Open Access
ARTICLE
Interpreting Randomly Wired Graph Models for Chinese NER
1
The School of Electronic and Information Engineering, Suzhou University of Science and Technology, Suzhou, China
2
The School of Computer Science, Texas Tech University, Texas, USA
* Corresponding Author: Xuefeng Xi. Email:
(This article belongs to the Special Issue: Advanced Intelligent Decision and Intelligent Control with Applications in Smart City)
Computer Modeling in Engineering & Sciences 2023, 134(1), 747-761. https://doi.org/10.32604/cmes.2022.020771
Received 10 December 2021; Accepted 25 February 2022; Issue published 24 August 2022
Abstract
Interpreting deep neural networks is of great importance to understand and verify deep models for natural language processing (NLP) tasks. However, most existing approaches only focus on improving the performance of models but ignore their interpretability. In this work, we propose a Randomly Wired Graph Neural Network (RWGNN) by using graph to model the structure of Neural Network, which could solve two major problems (word-boundary ambiguity and polysemy) of Chinese NER. Besides, we develop a pipeline to explain the RWGNN by using Saliency Map and Adversarial Attacks. Experimental results demonstrate that our approach can identify meaningful and reasonable interpretations for hidden states of RWGNN.Keywords
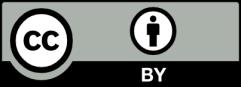