector Machine (SVM), were used to predict the response status of each compound based on the mRNA and mutation characteristics determined using statistical methods. The most suitable method for each drug was proposed by comparing the prediction accuracy of different ML methods, and the selected mRNA and mutation characteristics were identified as molecular features for the drug-responsive cancer subtype. Finally, the prognostic influence of molecular features on the mutational subtype of LUAD in publicly available datasets. Results: Our analyses yielded 1,564 gene features and 45 mutational features for 46 drugs. Applying the ML approach to predict the drug response for each medication revealed an upstanding performance for SVM in predicting Afuresertib drug response (area under the curve [AUC] 0.875) using CIT, GAS2L3, STAG3L3, ATP2B4-mut, and IL15RA-mut as molecular features. Furthermore, the ANN algorithm using 9 mRNA characteristics demonstrated the highest prediction performance (AUC 0.780) in Gefitinib with CCL23-mut. Conclusion: This work extensively investigated the mRNA and mutation signatures associated with drug response in LUAD using a machine-learning approach and proposed a priority algorithm to predict drug response for different drugs.Keywords
Cite This Article
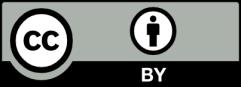