Open Access
ARTICLE
An Effective Machine-Learning Based Feature Extraction/Recognition Model for Fetal Heart Defect Detection from 2D Ultrasonic Imageries
1 College of Engineering, Huaqiao University, Quanzhou, 362021, China
2 Department of Ultrasound, The Second Affiliated Hospital of Fujian Medical University, Quanzhou, 362021, China
3 Collaborative Innovation Center for Maternal and Infant Health Service Application Technology, Quanzhou Medical College, Quanzhou, 362021, China
* Corresponding Author: Guorong Lv. Email:
(This article belongs to the Special Issue: Enabled and Human-centric Computational Intelligence Solutions for Visual Understanding and Application)
Computer Modeling in Engineering & Sciences 2023, 134(2), 1069-1089. https://doi.org/10.32604/cmes.2022.020870
Received 20 December 2021; Accepted 14 March 2022; Issue published 31 August 2022
Abstract
Congenital heart defect, accounting for about 30% of congenital defects, is the most common one. Data shows that congenital heart defects have seriously affected the birth rate of healthy newborns. In Fetal and Neonatal Cardiology, medical imaging technology (2D ultrasonic, MRI) has been proved to be helpful to detect congenital defects of the fetal heart and assists sonographers in prenatal diagnosis. It is a highly complex task to recognize 2D fetal heart ultrasonic standard plane (FHUSP) manually. Compared with manual identification, automatic identification through artificial intelligence can save a lot of time, ensure the efficiency of diagnosis, and improve the accuracy of diagnosis. In this study, a feature extraction method based on texture features (Local Binary Pattern LBP and Histogram of Oriented Gradient HOG) and combined with Bag of Words (BOW) model is carried out, and then feature fusion is performed. Finally, it adopts Support Vector Machine (SVM) to realize automatic recognition and classification of FHUSP. The data includes 788 standard plane data sets and 448 normal and abnormal plane data sets. Compared with some other methods and the single method model, the classification accuracy of our model has been obviously improved, with the highest accuracy reaching 87.35%. Similarly, we also verify the performance of the model in normal and abnormal planes, and the average accuracy in classifying abnormal and normal planes is 84.92%. The experimental results show that this method can effectively classify and predict different FHUSP and can provide certain assistance for sonographers to diagnose fetal congenital heart disease.Keywords
Cite This Article
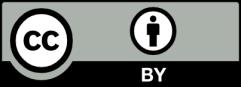