Open Access
ARTICLE
Differentiate Xp11.2 Translocation Renal Cell Carcinoma from Computed Tomography Images and Clinical Data with ResNet-18 CNN and XGBoost
1 Department of Urology, Nanjing Drum Tower Hospital, The Affiliated Hospital of Nanjing University Medical School, Nanjing, 210008, China
2 School of Data Science, Perdana University, Serdang, 43400, Malaysia
3 State Key Laboratory for Novel Software Technology, Nanjing University, Nanjing, 210008, China
* Corresponding Authors: Tong Lu. Email: ; Weidong Gan. Email:
# These authors contributed equally to this work
(This article belongs to the Special Issue: Computer Modeling of Artificial Intelligence and Medical Imaging)
Computer Modeling in Engineering & Sciences 2023, 136(1), 347-362. https://doi.org/10.32604/cmes.2023.024909
Received 13 June 2022; Accepted 23 August 2022; Issue published 05 January 2023
Abstract
This study aims to apply ResNet-18 convolutional neural network (CNN) and XGBoost to preoperative computed tomography (CT) images and clinical data for distinguishing Xp11.2 translocation renal cell carcinoma (Xp11.2 tRCC) from common subtypes of renal cell carcinoma (RCC) in order to provide patients with individualized treatment plans. Data from 45 patients with Xp11.2 tRCC from January 2007 to December 2021 are collected. Clear cell RCC (ccRCC), papillary RCC (pRCC), or chromophobe RCC (chRCC) can be detected from each patient. CT images are acquired in the following three phases: unenhanced, corticomedullary, and nephrographic. A unified framework is proposed for the classification of renal masses. In this framework, ResNet-18 CNN is employed to classify renal cancers with CT images, while XGBoost is adopted with clinical data. Experiments demonstrate that, if applying ResNet-18 CNN or XGBoost singly, the latter outperforms the former, while the framework integrating both technologies performs similarly or better than urologists. Especially, the possibility of misclassifying Xp11.2 tRCC, pRCC, and chRCC as ccRCC by the proposed framework is much lower than urologists.Keywords
Cite This Article
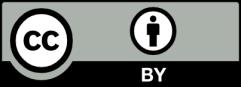