Open Access
ARTICLE
Fractional Gradient Descent RBFNN for Active Fault-Tolerant Control of Plant Protection UAVs
1 School of Mechanical Engineering, Yangzhou University, Yangzhou, 225009, China
2 College of Intelligent Manufacturing, Yangzhou Polytechnic Institute, Yangzhou, 225009, China
3 School of Automation, Nanjing University of Aeronautics and Astronautics, Nanjing, 211106, China
* Corresponding Author: Jianfeng Zhang. Email:
Computer Modeling in Engineering & Sciences 2024, 138(3), 2129-2157. https://doi.org/10.32604/cmes.2023.030535
Received 12 April 2023; Accepted 26 July 2023; Issue published 15 December 2023
Abstract
With the increasing prevalence of high-order systems in engineering applications, these systems often exhibit significant disturbances and can be challenging to model accurately. As a result, the active disturbance rejection controller (ADRC) has been widely applied in various fields. However, in controlling plant protection unmanned aerial vehicles (UAVs), which are typically large and subject to significant disturbances, load disturbances and the possibility of multiple actuator faults during pesticide spraying pose significant challenges. To address these issues, this paper proposes a novel fault-tolerant control method that combines a radial basis function neural network (RBFNN) with a second-order ADRC and leverages a fractional gradient descent (FGD) algorithm. We integrate the plant protection UAV model’s uncertain parameters, load disturbance parameters, and actuator fault parameters and utilize the RBFNN for system parameter identification. The resulting ADRC exhibits load disturbance suppression and fault tolerance capabilities, and our proposed active fault-tolerant control law has Lyapunov stability implications. Experimental results obtained using a multi-rotor fault-tolerant test platform demonstrate that the proposed method outperforms other control strategies regarding load disturbance suppression and fault-tolerant performance.Graphical Abstract
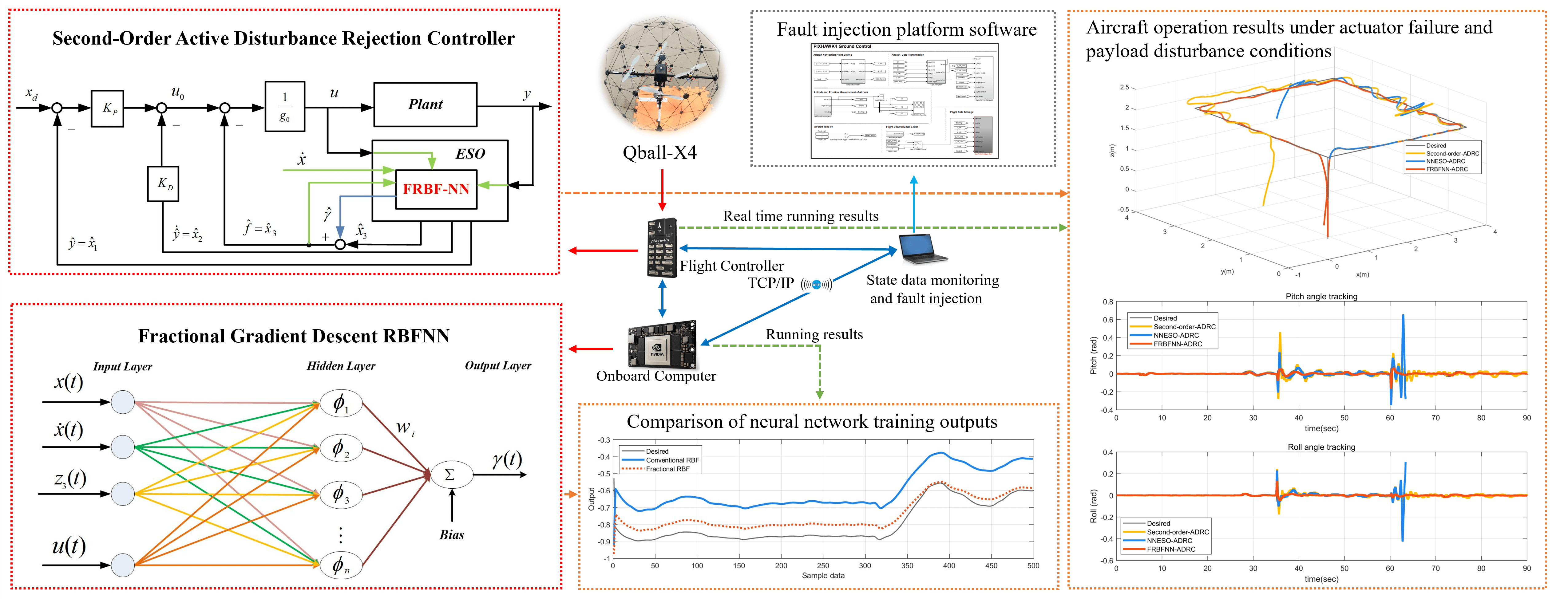
Keywords
Cite This Article
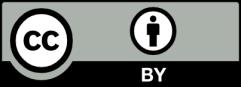