Open Access
EDITORIAL
Introduction to the Special Issue on Computer Modeling for Smart Cities Applications
1 Department of Electrical and Computer Engineering, Cleveland State University, Cleveland, OH 44115, USA
2 School of Computer Science, Xiamen University, Xiamen, 36100, China
3 School of Artificial Intelligent and Computer, Jiangnan University, Wuxi, 224122, China
* Corresponding Author: Wenbing Zhao. Email:
(This article belongs to the Special Issue: Computer Modeling for Smart Cities Applications)
Computer Modeling in Engineering & Sciences 2024, 138(2), 1015-1017. https://doi.org/10.32604/cmes.2023.031566
Received 12 July 2023; Accepted 19 July 2023; Issue published 17 November 2023
Abstract
This article has no abstract.A significant fraction of the world’s population is living in cities. With the rapid development of information and computing technologies (ICT), cities may be made smarter by embedding ICT into their infrastructure. By smarter, we mean that the city operation will be more efficient, cost-effective, energy-saving, be more connected, more secure, and more environmentally friendly. As such, a smart city is typically defined as a city that has a strong integration with ICT in all its components, including its physical components, social components, and business components [1,2].
In [1], a set of smart city functions was defined, including the smart economy (higher competition powered by an innovative spirit, entrepreneurship, and higher productivity), smart people (high level of social and human capital powered by a higher level of competence, affinity to life-long learning, greater flexibility and creativity, and better participation in public life), smart governance (higher level of participation powered by an increased level of transparency and better public services), smart mobility (more convenient and more efficient transportation powered by ICT), smart environment (better management of natural resources), and smart living (better quality of life).
In [2], the composition of a smart city was defined from a different perspective from [1], which includes smart community, smart hospitality, smart transportation, smart healthcare, smart grid, smart warehouse, and smart factory. Furthermore, Silva et al. [2] proposed an ICT architecture for smart cities, which includes the sensing layer, transmission layer, data management layer, and application layer.
This special issue attracted several dozens of submissions and accepted nine papers. These papers cover many interesting areas in the context of smart cities, ranging from fabric manufacturing to cultural relics protection, smart governments, smart warehousing, water quality analysis, water distribution network leakage management, indoor positioning, and smart environmental monitoring. That said, the research reported in this special issue is only the beginning of our goal. Much more research is needed to answer the set of research questions we outlined in the call for papers for this special issue: (1) what are the common set of requirements for smart cities? (2) how could the enabling technologies work together seamlessly toward future smart cities? (3) can we develop a comprehensive model for smart cities that captures all important perspectives?
In [3], the authors investigated how to automatically classify the images of yarn-dyed plaid fabrics, which could be useful in sample order production, fabric design, and fabric stock management. The proposed deep learning method with the strip pooling model improved between 2–4 percent over the state of art methods.
In [4], the authors studied how to identify material surface paint murals, which is an important step in the protection and restoration of murals. This paper proposed a novel method for the classification of matching pigments based on feature differences. The mural pigment is observed using a reflection spectrum for in situ analysis. The research belongs to the bigger scope of cultural relics protection.
In [5], the authors proposed a new accepted model for mobile government services. This model combines the information system success factor model and the Hofstede cultural dimensions theory. The new model captures five factors that might impact the acceptance of mobile government services, including information quality, service quality, power distance, uncertainty avoidance, and the balance between indulgence and restraint. The model is validated using questionnaires with 203 respondents in Jordan.
In [6], the authors investigated how to perform automated logistics pallet segmentation, which is a critical step in developing smart warehousing. This paper proposed a novel neural network called multi-feature fusion-guided multiscale bidirectional attention for logistics pallet segmentation. More specifically, three features are extracted and fused together to better distinguish foreground and background objects. Experiments showed that the proposed method can improve the prediction precision by up to 8.77%.
In [7], the authors proposed a new method for dividing the water distribution network into district metered areas. This division is critical in performing leakage management in water distribution networks. The proposed formation of district metered areas consists of three stages: (1) clustering; (2) boundary optimization; and (3) performance evaluation. The proposed method was validated with a real-world water network.
In [8], the authors studied how to advance the state of the arts in water quality analysis. Water quality analysis is not only essential to the understanding of the ecological state of aquatic life but also impacts the residents around the water area. More specifically, this paper explored the use of alternative water quality input variables as new discriminant features.
In [9], the authors presented a comprehensive review of the use of automated guided vehicles (AGVs) in warehouses. This paper compared the pros and cons of centralized and decentralized AGV systems and reviewed the optimization methods on the warehouse layout and operation, as well as AGV scheduling algorithms. The authors proposed a novel method for multi-AGV routing planning.
In [10], the authors proposed a new method of indoor positioning. This method is called a strong tracking particle filter based on the chi-square test (SPFC). The proposed SPFC method fuses indoor WiFi signals and motion data collected from a smartphone (via the built-in IMU). The validation of the proposed method was done via a simulation, which demonstrated a 12%–15% reduction in positioning error.
In [11], the authors investigated remote sensing image classification with a novel algorithm called transductive transfer dictionary learning (TTDL). The research work could be significant in smart environmental monitoring. The proposed TTDL algorithm was validated with several public remote-sensing image datasets and demonstrated good classification performance.
Funding Statement: This editorial work was supported in part by the US NSF Grant 2215388.
Conflicts of Interest: The authors declare that they have no conflicts of interest to report regarding the present study.
References
1. Batty, M., Axhausen, K. W., Giannotti, F., Pozdnoukhov, A., Bazzani, A. et al. (2012). Smart cities of the future. The European Physical Journal Special Topics, 214, 481–518. [Google Scholar]
2. Silva, B. N., Khan, M., Han, K. (2018). Towards sustainable smart cities: A review of trends, architectures, components, and open challenges in smart cities. Sustainable Cities and Society, 38, 697–713. [Google Scholar]
3. Zhang, X., Gao, W., Pan, R. (2022). Deep neural network with strip pooling for image classification of yarn-dyed plaid fabrics. Computer Modeling in Engineering & Sciences, 130(3), 1533–1546. https://doi.org/10.32604/cmes.2022.018763 [Google Scholar] [PubMed] [CrossRef]
4. Da, M., Wang, H., Wang, K., Wang, Z. (2022). Spectral matching classification method of multi-state similar pigments based on feature differences. Computer Modeling in Engineering & Sciences, 131(1), 513–527. https://doi.org/10.32604/cmes.2022.019040 [Google Scholar] [PubMed] [CrossRef]
5. Althunibat, A., Abdallah, M., Almaiah, M. A., Alabwaini, N., Alrawashdeh, T. A. (2022). An acceptance model of using mobile-government services (AMGS). Computer Modeling in Engineering & Sciences, 131(2), 865–880. https://doi.org/10.32604/cmes.2022.019075 [Google Scholar] [PubMed] [CrossRef]
6. Cai, W., Song, Y., Duan, H., Xia, Z., Wei, Z. (2022). Multi-feature fusion-guided multiscale bidirectional attention networks for logistics pallet segmentation. Computer Modeling in Engineering & Sciences, 131(3), 1539–1555. https://doi.org/10.32604/cmes.2022.019785 [Google Scholar] [PubMed] [CrossRef]
7. Sharma, A. N., Dongre, S. R., Gupta, R., Pandey, P., Bokde, N. D. (2022). Partitioning of water distribution network into district metered areas using existing valves. Computer Modeling in Engineering & Sciences, 131(3), 1515–1537. https://doi.org/10.32604/cmes.2022.018867 [Google Scholar] [PubMed] [CrossRef]
8. Wong, W. Y., Khallel, A., Hasikin, K., Salwa, A., Razak, S. A. et al. (2022). Water quality index using modified random forest technique: Assessing novel input features. Computer Modeling in Engineering & Sciences, 132(3), 1011–1038. https://doi.org/10.32604/cmes.2022.019244 [Google Scholar] [PubMed] [CrossRef]
9. Zhang, Z., Chen, J., Guo, Q. (2023). Application of automated guided vehicles in smart automated warehouse systems: A survey. Computer Modeling in Engineering & Sciences, 134(3), 1529–1563. https://doi.org/10.32604/cmes.2022.021451 [Google Scholar] [PubMed] [CrossRef]
10. Qian, L., Li, J., Tang, Q., Liu, M., Yuan, B. et al. (2023). Strong tracking particle filter based on the chi-square test for indoor positioning. Computer Modeling in Engineering & Sciences, 136(2), 1441–1455. https://doi.org/10.32604/cmes.2023.024534 [Google Scholar] [PubMed] [CrossRef]
11. Zhu, J., Chen, H., Fan, Y., Ni, T. (2023). Transductive transfer dictionary learning algorithm for remote sensing image classification. Computer Modeling in Engineering & Sciences, 137(3), 2267–2283. https://doi.org/10.32604/cmes.2023.027709 [Google Scholar] [PubMed] [CrossRef]
Cite This Article
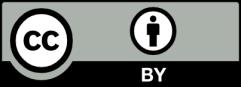