Open Access
ARTICLE
Transformer-Aided Deep Double Dueling Spatial-Temporal Q-Network for Spatial Crowdsourcing Analysis
Department of Computing, Hangzhou Dianzi University, Hangzhou, 310018, China
* Corresponding Author: Dongyang Ou. Email:
(This article belongs to the Special Issue: Machine Learning Empowered Distributed Computing: Advance in Architecture, Theory and Practice)
Computer Modeling in Engineering & Sciences 2024, 139(1), 893-909. https://doi.org/10.32604/cmes.2023.031350
Received 08 June 2023; Accepted 11 October 2023; Issue published 30 December 2023
Abstract
With the rapid development of mobile Internet, spatial crowdsourcing has become more and more popular. Spatial crowdsourcing consists of many different types of applications, such as spatial crowd-sensing services. In terms of spatial crowd-sensing, it collects and analyzes traffic sensing data from clients like vehicles and traffic lights to construct intelligent traffic prediction models. Besides collecting sensing data, spatial crowdsourcing also includes spatial delivery services like DiDi and Uber. Appropriate task assignment and worker selection dominate the service quality for spatial crowdsourcing applications. Previous research conducted task assignments via traditional matching approaches or using simple network models. However, advanced mining methods are lacking to explore the relationship between workers, task publishers, and the spatio-temporal attributes in tasks. Therefore, in this paper, we propose a Deep Double Dueling Spatial-temporal Q Network (D3SQN) to adaptively learn the spatial-temporal relationship between task, task publishers, and workers in a dynamic environment to achieve optimal allocation. Specifically, D3SQN is revised through reinforcement learning by adding a spatial-temporal transformer that can estimate the expected state values and action advantages so as to improve the accuracy of task assignments. Extensive experiments are conducted over real data collected from DiDi and ELM, and the simulation results verify the effectiveness of our proposed models.Graphical Abstract
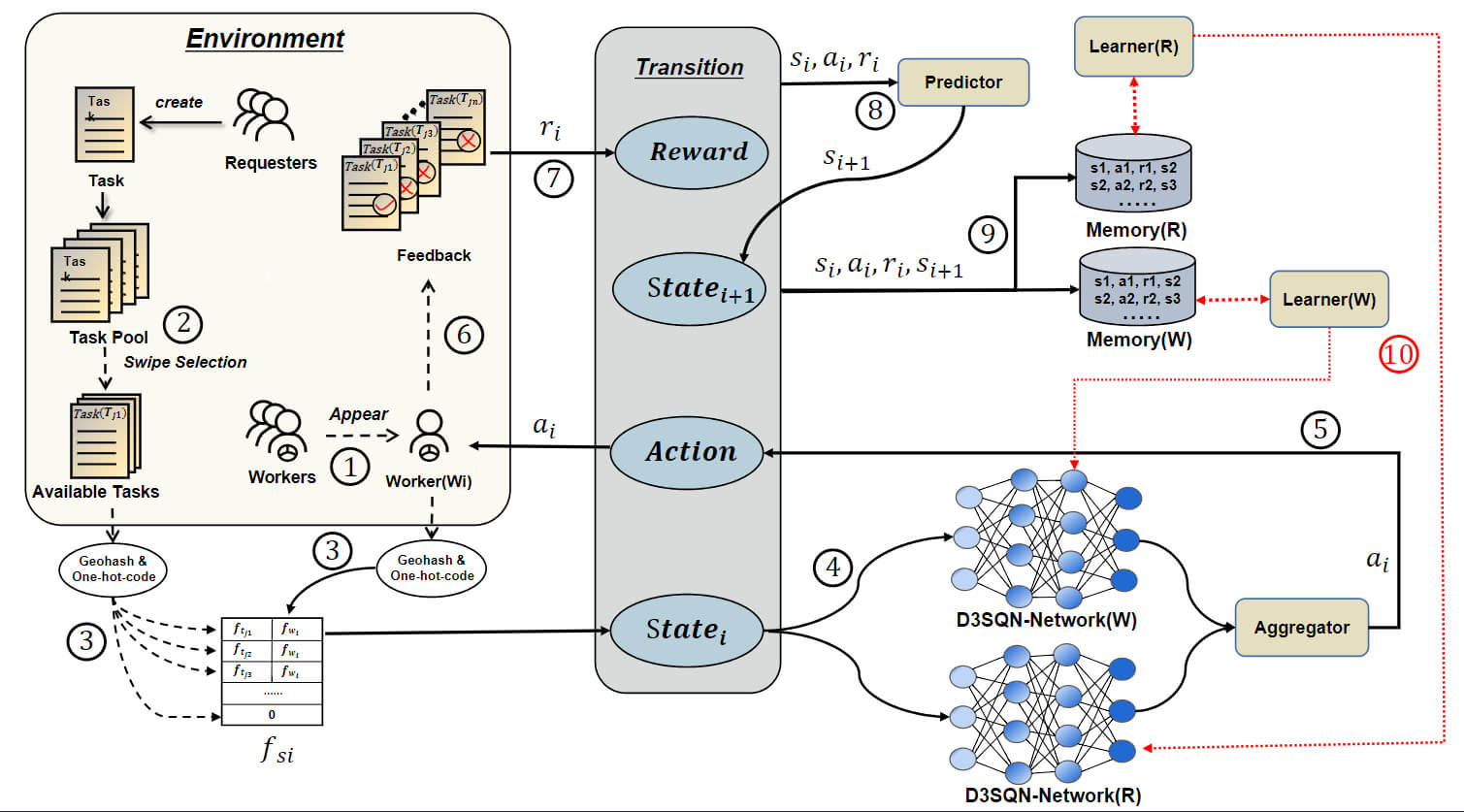
Keywords
Cite This Article
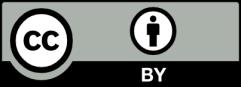