Open Access
ARTICLE
FP-STE: A Novel Node Failure Prediction Method Based on Spatio-Temporal Feature Extraction in Data Centers
1 State Key Laboratory of Networking and Switching Technology, Beijing University of Posts and Telecommunications, Beijing, China
2 Beijing Smartchip Microelectronics Technology Company Limited, Beijing, China
3 The 54th Research Institute of CETC, Shijiazhuang, China
* Corresponding Author: Yang Yang. Email:
Computer Modeling in Engineering & Sciences 2020, 123(3), 1015-1031. https://doi.org/10.32604/cmes.2020.09404
Received 10 December 2019; Accepted 17 March 2020; Issue published 28 May 2020
Abstract
The development of cloud computing and virtualization technology has brought great challenges to the reliability of data center services. Data centers typically contain a large number of compute and storage nodes which may fail and affect the quality of service. Failure prediction is an important means of ensuring service availability. Predicting node failure in cloud-based data centers is challenging because the failure symptoms reflected have complex characteristics, and the distribution imbalance between the failure sample and the normal sample is widespread, resulting in inaccurate failure prediction. Targeting these challenges, this paper proposes a novel failure prediction method FP-STE (Failure Prediction based on Spatio-temporal Feature Extraction). Firstly, an improved recurrent neural network HW-GRU (Improved GRU based on HighWay network) and a convolutional neural network CNN are used to extract the temporal features and spatial features of multivariate data respectively to increase the discrimination of different types of failure symptoms which improves the accuracy of prediction. Then the intermediate results of the two models are added as features into SCSXGBoost to predict the possibility and the precise type of node failure in the future. SCS-XGBoost is an ensemble learning model that is improved by the integrated strategy of oversampling and cost-sensitive learning. Experimental results based on real data sets confirm the effectiveness and superiority of FP-STE.Keywords
Cite This Article
Yang, Y., Dong, J., Fang, C., Xie, P., An, N. (2020). FP-STE: A Novel Node Failure Prediction Method Based on Spatio-Temporal Feature Extraction in Data Centers. CMES-Computer Modeling in Engineering & Sciences, 123(3), 1015–1031. https://doi.org/10.32604/cmes.2020.09404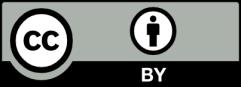