Open Access
ARTICLE
Pedestrian and Vehicle Detection Based on Pruning YOLOv4 with Cloud-Edge Collaboration
1
School of Computer Science and Engineering, Huizhou University, Huizhou, 516007, China
2
School of Computer Science and Engineering, South China University of Technology, Guangzhou, 510006, China
3
School of Computer Science and Technology, South China Normal University, Guangzhou, 510631, China
4
Department of New Network Technologies, Peng Cheng Laboratory, Shenzhen, 518066, China
5
Shenzhen Institute of Advanced Technology, Chinese Academy of Sciences, Shenzhen, 518055, China
* Corresponding Authors: Weiwei Lin. Email: ; Bo Liu. Email:
(This article belongs to the Special Issue: Advances in Edge Intelligence for Internet of Things)
Computer Modeling in Engineering & Sciences 2023, 137(2), 2025-2047. https://doi.org/10.32604/cmes.2023.026910
Received 31 October 2022; Accepted 03 February 2023; Issue published 26 June 2023
Abstract
Nowadays, the rapid development of edge computing has driven an increasing number of deep learning applications deployed at the edge of the network, such as pedestrian and vehicle detection, to provide efficient intelligent services to mobile users. However, as the accuracy requirements continue to increase, the components of deep learning models for pedestrian and vehicle detection, such as YOLOv4, become more sophisticated and the computing resources required for model training are increasing dramatically, which in turn leads to significant challenges in achieving effective deployment on resource-constrained edge devices while ensuring the high accuracy performance. For addressing this challenge, a cloud-edge collaboration-based pedestrian and vehicle detection framework is proposed in this paper, which enables sufficient training of models by utilizing the abundant computing resources in the cloud, and then deploying the well-trained models on edge devices, thus reducing the computing resource requirements for model training on edge devices. Furthermore, to reduce the size of the model deployed on edge devices, an automatic pruning method combines the convolution layer and BN layer is proposed to compress the pedestrian and vehicle detection model size. Experimental results show that the framework proposed in this paper is able to deploy the pruned model on a real edge device, Jetson TX2, with 6.72 times higher FPS. Meanwhile, the channel pruning reduces the volume and the number of parameters to 96.77% for the model, and the computing amount is reduced to 81.37%.Keywords
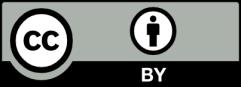