Open Access
ARTICLE
Multi-Feature Fusion-Guided Multiscale Bidirectional Attention Networks for Logistics Pallet Segmentation
1
School of Logistics and Transportation, Central South University of Forestry and Technology, Changsha, 410004, China
2
Graduate School, Northern Arizona University, Flagstaff, AZ 86011, USA
* Corresponding Author: Zhanguo Wei. Email:
(This article belongs to the Special Issue: Computer Modeling for Smart Cities Applications)
Computer Modeling in Engineering & Sciences 2022, 131(3), 1539-1555. https://doi.org/10.32604/cmes.2022.019785
Received 15 October 2021; Accepted 01 December 2021; Issue published 19 April 2022
Abstract
In the smart logistics industry, unmanned forklifts that intelligently identify logistics pallets can improve work efficiency in warehousing and transportation and are better than traditional manual forklifts driven by humans. Therefore, they play a critical role in smart warehousing, and semantics segmentation is an effective method to realize the intelligent identification of logistics pallets. However, most current recognition algorithms are ineffective due to the diverse types of pallets, their complex shapes, frequent blockades in production environments, and changing lighting conditions. This paper proposes a novel multi-feature fusion-guided multiscale bidirectional attention (MFMBA) neural network for logistics pallet segmentation. To better predict the foreground category (the pallet) and the background category (the cargo) of a pallet image, our approach extracts three types of features (grayscale, texture, and Hue, Saturation, Value features) and fuses them. The multiscale architecture deals with the problem that the size and shape of the pallet may appear different in the image in the actual, complex environment, which usually makes feature extraction difficult. Our study proposes a multiscale architecture that can extract additional semantic features. Also, since a traditional attention mechanism only assigns attention rights from a single direction, we designed a bidirectional attention mechanism that assigns cross-attention weights to each feature from two directions, horizontally and vertically, significantly improving segmentation. Finally, comparative experimental results show that the precision of the proposed algorithm is 0.53%–8.77% better than that of other methods we compared.Keywords
Cite This Article
Citations
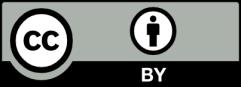