Open Access
ARTICLE
Analytical and Numerical Methods to Study the MFPT and SR of a Stochastic Tumor-Immune Model
1 School of Mathematics and Statistics, Xidian University, Xi’an, 710071, China
2 Innovation Center of Faculty of Mechanical Engineering, Belgrade, 11100, Serbia
* Corresponding Author: Wei Li. Email:
Computer Modeling in Engineering & Sciences 2024, 138(3), 2177-2199. https://doi.org/10.32604/cmes.2023.030728
Received 19 April 2023; Accepted 03 August 2023; Issue published 15 December 2023
Abstract
The Mean First-Passage Time (MFPT) and Stochastic Resonance (SR) of a stochastic tumor-immune model with noise perturbation are discussed in this paper. Firstly, considering environmental perturbation, Gaussian white noise and Gaussian colored noise are introduced into a tumor growth model under immune surveillance. As follows, the long-time evolution of the tumor characterized by the Stationary Probability Density (SPD) and MFPT is obtained in theory on the basis of the Approximated Fokker-Planck Equation (AFPE). Herein the recurrence of the tumor from the extinction state to the tumor-present state is more concerned in this paper. A more efficient algorithm of Back-Propagation Neural Network (BPNN) is utilized in order to testify the correction of the theoretical SPD and MFPT. With the existence of a weak signal, the functional relationship between Signal-to-Noise Ratio (SNR), noise intensities and correlation time is also studied. Numerical results show that both multiplicative Gaussian colored noise and additive Gaussian white noise can promote the extinction of the tumors, and the multiplicative Gaussian colored noise can lead to the resonance-like peak on MFPT curves, while the increasing intensity of the additive Gaussian white noise results in the minimum of MFPT. In addition, the correlation times are negatively correlated with MFPT. As for the SNR, we find the intensities of both the Gaussian white noise and the Gaussian colored noise, as well as their correlation intensity can induce SR. Especially, SNR is monotonously increased in the case of Gaussian white noise with the change of the correlation time. At last, the optimal parameters in BPNN structure are analyzed for MFPT from three aspects: the penalty factors, the number of neural network layers and the number of nodes in each layer.Keywords
In recent years, a varieties of tumor diseases have been observed and brought a revolution in the field of cancer treatment. In order to explore the law of tumor growth and extinction, a good many of researchers dedicate to study the tumor models, the issues including the growth of anti-tumor, the competition between the tumor cells and the immune system [1–5].
Some deterministic tumor models such as Logistic model [6], Gompertz model [7] have been proved to be the powerful models to describe the dynamical evolution of the tumor, these models are not only helpful for understanding the competition between the tumor cells and the immune system, but also beneficial for assisting the tumor’s elimination or extinction. However, in recent decades, scholars began to realize that these deterministic models are not consistent with the reality enough because they ignore the effects of environmental perturbations. In fact, the growth of the malignant tumors relies heavily on the synthesis of the proteins which are very sensitive to the environmental perturbations [8–10]. However, uncertain factors such as body temperature, blood pressure, oxygen level, and others in cells’ microenvironment can bring the random fluctuation to the population of the tumor cells and the immune cells. Therefore, stochastic dynamical models regarding to the tumor and the immune system are gradually proposed instead of the deterministic ones [11–13], in which the mentioned uncertain microenvironment is usually modeled by random noises with a certain probability distribution.
Some valuable works have been achieved for the stochastic tumor or tumor-immune models. In 2012, Li et al. [14] considered a tumor growth model perturbed by a Gaussian white noise together with a dichotomous noise, he used the Mean First Extinction Time (MFET) as an index to analyze the transitions from the steady state to the extinction state of the tumor cells. In 2018, Ochab-Marcinek et al. [15] studied how dichotomous noise and Gaussian white-noise affected the MFET of the cell population in a tumor-immune model. They observed the important dynamical phenomena of Resonance Activation (RA) and Noise Enhanced Stability (NES). In 2020, Han et al. [16] discussed the tumor growth model under the immune surveillance with stochastic fluctuations modelled as Gaussian white noises, he defined the extinction time as the time when the tumor firstly escaped to the extinction state from the steady state, and simulated the effect of different noise parameters on the most probable extinction time. In 2022, the first author of this paper [17] discussed MFET from the steady-state state to the extinction state in a stochastic tumor-immune model with Gaussian white-noises, they discovered the phenomena of the NES as well as Stochastic Resonant (SR).
Based on the current references above, we summarize that the stochastic tumor models especially stochastic tumor-immune models regarding tumor state transition are concentrated on the Gaussian white-noise or dichotomous noise perturbation. However, the significant noise perturbations such as color noise or non-Gaussian noises are seldom mentioned in the existing literature. According to the authors’ knowledge, only [18] considered a tumor growth model perturbed by Gaussian colored noise in 2022, and this paper studied the noise-induced phenomenon of the transition from a steady state to an extinction state by MFET.
In addition, we have to mention that the previous works mainly focus on the issue of the MFPT from the steady state to the extinction state. The reason for this is that people care much about the time for a human body from a tumor-present state to a healthy state. That is, the time probably for a person to be cured well from the tumor state is an important purpose of the tumor treatment. Nevertheless, it is true that tumor recurrence is a typical feature of tumors compared with others disease especially the malignant tumors; how long will it take to be recurrent for a tumor patient after a previous recovery? It is also extremely important issue concerned in the tumor treatment. Therefore, the discussion for MFPT from the extinction state to the steady state in a tumor-immune model is also definitely necessary and significant, this is exactly one of the key innovation of this paper.
Since the MFPT is a significant index used to describe the noise-induced escape process in stochastic tumor-immune models, how to obtain this index and how to solve the governing equations become the following task. Generally, MFPT is governed by a back-forward partial differential equation with nonlinear functional coefficients, the developing methods such as finite difference method [19,20], path integral method [21] and Galerkin method [22,23] are common used to solve a partial differential equation. However, these methods usually have strict requirements for the dimension or domain mesh, the accuracy and computer running time can not be balanced at the same time. Back-Propagation Neural Network (BPNN) has been proved to be a viable, multipurpose and robust computational methodology with solid theoretic support and strong potential applications [24,25]. Many existing studies have shown the approximation ability of BPNN to nonlinear functions as well as the efficiency in solving solution of the nonlinear models [26]. It is interesting to see that BPNN is not much applied to study MFPT problem in the field of the stochastic dynamical system, which inspires us to extend this algorithm to the case of the tumor-immune model.
On the other hand, Stochastic Resonance (SR) is a dynamical phenomenon usually happened in a stochastic dynamical system, refer to the response to an input signal enhanced by the optimal amount of noise. Owing to the role of the SR, it has been mentioned in certain tumor-immune research as one of the indexes to measure the tumor dynamics. The representative works with regard to the SR include that Li et al. [27] derived a theory for the SR by using the two-state approach in 2011, and obtained the corresponding signal-to-noise ratio (SNR). It is found that in subthreshold periodic treatment, weak environmental fluctuations can induce the extinction of the tumor cells. In 2018, Shi et al. [28] considered the SR of asymmetric bistable systems with multiplicative white noise and additive color noise, and studied the influence of parameters on the SR from the perspective of the SNR. In 2021, Guo et al. [29] established the tumor growth model excited by a Levy noise and a Gaussian white noise, he studied the relationship between the SNR and the noise intensity by a kind of numerical simulation, and obtained a conclusion that all parameters in the model can induce the SR. It can be seen that the SNR is the most commonly used index in SR research. Therefore, this paper also uses SNR to describe and analyze the phenomenon of the SR.
To sum up, this paper aims to improve the current research about the stochastic tumor-immune model from four points: (1) Considering color noise to be the random perturbation in the cell microenvironment; (2) Focusing on the transition from the tumor extinction to the tumor recurrence; (3) Using two methods of the analytical process and numerical algorithm to obtain the indexes of the MFPT and the SR; (4) Applying the BPNN to solve the governing the MFPT and the SR equations to testify the correction and effectiveness of the proposed methods.
2 Stochastic Tumor-Immune Model with Correlated Noises
The interaction between the tumor cells and the immune system can be illustrated by Fig. 1 based on Michaelis-Menten enzymatic reaction. Correspondingly, the mathematical model governing the tumor growth under the immune surveillance can be expressed by a differential equation after a series of mathematical procedure [17] as
where
where
and one unstable state solution
Figure 1: The interaction between tumor cells and immune system
Considering that most biochemical systems in reality are open systems, they often exchange the information and the energy through the interaction with the outside world. So it is necessary to consider the impact of the environmental fluctuations on the systems, which are often modeled as noise in the mathematical models. In this section, we will model the environmental fluctuations in the tumor-immune model Eq. (1) as multiplicative Gaussian colored noise
where the noises
in which
and
where
3 Stochastic Dynamics for SPD, MFPT, and SR
3.1 Theoretic Solution for SPD
Due to the existence of the colored noises, we know that Eq. (6) is a one-dimensional non-Markovian process, which means that the Fokker-Planck equation describing the probability density of the tumor cells cannot be obtained directly. Therefore, we will deal with this problem through the theory of Unified Colored Noise Approximation (UCNA) to obtain an approximate Markovian process and AFPE [30]. For convenience, we rewrite Eq. (6) as
Correspondingly,
Firstly, through some transformations associated with the UCNA method, we can obtain the following one-dimensional approximate Markovian process from Eqs. (6)–(9), that is
where
Instead, the expressions of
Finally, the AFPE corresponding to Eq. (6) can be described as
where
so the expressions corresponding to
Basically, the evolution of the tumor cells under the immune surveillance is a long-term process, so we generally concerns the stationary probability density of the tumor cells, which means the case of
its boundary condition and normalization condition are, respectively
and
where
where
in which
Substitute
3.2 Theoretical Result for MFPT
Generally, MFPT is defined as the average transition time of the system response from one state to another state. The average time from the steady state to an extinction state or from a tumor-free state to a tumor-present state are most concerned issues in the stochastic tumor-immune model. In this section, we will pay attention to observe whether the environmental perturbation can result in the tumor recurrence. Correspondingly, we will focus on studying the transition from the extinction state
It has been proved [31] that MFPT is controlled by a backward Kolomogorov equation with certain boundary conditions and initial conditions. Denote the MFPT by
with boundary conditions
Regarding the proposed stochastic tumor-immune model, left boundary
If the noise intensity
Substitute the potential function
3.3 Theoretical Results of SR and SNR
In the actual environment of the tumor growth, noises have an important impact on the evolutionary characteristics for the tumor and the immune systems. Therefore, except for SPD and MFPT, the influence of noises on SR has also been widely concerned by scholars. The background of SR phenomenon has been involved in many subjects such as physics [33], chemistry [34], biology [35], communication [36] and electronics [37], and SR has been proven to be a kind of common physical phenomenon in nature. Among the various measures describing SR, SNR is one of the most representative index. Therefore, this section will discuss the SNR of the stochastic tumor-immune model Eq. (6).
Considering the periodic effects caused by the environmental fluctuations, we take an additive periodic signal
In this way, the nonlinear function
where A and
in which the expressions of the drift function
When
where the expression of the generalized potential function
in which
Review the method proposed in Section 3.2, we can obtain the MFPT of the tumor cells from the extinction state
According to the the inverse relationship between the escape rate and the MFPT, the escape rates of the tumor cells between the extinction state and the tumor-present state are obtained as follows:
In order to understand the characteristics of the SR, it is necessary to calculate the SRN for Eq. (6) with multiplicative Gaussian colored noise and additive Gaussian white noise in the output signal power spectrum.
Using signal output
where the expressions of
in which
Finally, we get the expression of the SNR for the model (29)
where
4 Back-Propagation Neural Network Algorithm
The unique nonlinear simulation and adaptive processing abilities of the BPNN make it widely used in the fields of pattern recognition, intelligent control, combinatorial optimization and so on. In recent years, BPNN has been continuously combined with other traditional methods, it has played an important role in a good many fields with its superior speed and robustness [43,44].
In this section, we are going to use the combination of the BPNN algorithm together with the backward Kolomogorov equation to numerically simulate the SPD and the MFPT, in order to obtain the transition from the extinction state to the tumor-present state of the tumor cells.
4.1 Structure of the Neural Network
Supposing there are
where
Figure 2: The structure of BPNN
4.2 Construct the Loss Function
Loss function is the most basic element in the neural network. The definition and optimization of the loss function will directly affect the output of the neural network. Therefore, we will realize the simulation of SPD and MFPT by defining different loss functions, and write the simulation results as
where
For the case of using neural network to simulate MFPT, the backward Kolomogorov Eq. (25) and the corresponding boundary conditions Eq. (26) are considered as the governing conditions with penalty factors
where
in which the output
5 Theoretical and BPNN Results
5.1 Effect of the Noises on SPD
The effect of environmental fluctuations on the growth of the tumor cells is an important issue in the study of tumor-immune models. Therefore, in this section, we will analyze the influence of different noise parameters on the tumor by way of the SPDs, and use BPNN to simulate SPDs with the same parametric values as well.
The curves in Fig. 3 describe the changes of SPDs with the different noise parameters. Fig. 3a displays the effect of multiplicative noise intensity
Figure 3: Influence of different noise parameters on SPDs with
5.2 Effect of the Noises on MFPT
In addition to the SPD, the performance of the MFPT is also of great significance to the study of tumor immunity, which is a remarkable index to indicate the average transition time from one state to another state. In general, the transition from a steady state to a zero state means that the patient has been cured and the tumor cells are extinct. The transition from a zero state to a steady state, however, means that the tumor has been recurred and the tumor cells appears again. Correspondingly, the knowledge about the recurrence time is especially important for a patient to take all reasonable precaution. Therefore, in this subsection, we will mainly study the effect of environmental fluctuations on the MFPT of the tumor cells from the extinction to the tumor-present state. Based on the theoretical solution of Eq. (28) and neural network solution Eq. (47) for MFPT, Fig. 4 provides the influence of different noise parameters on MFPT.
Figure 4: MFPT is a function of
Firstly, Fig. 4a depicts the change of the MFPT with the intensity of the multiplicative Gaussian colored noise
Different from Figs. 4 and 5 mainly describes the influence of the multiplicative noise intensity
Figure 5: MFPT is a function of
At the end of this section, we discuss the relationship between the MFPT and the correlation time
Figure 6: MFPT is a function of
Fig. 7a shows the variation of the SNR with the multiplicative noise intensity
Figure 7: SNR performance under the influence of different noise parameters with
6 Discussion on Optimal BPNN Structure
In this section, our main work is to analyze the feasibility and effectiveness of using BPNN to simulate MFPT. There are three main factors affecting the simulation effect of the neural network, which are the number of hidden layers, the number of nodes in each hidden layer and the value of penalty factors in the loss function. In addition, in order to better show the simulation effect of BPNN on MFPT, we determine that the parameter values in model Eq. (6) are
Firstly, the penalty factors can adjust the proportion of different control conditions in the loss function. So it is necessary to discuss the values of the best penalty factors in the loss function
where
Table 1 indicates that when
Secondly, the number of hidden layers and the number of nodes in the hidden layers determine the structure of the neural network, and their influence on the operation efficiency and accuracy of the neural network is also very important. In order to select the optimal number of hidden layers and nodes, we consider their impact on the performance of the loss function. From Fig. 8, we can find that in the process of gradually increasing the number of hidden layers of BPNN from
Figure 8: The comparison of loss functions with different number of hidden layers
Finally, Fig. 9 describes the influence of the number of nodes in the BPNN hidden layer on the neural network simulation results. We find that, compared with the number of hidden layers, the number of nodes has less effect on the performance of the loss function. However, as the number of nodes in each layer increases from
Figure 9: The comparison of loss functions with different number of nodes
To sum up, through the discussion of the experimental results, we have obtained the optimal loss function and neural network structure for the tumor-immune model as
Figure 10: Accuracy diagram of BPNN simulation results
In conclusion, this paper focuses on the dynamic properties of the stochastic tumor-immune model perturbed by multiplicative Gaussian colored noise and additive Gaussian white noise, including the effects of noise parameters on SPD, MFPT and SR. Firstly, the expressions of SPD and MFPT were obtained by using the generalized potential function. Then, using the escape rates of tumor cells between the extinction and the tumor-present state, we obtained the exact solution of SNR which was the most representative index of SR. These results showed that in this model, the influence of noise intensity and correlation time on MFPT was complex and diverse. The possibility of tumor recurrence could not be simply controlled by a certain noise parameter. For example, in a smaller correlation time
Acknowledgement: The authors wish to express their appreciation to the reviewers for their helpful suggestions which greatly improved the presentation of this paper.
Funding Statement: This work was supported by National Natural Science Foundation of China (Nos. 12272283, 12172266).
Author Contributions: The authors confirm contribution to the paper as follows: study conception and design: Ying Zhang, Wei Li, Guidong Yang, Snezana Kirin; data collection: Ying Zhang, Guidong Yang; analysis and interpretation of results: Wei Li, Snezana Kirin; draft manuscript preparation: Ying Zhang, Wei Li. All authors reviewed the results and approved the final version of the manuscript.
Availability of Data and Materials: The datasets generated during the current study are not publicly available but are available from the corresponding author.
Conflicts of Interest: The authors declare that they have no conflicts of interest to report regarding the present study.
References
1. Leschiera, E., Lorenzi, T., Shen, S., Almeida, L., Audebert, C. (2022). A mathematical model to study the impact of intra-tumour heterogeneity on anti-tumour CD8+ T cell immune response. Journal of Theoretical Biology, 538, 111028. [Google Scholar] [PubMed]
2. Das, P., Das, P., Mukherjee, S. (2020). Stochastic dynamics of Michaelis–Menten kinetics based tumor-immune interactions. Physica A, 541, 123603. [Google Scholar]
3. Chen, Z. H., Zha, H. J., Shu, Z. Q., Ye, J. Y., Pan, J. J. (2022). Assess medical screening and isolation measures based on numerical method for COVID-19 epidemic model in Japan. Computer Modeling in Engineering & Sciences, 130(2), 841–854. https://doi.org/10.32604/cmes.2022.017574 [Google Scholar] [CrossRef]
4. Hao, M., Jia, W., Wang, L., Li, F. (2022). Most probable trajectory of a tumor model with immune response subjected to asymmetric Lévy noise. Chaos Solitons Fractals, 165, 112765. [Google Scholar]
5. Rihan, F. A., Udhayakumar, K. (2023). Fractional order delay differential model of a tumor-immune system with vaccine efficacy: Stability, bifurcation and control. Chaos Solitons Fractals, 173, 113670. [Google Scholar]
6. Atuegwu, N. C., Arlinghaus, L. R., Li, X., Chakravarthy, A. B., Abramson, V. G. et al. (2013). Parameterizing the logistic model of tumor growth by DW-MRI and DCE-MRI data to predict treatment response and changes in breast cancer cellularity during neoadjuvant chemotherapy. Translational Oncology, 6(3), 256–264. [Google Scholar] [PubMed]
7. Patmanidis, S., Charalampidis, A. C., Kordonis, I., Mitsis, G. D., Papavassilopoulos, G. P. (2017). Comparing methods for parameter estimation of the Gompertz tumor growth model. IFAC-PapersOnLine, 50(1), 12203–12209. [Google Scholar]
8. Liao, T. (2022). The impact of plankton body size on phytoplankton-zooplankton dynamics in the absence and presence of stochastic environmental fluctuation. Chaos Solitons Fractals, 154, 111617. [Google Scholar]
9. Qi, H., Meng, X. (2021). Mathematical modeling, analysis and numerical simulation of HIV: The influence of stochastic environmental fluctuations on dynamics. Mathematics and Computers in Simulation, 187, 700–719. [Google Scholar]
10. Deng, Y., Liu, M. (2020). Analysis of a stochastic tumor-immune model with regime switching and impulsive perturbations. Applied Mathematical Modelling, 78, 482–504. [Google Scholar]
11. Fiasconaro, A., Ochab-Marcinek, A., Spagnolo, B., Gudowska-Nowak, E. (2008). Monitoring noise-resonant effects in cancer growth influenced by external fluctuations and periodic treatment. European Physical Journal B, 65(3), 435–442. [Google Scholar]
12. Yin, A., Moes, D. J. A., van Hasselt, J. G., Swen, J. J., Guchelaar, H. J. (2019). A review of mathematical models for tumor dynamics and treatment resistance evolution of solid tumors. CPT: Phamacometrics and System Phamacology, 8(10), 720–737. [Google Scholar]
13. Fiasconaro, A., Spagnolo, B., Ochab-Marcinek, A., Gudowska-Nowak, E. (2006). Co-occurrence of resonant activation and noise-enhanced stability in a model of cancer growth in the presence of immune response. Physical Review E, 74(4), 041904. [Google Scholar]
14. Li, D., Xu, W., Sun, C., Wang, L. (2012). Stochastic fluctuation induced the competition between extinction and recurrence in a model of tumor growth. Physics Letter A, 376(22), 1771–1776. [Google Scholar]
15. Ochab-Marcinek, A., Fiasconaro, A., Gudowska-Nowak, E., Spagnolo, B. (2006). Coexistence of resonant activation and noise enhanced stability in a model of tumor-host interaction: Statistics of extinction times. Acta Physica Polonica B, 37, 1651–1666. [Google Scholar]
16. Han, P., Xu, W., Wang, L., Zhang, H., Ma, S. (2020). Most probable dynamics of the tumor growth model with immune surveillance under cross-correlated noises. Physica A, 547, 123833. [Google Scholar]
17. Li, W., Zhang, Y., Huang, D., Rajic, V. (2022). Study on stationary probability density of a stochastic tumor-immune model with simulation by ANN algorithm. Chaos Solitons Fractals, 159, 112145. [Google Scholar]
18. Hua, M., Wu, Y. (2022). Transition and basin stability in a stochastic tumor growth model with immunization. Chaos Solitons Fractals, 111953. [Google Scholar]
19. Jiang, Y. (2015). A new analysis of stability and convergence for finite difference schemes solving the time fractional Fokker–Planck equation. Applied Mathematical Modelling, 39(3), 1163–1171. [Google Scholar]
20. Sepehrian, B., Radpoor, M. K. (2015). Numerical solution of non-linear Fokker–Planck equation using finite differences method and the cubic spline functions. Applied Mathematics and Computation, 262, 187–190. [Google Scholar]
21. Mulakala, C., Kaznessis, Y. N. (2009). Path-integral method for predicting relative binding affinities of protein-ligand complexes. Journal of the American Chemical Society, 131(12), 4521–4528. [Google Scholar] [PubMed]
22. Sun, Z., Carrillo, J. A., Shu, C. W. (2018). A discontinuous Galerkin method for nonlinear parabolic equations and gradient flow problems with interaction potentials. Journal of Computational Physics, 352, 76–104. [Google Scholar]
23. Zheng, Y., Li, C., Zhao, Z. (2010). A fully discrete discontinuous galerkin method for nonlinear fractional Fokker-Planck equation. Mathematical Problems in Engineering, 2010, 279038. [Google Scholar]
24. Bararnia, H., Esmaeilpour, M. (2022). On the application of physics informed neural networks (PINN) to solve boundary layer thermal-fluid problems. International Communications in Heat and Mass Transfer, 132, 105890. [Google Scholar]
25. Santosh, T., Soni, R. K., Eswaraiah, C., Kumar, S. (2022). Application of artificial neural network method to predict the breakage properties of PGE bearing chromite ore. Advanced Powder Technology, 33(3), 103450. [Google Scholar]
26. Li, S., Yang, Y. (2021). A recurrent neural network framework with an adaptive training strategy for long-time predictive modeling of nonlinear dynamical systems. Journal of Sound and Vibration, 506, 116167. [Google Scholar]
27. Li, D., Xu, W., Guo, Y., Xu, Y. (2011). Fluctuations induced extinction and stochastic resonance effect in a model of tumor growth with periodic treatment. Physics Letters A, 375(5), 886–890. [Google Scholar]
28. Shi, P., Xia, H., Han, D., Fu, R., Yuan, D. (2018). Stochastic resonance in a time polo-delayed asymmetry bistable system driven by multiplicative white noise and additive color noise. Chaos Solitons Fractals, 108, 8–14. [Google Scholar]
29. Guo, Y., Yao, T., Wang, L., Tan, J. (2021). Lévy noise-induced transition and stochastic resonance in a tumor growth model. Applied Mathematics Modelling, 94, 506–515. [Google Scholar]
30. Cao, L., Wu, D. J., Ke, S. Z. (1995). Bistable kinetic model driven by correlated noises: Unified colored-noise approximation. Physical Review E, 52(3), 3228. [Google Scholar]
31. Wu, J. L., Duan, W. L., Luo, Y., Yang, F. (2020). Time delay and non-Gaussian noise-enhanced stability of foraging colony system. Physica A, 553, 124253. [Google Scholar]
32. Jia, Y., Yu, S. N., Li, J. R. (2000). Stochastic resonance in a bistable system subject to multiplicative and additive noise. Physical Review E, 62(2), 1869–1878. [Google Scholar]
33. Cheng, G., Liu, W., Gui, R., Yao, Y. (2020). Sine-wiener bounded noise-induced logical stochastic resonance in a two-well potential system. Chaos Solitons Fractals, 131, 109514. [Google Scholar]
34. Wang, B., Gao, F., Gupta, M. K., Królczyk, G., Gardoni, P. et al. (2022). Risk analysis of a flywheel battery gearbox based on optimized stochastic resonance model. Journal of Energy Storage, 52, 104926. [Google Scholar]
35. Yamazaki, H., Lioumis, P. (2022). Stochastic resonance at early visual cortex during figure orientation discrimination using transcranial magnetic stimulation. Neuropsychologia, 168, 108174. [Google Scholar] [PubMed]
36. Zhou, Z., Yu, W., Wang, J., Liu, M. (2022). A high dimensional stochastic resonance system and its application in signal processing. Chaos Solitons Fractals, 154, 111642. [Google Scholar]
37. Jiao, S., Gao, R., Zhang, D., Wang, C. (2022). A novel method for UWB weak signal detection based on stochastic resonance and wavelet transform. Chinese Journal of Physics, 76, 79–93. [Google Scholar]
38. Ning, L. J., Xu, W., Yang, X. L. (2007). The mean first-passage time for an asymmetric bistable system driven by multicative and additive noise with colored correlations. Acta Physical Sinica, 56(1), 25–29. [Google Scholar]
39. Zan, W., Xu, Y., Metzler, R., Kurths, J. (2021). First-passage problem for stochastic differential equations with combined parametric gaussian and lévy white noises via path integral method. Journal of Computational Physics, 435, 110264. [Google Scholar]
40. Yin, H., Wen, X. (2019). First passage times and minimum actions for a stochastic minimal bistable system. Chinese Journal of Physics, 59, 220–230. [Google Scholar]
41. Boehm, U., Cox, S., Gantner, G., Stevenson, R. (2021). Fast solutions for the first-passage distribution of diffusion models with space-time-dependent drift functions and time-dependent boundaries. Journal of Mathematical Psychology, 105, 102613. [Google Scholar]
42. Hohenegger, C., Durr, R., Senter, D. (2017). Mean first passage time in a thermally fluctuating viscoelastic fluid. Journal of Non-Newtonian Fluid Mechanics, 242, 48–56. [Google Scholar]
43. Wei, J. L., Wu, G. C., Liu, B. Q., Zhao, Z. G. (2022). New semi-analytical solutions of the time-fractional Fokker-Planck equation by the neural network method. Optik, 168896. [Google Scholar]
44. Guo, X., Zhang, Y. D., Lu, S., Lu, Z. (2022). A survey on machine learning in COVID-19 diagnosis. Computers, Materials & Continua, 130(1), 23–71. https://doi.org/10.32604/cmes.2021.017679 [Google Scholar] [CrossRef]
Cite This Article
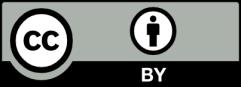