Open Access
ARTICLE
gscaLCA in R: Fitting Fuzzy Clustering Analysis Incorporated with Generalized Structured Component Analysis
1
Department of Education, College of Educational Sciences, Yonsei University, Seoul, 03722, South Korea
2
Psychometrics Department, American Board of Internal Medicine, Philadelphia, 19016, USA
3
Department of Educational Research Methodology, School of Education, University of North Carolina at Greensboro,
Greensboro, 27412, USA
4
Department of Psychology, McGill University, Montreal, H3A 0G4, Canada
* Corresponding Author: Ji Hoon Ryoo. Email:
(This article belongs to the Special Issue: Algebra, Number Theory, Combinatorics and Their Applications: Mathematical Theory and Computational Modelling)
Computer Modeling in Engineering & Sciences 2022, 132(3), 801-822. https://doi.org/10.32604/cmes.2022.019708
Received 10 October 2021; Accepted 25 January 2022; Issue published 27 June 2022
Abstract
Clustering analysis identifying unknown heterogenous subgroups of a population (or a sample) has become increasingly popular along with the popularity of machine learning techniques. Although there are many software packages running clustering analysis, there is a lack of packages conducting clustering analysis within a structural equation modeling framework. The package, gscaLCA which is implemented in the R statistical computing environment, was developed for conducting clustering analysis and has been extended to a latent variable modeling. More specifically, by applying both fuzzy clustering (FC) algorithm and generalized structured component analysis (GSCA), the package gscaLCA computes membership prevalence and item response probabilities as posterior probabilities, which is applicable in mixture modeling such as latent class analysis in statistics. As a hybrid model between data clustering in classifications and model-based mixture modeling approach, fuzzy clusterwise GSCA, denoted as gscaLCA, encompasses many advantages from both methods: (1) soft partitioning from FC and (2) efficiency in estimating model parameters with bootstrap method via resolution of global optimization problem from GSCA. The main function, gscaLCA, works for both binary and ordered categorical variables. In addition, gscaLCA can be used for latent class regression as well. Visualization of profiles of latent classes based on the posterior probabilities is also available in the package gscaLCA. This paper contributes to providing a methodological tool, gscaLCA that applied researchers such as social scientists and medical researchers can apply clustering analysis in their research.Keywords
Cite This Article
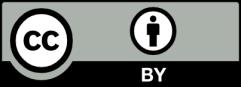