Open Access
ARTICLE
Tensile Strain Capacity Prediction of Engineered Cementitious Composites (ECC) Using Soft Computing Techniques
1 Civil Engineering Department, University of Halabja, Halabja, Iraq
2 University of Sulaimani, College of Engineering, Civil Engineering Department, Sulaimani, Iraq
3 Civil Engineering Department, Komar University of Science and Technology, Sulaimani, Iraq
4 Department of Engineering, Kurdistan Institution for Strategic Studies and Scientific Researches, Sulaimani, Iraq
5 Department of Civil Engineering, American University of Sharjah, Sharjah, 26666, UAE
* Corresponding Author: Rabar H. Faraj. Email:
(This article belongs to the Special Issue: Meta-heuristic Algorithms in Materials Science and Engineering)
Computer Modeling in Engineering & Sciences 2024, 138(3), 2925-2954. https://doi.org/10.32604/cmes.2023.029392
Received 16 February 2023; Accepted 04 August 2023; Issue published 15 December 2023
Abstract
Plain concrete is strong in compression but brittle in tension, having a low tensile strain capacity that can significantly degrade the long-term performance of concrete structures, even when steel reinforcing is present. In order to address these challenges, short polymer fibers are randomly dispersed in a cement-based matrix to form a highly ductile engineered cementitious composite (ECC). This material exhibits high ductility under tensile forces, with its tensile strain being several hundred times greater than conventional concrete. Since concrete is inherently weak in tension, the tensile strain capacity (TSC) has become one of the most extensively researched properties. As a result, developing a model to predict the TSC of the ECC and to optimize the mixture proportions becomes challenging. Meanwhile, the effort required for laboratory trial batches to determine the TSC is reduced. To achieve the research objectives, five distinct models, artificial neural network (ANN), nonlinear model (NLR), linear relationship model (LR), multi-logistic model (MLR), and M5P-tree model (M5P), are investigated and employed to predict the TSC of ECC mixtures containing fly ash. Data from 115 mixtures are gathered and analyzed to develop a new model. The input variables include mixture proportions, fiber length and diameter, and the time required for curing the various mixtures. The model’s effectiveness is evaluated and verified based on statistical parameters such as R2, mean absolute error (MAE), scatter index (SI), root mean squared error (RMSE), and objective function (OBJ) value. Consequently, the ANN model outperforms the others in predicting the TSC of the ECC, with RMSE, MAE, OBJ, SI, and R2 values of 0.42%, 0.3%, 0.33%, 0.135%, and 0.98, respectively.Keywords
Cite This Article
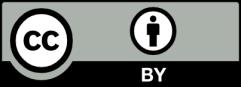