Open Access
ARTICLE
Study on the Improvement of the Application of Complete Ensemble Empirical Mode Decomposition with Adaptive Noise in Hydrology Based on RBFNN Data Extension Technology
1 School of Water Conservancy Engineering, Zhengzhou University, Zhengzhou, 450001, China
2 Yellow River Institute for Ecological Protection & Regional Coordinated Development, Zhengzhou University, Zhengzhou, 450001, China
3 North China University of Water Resources and Electric Power, Zhengzhou, 450046, China
4 School of Civil Engineering and Environmental Sciences, University of Oklahoma, Norman, OK, 73019, USA
* Corresponding Author: Bin Sun. Email:
(This article belongs to the Special Issue: Machine Learning based Methods for Mechanics)
Computer Modeling in Engineering & Sciences 2021, 126(2), 755-770. https://doi.org/10.32604/cmes.2021.012686
Received 08 July 2020; Accepted 14 October 2020; Issue published 21 January 2021
Abstract
The complex nonlinear and non-stationary features exhibited in hydrologic sequences make hydrological analysis and forecasting difficult. Currently, some hydrologists employ the complete ensemble empirical mode decomposition with adaptive noise (CEEMDAN) method, a new time-frequency analysis method based on the empirical mode decomposition (EMD) algorithm, to decompose non-stationary raw data in order to obtain relatively stationary components for further study. However, the endpoint effect in CEEMDAN is often neglected, which can lead to decomposition errors that reduce the accuracy of the research results. In this study, we processed an original runoff sequence using the radial basis function neural network (RBFNN) technique to obtain the extension sequence before utilizing CEEMDAN decomposition. Then, we compared the decomposition results of the original sequence, RBFNN extension sequence, and standard sequence to investigate the influence of the endpoint effect and RBFNN extension on the CEEMDAN method. The results indicated that the RBFNN extension technique effectively reduced the error of medium and low frequency components caused by the endpoint effect. At both ends of the components, the extension sequence more accurately reflected the true fluctuation characteristics and variation trends. These advances are of great significance to the subsequent study of hydrology. Therefore, the CEEMDAN method, combined with an appropriate extension of the original runoff series, can more precisely determine multi-time scale characteristics, and provide a credible basis for the analysis of hydrologic time series and hydrological forecasting.Keywords
Cite This Article
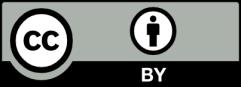