Open Access
ARTICLE
Hybridization of Fuzzy and Hard Semi-Supervised Clustering Algorithms Tuned with Ant Lion Optimizer Applied to Higgs Boson Search
1 Faculty of Sciences and Technology, Department of Applied Mathematics and Computer Sciences, Cadi Ayyad University, Marrakech, 40000, Morocco
2 Robotics and Internet-of-Things Lab (RIOTU), Prince Sultan University, Riyadh, 12435, Saudi Arabia
3 Faculty of Computers and Artificial Intelligence, Benha University, Benha, 13511, Egypt
* Corresponding Author: Soukaina Mjahed. Email:
Computer Modeling in Engineering & Sciences 2020, 125(2), 459-494. https://doi.org/10.32604/cmes.2020.010791
Received 30 March 2020; Accepted 31 July 2020; Issue published 12 October 2020
Abstract
This paper focuses on the unsupervised detection of the Higgs boson particle using the most informative features and variables which characterize the “Higgs machine learning challenge 2014” data set. This unsupervised detection goes in this paper analysis through 4 steps: (1) selection of the most informative features from the considered data; (2) definition of the number of clusters based on the elbow criterion. The experimental results showed that the optimal number of clusters that group the considered data in an unsupervised manner corresponds to 2 clusters; (3) proposition of a new approach for hybridization of both hard and fuzzy clustering tuned with Ant Lion Optimization (ALO); (4) comparison with some existing metaheuristic optimizations such as Genetic Algorithm (GA) and Particle Swarm Optimization (PSO). By employing a multi-angle analysis based on the cluster validation indices, the confusion matrix, the efficiencies and purities rates, the average cost variation, the computational time and the Sammon mapping visualization, the results highlight the effectiveness of the improved Gustafson–Kessel algorithm optimized with ALO (ALOGK) to validate the proposed approach. Even if the paper gives a complete clustering analysis, its novel contribution concerns only the Steps (1) and (3) considered above. The first contribution lies in the method used for Step (1) to select the most informative features and variables. We used the t-Statistic technique to rank them. Afterwards, a feature mapping is applied using Self-Organizing Map (SOM) to identify the level of correlation between them. Then, Particle Swarm Optimization (PSO), a metaheuristic optimization technique, is used to reduce the data set dimension. The second contribution of this work concern the third step, where each one of the clustering algorithms as K-means (KM), Global K-means (GlobalKM), Partitioning Around Medoids (PAM), Fuzzy C-means (FCM), Gustafson–Kessel (GK) and Gath–Geva (GG) is optimized and tuned with ALO.Keywords
Cite This Article
Citations
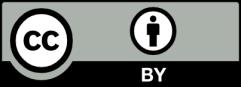