Open Access
ARTICLE
NFT Security Matrix: Towards Modeling NFT Ecosystem Threat
1 Key Laboratory of Trustworthy Distributed Computing and Service, Beijing University of Posts and Telecommunications, Beijing, 100876, China
2 Zhongguancun Laboratory, Beijing, 100094, China
3 Institute of Information Engineering, Chinese Academy of Sciences, Beijing, 100085, China
* Corresponding Author: Jie Yin. Email:
(This article belongs to the Special Issue: The Bottleneck of Blockchain Techniques: Scalability, Security and Privacy Protection)
Computer Modeling in Engineering & Sciences 2024, 139(3), 3255-3285. https://doi.org/10.32604/cmes.2024.043855
Received 14 July 2023; Accepted 20 September 2023; Issue published 11 March 2024
Abstract
Digital assets have boomed over the past few years with the emergence of Non-fungible Tokens (NFTs). To be specific, the total trading volume of digital assets reached an astounding $55.5 billion in 2022. Nevertheless, numerous security concerns have been raised by the rapid expansion of the NFT ecosystem. NFT holders are exposed to a plethora of scams and traps, putting their digital assets at risk of being lost. However, academic research on NFT security is scarce, and the security issues have aroused rare attention. In this study, the NFT ecological process is comprehensively explored. This process falls into five different stages encompassing the entire lifecycle of NFTs. Subsequently, the security issues regarding the respective stage are elaborated and analyzed in depth. A matrix model is proposed as a novel contribution to the categorization of NFT security issues. Diverse data are collected from social networks, the Ethereum blockchain, and NFT markets to substantiate our claims regarding the severity of security concerns in the NFT ecosystem. From this comprehensive dataset, nine key NFT security issues are identified from the matrix model and then subjected to qualitative and quantitative analysis. This study aims to shed light on the severity of NFT ecosystem security issues. The findings stress the need for increased attention and proactive measures to safeguard the NFT ecosystem.Keywords
In recent years, blockchain technology has been widely used in finance, public services, medical care, privacy protection, Internet of Things and many other fields. It can help improve efficiency, reduce costs, enhance security and protect privacy in these areas. Raj et al. [1] proposed an access control for a healthcare monitoring system using blockchain-based smart contracts. The proposed method can effectively improve the privacy and security of patient data. Gupta et al. [2] used blockchain technology to solve the inherent difficulties of data storage and retrieval in healthcare cloud-based cyber-physical systems. Far et al. [3] analyzed Distributed Autonomous Organization (DAOs), and introduced Blockchain-Based Anonymous Reporting (BBAR) as a collective monitoring mechanism in DAOs with its requirements and implementation methods. The author also explored the Metaverse, which is a digital environment built on Web 3.0 technologies and blockchain, their research suggests that the Metaverse will become increasingly popular, with the virtual world incorporating more aspects of the physical world [3]. It is not difficult to see that blockchain technology has received extensive attention and application.
NFT (Non-Fungible Token) [4] refers to an architecture developed based on blockchain technology, representing the unique and tamper-proof cryptographic proofs of digital ownership. It serves as a decentralized certificate of ownership in terms of virtual or physical assets. The core value of NFT lies in the digitization of content assets and the development of digital identity, asset ownership, and proof of ownership in decentralized environments. In the future, NFTs may serve as a form of soulful tokens, acting as bridges and hopes connecting the digital and physical spaces. In the digital realm, NFTs are dependent on underlying public chains (e.g., Ethereum), smart contracts, digital wallets, and storage protocols as the foundational infrastructure. Issues on top of this infrastructure are cryptocurrencies, comprising native cryptocurrencies and fungible tokens, which inject an economic model into the NFT ecosystem. This economic model provides incentives for NFT creators in terms of artistic creation and intellectual property protection, facilitates payment methods for NFT trading, and creates an economic cycle in the NFT ecosystem. This, in turn, promotes liquidity and trading activities in the NFT market. Additionally, individuals such as Vitalik Buterin [5], the founder of Ethereum, have introduced “soul-binding tokens,” a type of non-transferable NFT that directly binds personal identities to the digital space (Fig. 1).
Figure 1: Architecture of NFTs ecosystem
NFT, an emerging concept in the digital asset field, has been leaping forward over the past few years. The trading volume of the NFT market has explosively surged, such that a multitude of NFT investors across a wide range of categories (e.g., digital avatars, music, sports, and gaming items) have been attracted. The total trading volume of NFTs reached $55.5 billion in 2022, marking an increase of 175% compared with the previous year [6]. For instance, the iconic NFT project CryptoPunks (Fig. 2), created by Larva Labs in 2017, is inspired by London’s punk scene, cyberpunk movement, and electronic music art. As of June 01, 2023, CryptoPunks has gained a total trading volume of $2.58 billion. To be specific, the most expensive single CryptoPunk, #5822, was sold for approximately $23.7 million in February 2022. In general, from the primary market to the secondary market, the daily trading volume of NFTs has reached millions or even billions of dollars. Furthermore, the number of NFT holders has been rising rapidly, with a growing participation of individuals, celebrities, as well as institutions in NFT trading and creation. A vast ecosystem has been formed around NFTs, where a wide variety of participants and activities are involved.
Figure 2: CryptoPunks trading records
The prosperous development of NFTs has also triggered a series of security issues. Fraud by NFT issuers has been reported as one of the most common security issues. NFT holders cannot restrain the NFT issuer, and the issuer can easily exit the NFT project, or become non-responsive after selling NFTs, which is known as “rug-pull” or “soft rug-pull”. For instance, Pixelmon NFT issuer serves as a typical example of a soft rug-pull scam. The issuer initially promised to build an AAA-rated metaverse game with Pixelmon NFTs, whereas the delivered edition was of poor quality and was exposed as NFTs created by artists in a day [7]. Subsequently, in February 2022, the project team orchestrated a soft rug-pull scam, defrauding $71.4 million. Targeted NFT phishing attacks are considered another common security issue. A considerable number of NFT holders change their social network avatars as their NFTs (e.g., Bored Ape Yacht Club (BAYC) [8] or CryptoPunk [9]), whereas this practice can make them vulnerable to targeted attacks. Attackers employ social engineering techniques (e.g., luring and phishing) to steal NFT assets. On April 01, 2022, Jay Chou, a renowned musician, had his BAYC NFT, #3738, worth over $450,000, stolen through a phishing attack [10].
Although the security issues existing in the NFT ecosystem should be urgently addressed, policymakers have only recently begun to focus on NFT security and advocate for certain security policies. On April 24, 2023, the Information Technology and Innovation Foundation (ITIF) released a report titled “NFTs: US Policies and Priorities in 2023” [11]. The report suggests that the United States should take further important steps to tackle down the potential risks and challenges of NFT technology. It highlights the need for efforts from legislators and regulatory agencies to address policy issues (e.g., appropriate financial regulations, Intellectual Property (IP) rights, consumer protection, energy consumption, privacy, and content moderation). The government is urged to develop a joint analysis center to monitor illegal activities on public blockchains, such that the enforcement capabilities of federal agencies can be enhanced. Moreover, academic research on NFT security remains at its preliminary stages. However, the security issues surrounding NFTs have not aroused extensive attention and recognition, which serves as the motivation behind this study. The work of Das et al. [4] has been recognized as one of the crucial literature contributions to NFT security. They have systematically overviewed how the NFT ecosystem operates and categorized NFT security issues into three types (i.e., security risks in NFT marketplaces, security issues regarding external entities, and malicious user transaction behaviors). They have elucidated the specific manifestations of each type of security issue. However, their coverage of the NFT ecosystem is incomplete. For instance, the behavior of NFT issuers on social networks and NFT decentralized finance have not been mentioned.
The paper’s objective is to systematically study the current security issues in the NFT ecosystem so that the industry and the research community can gain a clearer global vision and future research direction based on our work. Accordingly, the composition of the NFT ecosystem is clarified in this paper, with a focus placed on the lifecycle of NFTs. Moreover, the NFT process falls into five stages, and the corresponding security issues are analyzed at the respective stage. Notably, the following contributions are elucidated as follows:
• In-depth analysis of the NFT ecosystem based on the NFT lifecycle. From the perspective of the NFT lifecycle, the processes are divided in the NFT ecosystem into five stages (i.e., release, deployment, minting, circulation, and derivative). The respective stage is thoroughly analyzed, with a focus on its key aspects (Section 3).
• Proposing a novel matrix model for NFT security issues. NFT security issues are classified based on the different malicious actors involved, i.e., the malicious behaviors of NFT issuers and attackers. Furthermore, the above-mentioned NFT security issues are analyzed in depth and categorized into the different stages of the NFT lifecycle. On that basis, a comprehensive matrix model is developed for NFT security issues (Section 4).
• Utilization of a comprehensive and multi-dimensional data collection approach. Diverse data are collected from various sources (e.g., Social Networks, Ethereum blockchain, and NFT markets). The above-described data types encompass a wide range of information. This data is integrated and processed, such that a correlation between NFT blockchain data and social network data is established. This correlation will help bridge the gap between Web3 data and Web2 data, such that a novel approach is generated to analyze and gain insights into the NFT ecosystem (Section 5).
• Conducting qualitative and quantitative analysis of NFT security issues. Nine key NFT security issues are selected from different stages of the NFT lifecycle for measurement and analysis. To be specific, a focus is placed on security issues regarding NFT issuers on social networks, and qualitative and quantitative analysis is conducted based on dimensions (e.g., anonymity, accessibility, activity, and credibility). In the above-described analysis, a novel approach to examining social network account behavior is adopted to expose scams in the NFT industry (Section 5).
The rest of this study is organized as follows. In Section 2, the working mechanism of NFTs is discussed, and the existing relevant research on NFT security is overviewed. In Section 3, the NFT ecological process is proposed, the NFT life cycle is divided into five stages, and the business logic of the respective stage is explained. In Section 4, NFT security issues are we classified, summarized, and refined into the NFT security issue matrix model. In Section 5, a focus is placed on the measurement of NFT security issues. Lastly, in Section 6, the conclusion of this study is drawn by summarizing the existing research.
Smart contracts are at the core of NFTs since they are automated codes running on a blockchain that define the attributes, ownership, and transactional behavior of NFTs. Typically, NFT creators follow recommended NFT smart contract standard protocols from the Ethereum developer community to create and deploy their NFTs. On that basis, the corresponding NFT smart contract addresses are generated. Different NFT smart contract addresses represent a wide variety of NFTs, making the contract address the unique identifier for distinguishing NFT varieties, instead of the NFT image. The most extensively employed smart contract standards currently comprise ERC721 [12] and ERC1155 [13], defining the basic interfaces, extension interfaces, and metadata interfaces for NFTs.
Metadata refers to a crucial component of NFTs since it associates information regarding the NFT assets, which comprise the name, description, and most importantly, the storage location of the digital asset (e.g., an image, audio, or video file). By calling the smart contract interface
Minting refers to a core business function of NFTs, aiming at tokenizing digital assets by linking them to the blockchain through metadata and assigning a unique TokenID. It is noteworthy that no standardized smart contract interface has been established for minting NFTs, and the minting interface is implemented by individual NFT project developers.
The example of BAYC NFT illustrates the working mechanism of NFTs (Fig. 3). First, the BAYC project deploys the NFT smart contract code on the Ethereum blockchain while associating the NFT metadata stored on the blockchain with the smart contract based on the
Figure 3: Working mechanism of an NFT
NFT, as a relatively new technology over the past few years, has led to research primarily focusing on the security aspects of the NFT ecosystem,transactions and NFT anti-fraud.
In terms of NFT ecosystem security, Dipanjan et al. [4] systematized how the NFT ecosystem works. They evaluated the deficiencies and security risks in NFT marketplaces in the ecosystem, vulnerabilities caused by external entities, and malicious user transaction behaviors. Additionally, they evaluated the potential number of counterfeit NFTs based on NFT collection names and NFT image dimensions. Wang et al. [14] emphasized the NFT ecosystem model and proposed an NFT threat assessment model based on STRIDE. Gupta et al. [15] categorized NFT security risks into three types, including Layer 8 risks (i.e., risks beyond the Open Systems Interconnection (OSI) seven-layer model), absence or failure risks, which are primarily related to hacker attacks or transaction platform errors, as well as external risks (e.g., regulatory and policy risks). Wang et al. [16] conducted the vulnerability analysis on NFTs and their associated digital assets. From the perspectives of NFT storage, accessibility, and duplicability, they measured and evaluated 12,353 NFT smart contracts on the Ethereum network. Their analysis revealed that 25.24% of NFT smart contracts on Ethereum are inaccessible, and 21.48% of NFT smart contracts are correlated with duplicate assets. However, none of the above-mentioned studies have systematically explored NFT security issues based on the NFT ecological processes. Therefore, in this paper, we will categorize the security issues based on each stage of the NFT ecological process.
In terms of NFT transaction security, Wen et al. [17] proposed a visualization-based detection and analysis method termed NFTDisk to identify transaction manipulation behaviors in NFT markets. They validated the effectiveness and usability of NFTDisk through two case studies and in-depth user interviews with 14 real NFT investors. Song et al. [18] presented a data mining and machine learning-based method to identify abnormal transaction behaviors in NFT markets. They extract 26 features from dimensions (e.g., the network graph of NFT transactions, transaction volume information, and transaction frequency). Using the K-means clustering algorithm, they grouped wallet addresses with similar behaviors and analyzed potential wallet addresses engaged in transaction manipulation. Their findings revealed that transaction manipulation wallet addresses account for 5.38% of the NFT market. Pelechrinis et al. [19] developed a model to estimate the profit obtained from selling specific collectibles on the NBA TopShot NFT marketplace. To be specific, they employed Random Forest to model the error of the profit model conditioned on the dependent variable’s density and evaluated the probability of transaction anomalies. Transactions with a probability of lower than 1% were marked as abnormal transactions. However, they did not reveal these security issues through measurements on the public blockchain. NFT transaction security is stage of the NFT ecological process, and they do not refine security issues of this stage either.
In terms of NFT anti-fraud, Chan et al. [20] analyzed and detected fraudulent behaviors in NFT smart contracts using semi-supervised learning and applied the detection results to the NFT social platform DTTD [21]. They evaluated the accuracy of detecting NFT fraud using a wide variety of statistical learning models based on social and categorical data from DTTD. Among the above-described models, LGBM (Light Gradient Boosting Machine) achieves the maximum accuracy of 94.38% on the validation set. Roy et al. [22] tracked 439 Twitter accounts that typically promote fraudulent NFT collectibles through giveaways and play a certain role in 1,028 NFT phishing attacks. As revealed by their findings, most accounts interacting with the above-mentioned promotional activities are bots, rapidly increasing the popularity of fraudulent NFT collectibles by inflating the number of likes, follows, and retweets. Li et al. [23] proposed a Temporal Transaction Aggregation Graph Network (TTAGN) to enhance phishing fraud detection on Ethereum. They identified phishing addresses and achieved 92.8% AUC and 81.6% F1-score on an Ethereum phishing fraud dataset by combining transaction features with common statistical and structural features obtained based on graph neural networks. Wu et al. [24] proposed a method for phishing fraud detection by mining transaction records. They reconstructed a transaction network using the historical transaction records of labeled phishing addresses, constructed features based on transaction amounts and timestamps, and employed one-class support vector machines (SVM) to classify nodes as the normal or phishing nodes. Kim et al. [25] proposed a theft detection system based on the transaction behavior for NFT theft attacks. These researchers extracted 83 million NFT transaction data and 742 thief accounts from the Ethereum blockchain and reported significant differences in transaction and social backgrounds between thieves and regular accounts. The researchers employed graph neural networks to capture the complex relationships in the NFT ecosystem while using several features (e.g., holding time, transaction type, transaction price, user activity time, in/out ratio, and adjacent nodes) to perform theft detection. But, in their NFT anti-fraud study, they do not make a clear distinction between NFT issuer fraud and hacker fraud behavior. Therefore, in this paper, we will construct NFT matrix models based on the behavior of these two different malicious entities.
In general, current research mainly focuses on specific aspects of NFT security, without providing a comprehensive overview of NFT security issues. Thus, the five stages of the NFT lifecycle are summarized based on NFT ecological process, and the security issues regarding the respective stage are elucidated.
In this section, we provide an overview (Fig. 4) of the economy ecological process of NFTs. Specifically, we identified the five stages of the ecological process of NFT lifecycle, as well as the two main actors (i.e., NFT issuser and NFT holder/trader) involved in this ecosystem, and the six infrastructures (i.e., Social Network, NFT storage server, NFT office website, NFT marketplace, NFT DeFi platform and blockchain) serve for this ecosystem. This study is based on the summary of the NFT industry chain surveyed. We tracked and analyzed the issuance and sale processes of dozens of head NFTs in the Ethereum ecosystem (e.g., BAYC [8], Azuki [26], CloneX [27], CoolCats [28]), and their processes are largely consistent with our findings. To facilitate the later elaboration of the NFT ecological process, we clarify the meaning of the two main actors involved in this process. i) NFT issuser: As the owner of the NFT project, the main members of the team include NFT creators or digital artists, marketing and technical staff. ii) NFT holder: As the user of the NFT ecosystem, they participate in NFT ecosystem activities such as trading, staking and socializing.
Figure 4: NFT ecological process
3.1 Ecological Process and Lifecycle
Based on the research of the NFT industrial chain, we give a brief overview of the NFT ecological process. It mainly includes ten processes (i.e., Warm-Up, Collecting, Creation, Deploy, Launch, Minting, Listing, Trading, Staking, and Lending).
Warm-up. NFT issuer announce their NFT project on Social Networks (e.g., Twitter, Instagram), making their NFT digital artwork available to anyone who wants to collect it.
Collecting. NFT holders or traders collect information about NFT projects from Social Networks (e.g.,Twitter, Instagram), and choose which NFT projects are worth collecting or holding.
Creation. NFT issuer create NFT digital artworks and upload them for storage on the Internet (e.g., cloud storage service, InterPlanetary File System (IPFS) [29]), and these storage locations will be linked on the blockchain when NFT issuer deploy NFT smart contract.
Deploy. NFT issuer developed NFT smart contract code which usually includes NFT minting, transfer and approve functions, and then deployed it on the blockchain to tokenize the digital artworks.
Launch. NFT issuer sell their NFTs on their official website. Usually, NFT official website is built on Web3 technology, which means that the back-end exchange logic of the website is based on blockchain technology, and users need to connect to the website through their digital wallet to interact with NFT’s smart contract.
Minting. NFT holders or traders mint NFTs from the issuer’s official website, which means their obtain NFT assets from this primary NFT marketplace. Usually, it is cheaper to get NFTs from this market. Thus, many NFT holders obtain low-cost NFT assets by minting activities.
Listing. NFT holders or traders list their NFT assets for sale on the NFT marketplaces. But,not every NFT assets can be listed and sold on the NFT Marketplace. Some marketplaces (e.g., OpenSea [30], Blur [31], SuperRare [32]) require either the seller or the NFT collection to be verified, So, much more information about NFT collections has been collected (e.g., NFT issuer’s social network accounts, NFT smart contract address, NFT metadata and images) before listing on the marketplaces.
Trading (Bid and Offer). On NFT marketplaces,NFT buyers can place bids or offers for the purchase of NFT assets. Once an NFT offer is accepted, the NFT Marketplace transfers the NFT assets from the seller’s address to the buyer’s address and the crypto payment from the buyer’s address to the seller’s address in a single transaction using the marketplace smart contract protocol.
Staking. NFT holders can deposit their NFTs on an NFT staking platform to receive rewards. Their NFT tokens will be locked on the platform in exchange for staking rewards. For example, Yuga Labs (issuer of BAYC NFTs) provides an official staking service for BAYC NFTs. BAYC holders can stake their NFTs and earn $APE Tokens as rewards [33].
Lending. NFT holders can borrow cryptocurrencies (e.g., ETH, USDC, and DAI) from lenders by using their NFTs as collateral. Usually, there is a risk of automatic liquidation when the NFT market price is less than their loan.
Above, we explained the ecological process of NFTs which includes six infrastructures (i.e., Social Network, NFT storage server, NFT office website, NFT marketplace, NFT DeFi platform and blockchain) that support this ecosystem. The blockchain is one of these infrastructures and serves as a public service. Thus, the criteria for the stages of the lifecycle of NFT are based on the interaction between the two main actors (NFT issuer and NFT holder) and the other five infrastructures. Therefore, the NFT lifecycle is divided into five stages (i.e., Release, Deployment, Minting, Circulation, and Derivative). We first provide a brief overview of NFT lifecycle stages (Table 1), and then detailed exposition of each stage.
At this stage, NFT issuers plan and prepare for the issuance of NFTs. The NFT project creators determine the positioning and design of the NFTs, publish project plans and whitepapers, and promote project information through a wide variety of channels (e.g., social network, online advertising, and art exhibitions) to attract potential buyers. Some NFT issuers may also recruit volunteers to promote and publicize the project. In return, the above-described volunteers may receive benefits (e.g., early access to pre-sales, whitelist privileges for minting NFTs, or discounted prices for purchasing NFTs).
The tasks involved in the positioning and design of NFTs are presented as follows. i) Determining the categorization of NFTs, i.e., NFTs can be categorized based on classifications provided by major NFT trading platforms (e.g., OpenSea), including Art, Gaming, Memberships, Profile Pictures (PFPs), Photography, Domain Names, Music, Sports, and Virtual Worlds. ii) Defining the basic attributes of NFTs, including determining the name, issuance quantity, description, appearance, and other relevant details of the NFTs.
The tasks involved in promotion and marketing are elucidated as follows. i) Building an official website: NFT issuers establish an official website that serves as an entry point for users to connect their wallets and mint NFTs. ii) Operating official social network accounts: The project creators actively manage official social network accounts, primarily on platforms (e.g., Twitter and YouTube) to share project updates, attract potential buyers, gain followers, and generate buzz for the project. iii) Managing online communities: NFT issuers engage with potential buyers via instant messaging platforms (e.g., Discord and Telegram), where they can directly interact with potential buyers and solve their questions while providing updates on the project’s progress.
At this stage, the NFT issuers complete the following tasks. i) Uploading digital artworks as images, audio, or videos to Internet storage, i.e., the NFT issuers upload the digital artworks to online storage platforms. ii) Creating NFT metadata and associating it with the NFT digital artworks, i.e., the project creators create NFT metadata, which covers the storage link of the digital artwork, descriptions, attributes, and other relevant information. iii) Writing and deploying the NFT smart contract on the blockchain, i.e., the NFT issuers develop the NFT smart contract code while deploying it on the selected blockchain network.
To enhance the entertainment, some project creators may release their NFTs as blind boxes. Thus, during the deployment stage, the uploaded NFT digital artwork link and NFT metadata are typically just placeholders. The practical NFT digital artwork link and NFT metadata are correlated with the NFTs once the project creators “reveal” them.
Notably, the storage of NFTs refers to a vital aspect at the deployment stage. Typically, two modes of storage are available for NFT digital artworks and metadata, which are centralized storage and decentralized storage. In centralized storage, NFTs are generally stored on the project creators’ servers or third-party public cloud services (e.g., Amazon Web Services or Alibaba Cloud). Besides, in decentralized storage, NFTs are typically stored on platforms (e.g., the InterPlanetary File System (IPFS) or the Arweave network).
The minting stage represents the process of converting a digital artwork into an NFT. This stage comprises the generation of an NFT token on the blockchain and the transfer of the NFT token to the wallet address of the first buyer. At the minting stage, the NFT issuers sell the NFT digital artworks on their official website. The first buyers connect their wallets to the official website while interacting with the NFT smart contract to invoke the minting function, such that the NFT transaction is completed. The minting function is typically a custom function with minting capabilities developed by the NFT issuers. Some NFT projects rely on the smart contracts of NFT marketplaces to handle the minting process.
In the above-described process, the first buyer should pay a minting fee. The price of the minting fee is determined by the project creators. Since the NFT is minted, the NFT smart contract generates a unique token ID from a zero address while transferring it to the wallet address of the first buyer. Most NFTs have a limited minting quantity to maintain their scarcity.
At the minting stage, project creators generally use marketing tactics (e.g., setting minting privileges and conducting NFT minting auctions):
• Minting Privileges. NFT issuers are likely to divide the minting process into two or more stages for the control of this process. Typically, there is a presale stage where whitelisted wallet addresses have priority for minting, followed by a public sale stage.
• NFT Minting Auctions. Minting auctions can be performed on the blockchain using different mechanisms (e.g., fixed-price auctions and Dutch auctions). In fixed-price auctions, the seller (project creator) sets a fixed price, usually denominated in ETH, and the NFT buyer should make a one-time payment for the NFT and a portion of the gas fees required for the transaction to be covered in a block. During Dutch auctions, the seller (project creator) sets an initial maximum price, which tends to decline with time till the NFTs are sold out.
The above-mentioned marketing tactics aim to create excitement and engagement among buyers during the minting stage.
At this stage, NFTs flow from the first buyers to the NFT secondary marketplaces, where NFT holders can freely engage in buying and selling activities. NFT secondary marketplaces (e.g., Opensea, Blur, and Lookshare) are decentralized applications (DApps) where users can connect their digital wallets through the web interface of the marketplace. The wallet address is adopted for the interaction with the smart contracts deployed on the NFT marketplace for on-chain transactions.
Types of Trading Operations. NFT marketplaces cover three major types of trading operations: 1) Listing NFTs for sale: NFT holders are enabled to list their NFTs for sale on the marketplace by setting a sale price and duration for the listing. They sign and authorize the marketplace’s smart contract to create the listing. 2) Buying NFTs: NFT buyers are capable of directly purchasing NFTs that are listed for sale on the marketplace. 3) Making Offers: If an NFT is not listed for sale, buyers can make offers to the NFT holders and wait for them to accept the offer, thereby completing the purchase.
NFT Transaction Fees. There are two types of fees regarding NFT transactions: 1) NFT Royalties: NFT projects set a royalty fee, which is a percentage of the transaction price, that is collected on each NFT transaction. Typically, the royalty fee ranges from 2% to 10%. 2) Marketplace Fees: NFT marketplaces charge a fee on each transaction. For instance, Opensea charges a 2.5% fee on the transaction price.
Smart Contracts on NFT Marketplaces. NFT marketplaces are typically built on decentralized trading protocols. For instance, Opensea utilizes the Wyvern protocol and Seaport protocol to ensure transaction security, scalability, and development efficiency.
During the trading stage, NFT holders have the opportunity to sell their NFTs at the desired price, and buyers can explore and acquire NFTs based on their interests and preferences. The secondary marketplaces provide liquidity and a platform for NFT trading activities, facilitating the exchange of NFTs among users.
At this stage, NFT holders can deposit their NFTs into NFT lending platforms as collateral and receive a certain proportion of ERC20 tokens, becoming borrowers on the NFT lending platform. Borrowers are required to pay a certain percentage of interest and adhere to a repayment schedule in the loan term. If the price of the collateralized NFT drops to a point where the loan becomes insolvent, the NFT will be liquidated and seized by the NFT lending platform. For instance, the ParaSpace lending platform provides an asset risk model that includes a calculation formula for the liquidation threshold of a wallet address [37] (Eq. (1)):
where Collaterali in ETH represents the current collateral value (measured in ETH), Liquidation Thresholdi represents the liquidation threshold for the specific quality of collateral. ParaSpace sets different liquidation thresholds for a wide variety of NFTs. For instance, the liquidation threshold for BAYC is set to 79%. Total Collateral in ETH represents the total value of all collateralizable assets (measured in ETH). Accordingly, the liquidation threshold for a wallet address is determined as the weighted average of the liquidation threshold values of all NFT assets held in the address. Prominent NFT lending platforms in the current market comprise BendDAO and NFTFI. The total loan amount in the NFT lending market has exceeded USD $1.5 billion [38], as indicated by the data analysis from Dune Analytics as of June 01, 2023.
The emergence of NFT lending platforms has expanded the utility and versatility of NFTs, such that holders are allowed to unlock the value of their NFT assets while maintaining ownership. Accordingly, an avenue is provided for liquidity generation and capital efficiency in the NFT ecosystem.
The NFT security issues matrix model is developed based on the previously mentioned five stages of the NFT lifecycle and inspired by the ATT&CK [39] framework in cybersecurity. This model categorizes NFT security issues while mapping them to the five stages of the NFT lifecycle (Table 2). Moreover, the security issues are classified into two different categories based on the differentiation of malicious entities (i.e., malicious activities of NFT issuers and hacker attacks). The former adversely affects NFT buyers, while the latter targets either NFT issuers or NFT buyers as victims.
The subsequent parts of the above-mentioned section will analyze and elaborate on the security issues in each of the five stages of the NFT lifecycle.
4.1 Security Issues at the Release Stage
4.1.1 Malicious Activities of NFT Issuers
Malicious activities conducted by NFT issuers in the release stage primarily involve fraudulent tactics aimed at attracting potential NFT buyers. The specific security issues are as follows.
Zombie Followers. These followers refer to false or inactive follower accounts that appear on the official social network platforms of NFT issuers (e.g., Twitter, Discord and Telegram). The above-mentioned zombie accounts have been generally created by automated scripts or bots to increase the number of followers and create a false sense of community activity and reputation for the project owners. Kapoor et al. [40] demonstrated the significant effect of social network on the value of NFTs, confirming the profitability of using zombie followers and similar malicious tactics. Their study analyzed 245,159 tweets posted by 17,155 unique users on Twitter, which were correlated with 62,997 NFT assets worth $19 million on OpenSea. They found that the number of project followers and the username of the blogger significantly affect the value of NFT assets, leading to the development of a predictive model for NFT asset values. Furthermore, Simone et al. [41] revealed a positive correlation between Twitter popularity metrics (e.g., the number of followers and tweets) and NFT trading volume, artwork prices, as well as the number of wallets holding the artwork.
Identity Anonymity. The anonymity of NFT issuers reveals their true intentions and motivations for issuing NFTs, such that their actions and responsibilities are difficult to trace. This anonymity poses certain risks and uncertainties to potential NFT investors. For instance, as revealed by Zagabond, the co-founder of Azuki, they had previously launched three rug pull projects, including one that imitated CryptoPunks, called CryptoPhunk, all under an anonymous identity [42].
False Marketing. NFT issuers engage in deceptive or misleading promotion by publishing false or unrealistic roadmaps, whitepapers, and so forth. To be specific, exaggerated development plans, incorrect timelines, unfulfilled features, or partnerships may be included, so as to mislead NFT investors and community members, create a bullish sentiment around the project, and attract more participation and funds.
Hackers primarily target the social network and community of NFT issuers, leading to the following security issues:
Phishing Attacks. Phishing attacks apply to different stages of the NFT lifecycle. Attackers gain access to the NFT issuer’s social network accounts or Discord server using penetration testing, social engineering, and other methods. They trick NFT holders into visiting phishing websites, where they are prompted to connect their digital wallets and unknowingly authorize the attacker’s address with the ability to transfer their NFTs. Through the ERC721 protocol, attackers can grant themselves approval by exploiting the setApprovalForAll function. For instance, on June 05, 2022, BAYC announced on their official Twitter account that their Discord server had been briefly attacked, resulting in the theft of approximately 200 ETH worth of NFTs. This attack occurred when a community administrator’s account was compromised, and the hacker impersonated the administrator to share a link to a phishing website [43]. At this stage, hackers primarily target accounts holding a significant number of NFT assets or high-value assets. Chen et al. [44] identified a serious privacy issue in the current NFT system, where the address of each NFT owner is stored in plain text. The above-mentioned vulnerability allows hackers to easily acquire information regarding the entire NFT asset portfolio and its value regarding a specific blockchain address, making it easier for them to orchestrate fraudulent activities targeting high-value NFT owners. To address the above-described problem, they proposed a novel trading scheme based on the OpenSea marketplace to conceal the NFT owner’s address during transactions.
Information Gathering. Attackers infiltrate NFT issuer’s communities to gather intelligence for subsequent attacks.
4.2 Security Issues at the Deployment Stage
4.2.1 Malicious Activities of NFT Issuers
Malicious activities by NFT issuers at the deployment stage primarily involve adopting unreasonable techniques to reduce the cost of NFT issuance, such that the usability, security, or reliability of the NFTs are sacrificed. The specific security issues are presented as follows:
Centralized Storage. NFT issuers deploy NFT metadata and media assets to centralized services, which can trigger data loss and affect the availability of NFTs.
Closed-Source Smart Contract. When deploying smart contracts, NFT issuers only deploy the compiled binary files (usually bytecode) to the blockchain network. The closed-source nature of the smart contract code can prevent NFT buyers from assessing its security and reliability.
At this stage, the primary attack method employed by NFT hackers continues to be phishing attacks, primarily occurring between the completion of NFT smart contract deployment by project owners and the announcement of the NFT minting time.
4.3 Security Issues at the Minting Stage
4.3.1 Malicious Activities of NFT Issuers
At this stage, malicious activities by NFT issuers primarily comprise the inclusion of backdoors in the NFT smart contracts and the use of deceptive tactics to create hype around the NFT. The specific security issues are elucidated as follows:
Smart Contract Backdoors. NFT issuers intentionally cover backdoors in the minting function to control NFT mining. Such backdoors comprise unlimited minting and massive reserved NFTs. i) Unlimited Mining. Accordingly, the scarcity of NFTs can be affected, their value can be diluted, and project owners are endowed with the ability to continuously mint and sell NFTs on the secondary market. For instance, the BAYC project faced allegations of an unlimited minting backdoor in their smart contract code, which was later resolved by relinquishing the owner’s permissions [45]. Project owners commonly retain the owner privileges after NFT issuance, such that a risk of potential misuse is posed. ii) Massive Reserved NFTs. NFT issuers reserve considerable NFTs for themselves through private mints or pre-sales to sell them on the secondary market at any time.
Minting Manipulation. NFT issuers use automated scripts to trigger minting events without generating NFTs or paying the minting fees. Consequently, a false impression of high minting activity is created, and NFT buyers, who fear missing out on the opportunity and participate in minting purchases, are deceived.
Airdrop Frauds. NFT issuers airdrop their self-minted NFTs to the wallet addresses of NFT whales or influencers, creating an illusion that the above-described entities also hold the NFTs. Thus, the followers or fans of NFT whales or influencers are deceived to participate in minting and purchasing those NFTs. Moreover, this fraudulent tactic applies to the circulation stage.
At this stage, NFT hackers primarily exploit business vulnerabilities. Typically, NFT offers lower prices for NFT issuance and minting compared with the prices on NFT marketplaces, such that significant profit potential can be created. Attackers often target popular NFTs that have high valuations and liquidity, as it becomes easier for them to offload considerable NFTs.
Automated Batch Minting. In general, NFT smart contracts have a public mint switch that allows anyone to participate in minting. While regular buyers use the official website’s web interface to mint NFTs, the front end control typically covers a countdown timer. Nevertheless, the public mint switch in the smart contract is generally activated in advance. Attackers exploit this time discrepancy and use automated scripts to invoke the public mint function in the smart contract, completing NFT minting. Thus numerous popular NFTs have already completed minting before the official web interface is made available, such that regular buyers are unlikely to purchase the NFTs.
Gas Wars. Under the high demand for minting NFTs that generally exceeds the supply, participants (e.g., regular buyers, attackers, and other participants) engage in bidding wars by paying additional gas fees. This ensures that their transactions are prioritized for inclusion in blockchain blocks, increasing their chances of successfully minting NFTs.
Minting Vulnerabilities. Attackers discover vulnerabilities in the minting function through smart contract code audits or fuzz testing. Examples of vulnerabilities comprise whitelist bypasses and signature replay attacks. i) Whitelist Bypass: Attackers participate in NFT minting without authorization by bypassing whitelist restrictions. Whitelists are mechanisms set up by project owners to limit NFT minting to specific user addresses. For instance, under the BAYC project, the whitelist verification was placed on the official website’s front end, whereas the smart contract only covered timestamp and mint quantity signatures. Attackers can generate signatures using the front end and directly call the smart contract’s mint function to mint NFTs. ii) Signature Replay Attacks: Attackers exploit the replay of already signed transaction information to mint NFTs multiple times. For instance, in the whitelist minting process of an NBA NFT project, an attacker (non-whitelisted) is capable of minting NFTs by duplicating the signature of a whitelisted user. Exploiting this vulnerability, an attacker minted 100 NFTs in one go and made millions of dollars in profit by selling them on the NFT marketplace.
4.4 Security Issues at the Circulation Stage
4.4.1 Malicious Activities of NFT Issuers
At this stage, malicious activities by NFT issuers primarily involve fraudulent activities in manipulating the NFT marketplace (secondary market) to gain additional profits or directly conducting rug pulls. The specific security issues are as follows:
Wash Trading. NFT issuers engage in artificially generated high-volume trading activities to create an illusion of a thriving and active market, attracting more buyers. Wash trading has been reported as a common practice in blockchain for manipulating trading volumes. Victor et al. [46] measured wash trading activities in two popular Ethereum decentralized exchanges, IDEX and EtherDelta. Their research revealed wash trading activities totaling over $159 million, with over 30% of tokens involved in wash trading. Likewise, a significant number of quantity-based trades exist in the NFT market. They have acquired transaction data from 52 leading ERC721 NFT collections on the Ethereum blockchain from January 01, 2018, to November 21, 2021. As revealed by the result of their analysis, approximately 3.93% of the addresses engaged in potential illegal wash trading, accounting for 2.04% of the total transactions and resulting in an increased trading volume of $149.5 million. Notably, intentional trading behavior by NFT holders should be excluded. For instance, NFT trading platforms (e.g., LooksRare [47] and Blur [31] incentivize NFT users) to trade on their platforms by providing rewards in the form of airdropped tokens. Many NFT holders engage in active wash trading to earn the above-mentioned rewards.
Pump and Dump. NFT issuers intentionally inflate the price of NFTs in a short period and then sell off a significant number of NFTs they hold when the price reaches its peak, such that a rapid price decline is triggered.
Nesting Frauds. NFT issuers abandon previous NFTs while launching novel ones in a continuous fraudulent manner. Typically, project owners offer certain benefits to holders of old NFTs (e.g., whitelist privileges for minting novel NFTs). Typically, a nesting pattern involves the successive release of NFT with different concepts. For instance, the Bored Bunny NFT project led to the creation of Bored Bad Bunnies and Machine Bunnies NFTs, all of which were sold at high prices. Lastly, the project owners conduct rug pulls [48], leaving investors empty-handed.
Mixer Withdrawal. NFT issuers use cryptocurrency mixing services (e.g., Tornado Cash) to transfer the proceeds from NFT sales. This behavior commonly serves as a precursor to an exit scam, since using mixer services makes it challenging to trace the cryptocurrency transferred by the project owners. For instance, approximately $1.3 million worth of Ethereum was transferred using Tornado Cash during the Frosties NFT exit scam [49].
Malicious activities by NFT hackers primarily aim at exploiting, defrauding, or profiting from NFT buyers in the NFT marketplace (secondary market). The specific security issues are elucidated as follows:
Counterfeit Fraud. Counterfeit Fraud covers piracy and forgery frauds. i) Piracy Fraud: Attackers duplicate existing popular and high-demand NFT collections. For instance, in April 2023, a U.S. court ruled that the RR/BAYC project infringed on the copyright of Yuga Labs [37]. ii) Forgery Fraud: Attackers create counterfeit artworks resembling the appearance of well-known NFT collections. They produce similar images, names, and descriptions to deceive buyers. For instance, the CryptoPhunks project, which forged CryptoPunks, generated significant trading volume on platforms (e.g., Opensea), with transactions reaching 2,214 ETH, equivalent to approximately $4.14 million [9]. NFT counterfeit fraud has long been a challenging issue. Some scholars have yielded their solutions to protect NFT copyrights. Kripa et al. [50] introduced a copyright protection scheme based on smart contracts and the InterPlanetary File System (IPFS). This scheme is capable of detecting similar images to existing NFTs and preventing their registration. Roberto et al. [51] proposed the CopyrightLY solution, based on blockchain and Semantic Web technologies, which allows creators to declare their NFT copyrights and provide corresponding proofs. Furthermore, they introduced the CLY token and a voting mechanism to address false claims through the crowdsourcing processes.
Floor Trading Arbitrage. Attackers are enabled to monitor the NFT marketplace for sell orders significantly below the floor price due to human errors. They engage in preemptive trading, purchasing the undervalued NFTs and reselling them at higher prices to other bidders. For instance, a small typo in the seller’s input for the sale price can trigger a price difference of tenfold.
Minting Announcement Arbitrage. Attackers are enabled to monitor NFT in the minting process and quickly analyze the updated metadata information released by the project owners. They prioritize acquiring NFTs with high rarity at lower prices on the NFT marketplace (secondary market) before the updated information becomes widely available.
4.5 Security Issues at the Derivative Stage
Generally, NFT issuers do not participate in the derivative stage of NFTs, as the security threats at this stage primarily come from attacks by hackers, particularly in NFT lending platforms. The specific security issues are as follows:
Flash Loan Attacks. Attackers utilize NFT lending platforms to borrow NFTs temporarily, gaining temporary ownership of the NFTs and exploiting this temporary ownership for arbitrage opportunities. For instance, in March 2022, the BAYC project planned to airdrop ApeCoin tokens to BAYC NFT holders based on their instantaneous ownership status. Attackers could leverage NFT flash loans to acquire temporary ownership, borrowing multiple BAYC NFTs on the NFT lending platform (NFTX) and claiming ApeCoin tokens, resulting in a profit of 293 ETH (approximately $820,000) [52].
Oracle Attacks. Attackers manipulate the prices of NFTs by attacking the oracle, causing NFT lending platforms to retrieve NFT prices from the oracle at significantly lower values than the market price. This manipulation triggers forced liquidations of NFTs at lower prices.
Logic Vulnerabilities in Lending Contracts. Design or implementation errors in the smart contracts of certain NFT lending platforms allow attackers to manipulate the contracts through vulnerabilities. For instance, on June 24, 2022, the NFT lending protocol XCarnival was targeted in a vulnerability attack, resulting in a profit of 3,087 ETH (approximately $3.8 million) for the hacker [53]. The specific exploitation comprised the failure of the contract to check whether the xToken address provided by the attacker was whitelisted by the project owner, as well as the lack of validation of the collateral status during borrowing, such that the attacker can be allowed to repeatedly use invalid collateral records for borrowing.
In the above-mentioned section, several key issues are selected from the stages of NFT release, deployment, minting, and circulation based on the NFT security issue matrix for measurements in the Ethereum NFT ecosystem. The above-described measurements aim at revealing and demonstrating the severity of NFT security issues.
At this stage, the primary focus is placed on the channels employed by NFT issuers for promotion on the Internet, including official websites, social network platforms (e.g.,Twitter), and instant messaging tools (e.g., Discord). Three security issues of the NFT issuers from the perspective of anonymity and credibility are revealed, i.e., identity anonymity, zombie followers, as well as fake information. The information channels used by NFT issuers on the Internet are collectively referred to as social network information in this study.
• Anonymity. NFT issuers intentionally withhold personally identifiable information. Its main manifestations on Web2 are that If there is no associated information on social networks and office website of the NFT issuers, it can be determined that the NFT project is anonymously released. This means that there is no access to NFT issuers’ personal and project information on the Internet, and no way to communicate with issuers.
• Credibility. The activity of the issuer’s social network account, particularly the tweets posted and the information on the account, is fraudulent or not. The trustworthiness of social network accounts can be assessed by the reputation score of the account’s zombie followers and spam messages.
Data Collection. OpenSea, the largest NFT marketplace at present [54], collects information such as official website URLs, Twitter accounts, Discord community URLs, etc., before listing an NFT project for trading. Therefore, OpenSea integrates the NFT smart contract information with the NFT issuer’s social network accounts and official website information. For example,the trading page of BAYC NFT on OpenSea [55], the address of the BAYC smart contract (0xbc4ca0eda7647a8ab7c2061c2e118a18a936f13d), the official website (http://www.boredapeyachtclub.com/), the discord address (https://discord.com/invite/3P5K3dzgdB) and the twitter address (https://twitter.com/BoredApeYC) are displayed on this page.This study utilizes OpenSea’s API interface [56] to collect social network information of 35,610 NFT projects that have been listed on OpenSea, forming a dataset of NFT social network information as shown in Table 3.
Indicator Collection. 1) Anonymity. Our measure of anonymity using data from OpenSea (currently the most-traded NFT marketplace) is based on the assumption that OpenSea is able to capture as completely as possible the social network accounts and website addresses of the NFT project. If OpenSea fails to capture this information, the NFT project is considered anonymous. Therefore, we measure anonymity by measuring how many and what percentage of NFT projects traded on OpenSea are not associated with social network account identities and their office website URL addresses. 2) Credibility. Assessing the credibility of social network accounts in the entire NFT industry by utilizing the zombie follower credibility score and fake spam credibility score evaluated through Botometer [57]. Botometer is a web-based program that uses machine learning to classify Twitter accounts as bot or human by looking at features of a profile including friends, social network structure, temporal activity, language and sentiment [58]. Botometer outputs an overall bot score (0–5) along with several other scores (e.g., fake_follower, spammer, and astroturf) that provides a measure of the likelihood that the account is a bot. fake_follower means bots purchased to increase follower counts and spammer means accounts labeled as spambots. Therefore, we chose these two metrics to assess the credibility of the behavior of the NFT issuer side Twitter account. A comparative analysis is conducted with popular Twitter accounts and non-NFT industry Twitter accounts.
Anonymity. Among the social network information of the 35,610 NFTs, a total of 25,050 official website URLs, 24,259 Twitter accounts, and 15,364 Discord community addresses were collected. There were 5,239 NFTs without any associated social network accounts and office website URL, which accounts for over 14.71% of NFTs listed on OpenSea without any collected social network information and their office website URL.
Credibility. The Twitter accounts of the top 4,770 NFTs are obtained based on their trading volume, representing the NFT industry. Subsequently, 400 accounts representing non-NFT industries are selected from Twitter’s recommended popular accounts (e.g., news, finance, IT, and entertainment). As indicated by the result, the distribution of fake_follower scores and spammer scores for NFT industry Twitter accounts exceeds that of non-NFT industry accounts. As depicted in Figs. 5 and 6, Botometer outputs fake_ follower and spammer score (0–5), for presentation purposes, we have multiplied all scores by 10. So, the median fake_ follower score for the non-NFT industry reaches 10, whereas that for the NFT industry is 19. The median spammer score for the non-NFT industry reaches 1, whereas that for the NFT industry is 5. Thus, the proportion of zombie followers and the dissemination of fake spam messages among NFT issuers’ Twitter followers is higher compared with other industries.
Figure 5: Fake_follower score distribution
Figure 6: Spammer score distribution
At this stage, the primary focus is placed on two security issues regarding the deployment of NFTs, i.e., centralized storage of NFTs and the closed-source nature of smart contract code. Das et al. [4] measured the above-mentioned problem based on the number of NFTs, revealing their existence. Besides, it is measured in accordance with the number of NFT projects, and the storage methods are measured in depth. By sorting the NFT projects based on trading volume, the smart contract addresses of the top 9,735 NFT projects are obtained, accounting for approximately 99.42% of the total trading volume in the entire NFT market.
The smart contract ABI (Application Binary Interfaces) information is collected using the API provided by EtherScan, an Ethereum blockchain explorer service. The smart contract addresses receiving a response of “contract source code not verified” are collected. Subsequently, whether the smart contract was deployed using the ERC721 protocol for NFTs is determined based on the method interface information in the ABI. Next, the TokenURI method is adopted to obtain the metadata information of the NFTs. Lastly, the URI where the NFT’s metadata is stored is obtained by parsing the image field in the metadata information.
Unverifiable smart contract code. Out of the 9,735 NFT smart contracts, 559 smart contract codes (approximately 5.74%) are found to be unverifiable, whereas 9,176 smart contract codes are verifiable.
NFT metadata storage methods. From the 9,735 NFT smart contracts, a total of 6,995 smart contracts deployed based on the ERC721 standard are extracted. Table 4 lists the measurement results of their metadata storage:
1) On-chain storage. Approximately 5.02% of the metadata is stored on the Ethereum blockchain, either by encoding the JSON format content of the metadata using BASE64 or directly storing the plaintext on the blockchain.
2) Off-chain storage. Approximately 41.6% of the metadata is stored using the IPFS [29] for decentralized storage. Moreover, 0.44% of the metadata is approximately stored using non-IPFS (specifically Arweave, as discovered during measurement) for decentralized storage.
17.86% of the metadata is stored on the Second-level domain (SLD) of the NFT’s official website. In other words, the metadata URI and the URL of the official website point to the identical SLD. The above-mentioned type of URI is labeled as “Official-SLD.” For instance, for a CloneX NFT with the TokenID of 1, the metadata URI is “https://clonex-assets.rtfkt.com/1,” and the official website is “http://www.rtfkt.com.” Both domains have the SLD “rtfkt.com.” The other type is storing NFT metadata with third-party services. This type of URI is labeled as “Non-Official-SLD.” As indicated by the measurement results, it accounts for approximately 35.08% of the metadata.
Subsequently, the accessibility of the off-chain stored NFT metadata URIs is measured, as shown in Fig. 7. As indicated by the result, the reliability of NFT metadata stored with “Non-Official-SLD” is the lowest, with 31.62% of the URIs being inaccessible. In general, decentralized storage methods appear to be more reliable than centralized storage methods.
Figure 7: Accessibility of the NFT metadata
At this stage, two security issues are measured, concerning the NFT issuer’s control over the NFT smart contract and the distribution of minted tokens. The measurements place a focus on the potential risks of unauthorized minting and fraudulent airdrops.
Control of NFT Smart Contracts. First, from the measured 9,176 verifiable smart contracts’ ABIs mentioned above, the owner () method of the smart contract is called to obtain the owner’s address. If the owner’s address is the Ethereum genesis address (0x0000000000000000000000000000000000000000), it indicates that the NFT issuer has relinquished control of the smart contract; otherwise, the NFT issuer retains control. Next, it is determined whether the NFT smart contract displays a proxy pattern, which allows for upgradability. ERC1967 has been reported as a common standard for proxy pattern smart contracts.
Airdrop Fraud. The distribution of NFTs received through airdrops is measured by analyzing the wallet addresses of NFT holders. Subsequently, a random sample of airdropped NFTs is selected to verify the contract’s security, such that the patterns of NFT airdrop fraud are revealed. Three NFT projects, i.e., BAYC (ranked first in trading volume), mfer (ranked between 30th and 50th in trading volume), and Starlink PixelNauts (ranked outside the top 1000), representing high, medium, and low popularity NFT projects, respectively, are selected. The floor price of NFT transactions is arranged from high to low, and wallet addresses of BAYC holders are considered “NFT Leader” wallet addresses. Airdrop refers to a method of casting NFTs, where NFT tokens are sent to target wallet addresses based on the Ethereum genesis address (0x0000000000000000000000000000000000000000). Thus, all transaction records of NFTs minted by the above-mentioned wallet addresses are obtained. Airdrop transactions and active minting transactions are distinguished using the MethodID. MethodID specifies the first four bytes of the hash value of the function name and parameters involved in the function call. Two common airdrop functions are selected for measurement, i.e., airdrop(address[] addresses, uint256 numberOfTokens) and airdrop(address[] to), with MethodIDs 0xc204642c and 0x729ad39e, respectively.
Control of NFT Smart Contracts. Among the 9,176 NFT smart contracts, only 32 contracts (less than 0.35%) relinquished control. Notably, 898 contracts (9.79%) were implemented using the ERC1967 protocol, such as the Phanta Bear NFT.
Distribution of Airdrop Quantities. A total of 5,715 wallet addresses holding BAYC, 5,462 wallet addresses holding mfer, and 853 wallet addresses holding Starlink PixelNauts were obtained. The box plot distribution of the number of airdropped NFTs per wallet address is shown in Fig. 8. As expected, NFT fraudsters are more inclined to airdrop NFTs to “big V” wallet addresses to exploit the perceived value held by influential individuals, facilitating fraudulent activities.
Figure 8: Distribution of airdrop quantities
From the wallet address (0x47d4f20ae83bcd350105f199f900e6e6104dab6a) with the maximum number of airdropped NFTs, a random sample of 10 airdropped NFTs was selected, and it was found that 8 of them had unverified smart contract code.
In this stage, the security issue of NFT counterfeiting is selected. Das et al. [4] have made some progress in measuring the quantity of NFT counterfeiting from two dimensions: NFT names and NFT images. However, the counterfeiting behavior of NFT issuers on social networks is also an important evaluation indicator. This study focuses on measuring the counterfeiting behavior of NFT issuers on Twitter.
During the circulation stage, the most significant risk for NFT buyers is the possibility of rug-pull or soft rug-pull, where the NFT issuers abandon their commitments. In a decentralized environment, NFT buyers have no means to hold the NFT issuers accountable. In this study, the accessibility and inactivity of the NFT issuers’ social network accounts are used to evaluate the likelihood of the project rug-pull or engaging in soft rug-pull behavior.
In terms of security issues concerning attackers in this phase, the paper also focuses on measuring the problem of NFT counterfeiting. Previous research by Das et al. [4] has explored NFT counterfeiting from the perspectives of NFT names and images. However, it is also crucial to evaluate the counterfeiting behavior of NFT issuers on social networks. Accordingly, this study measures the imitation of NFT issuers’ Twitter accounts.
Accessibility. The accessibility of the NFT issuers’ official website address, Discord community address, and Twitter account is measured based on three different methods. 1) The official website is a HTTP URL address. Therefore, we tested the accessibility of the website using HTTP protocol requests. In the RFC2616 protocol, HTTP HEAD method is often used for testing hypertext links for validity, accessibility, and recent modification [59]. To ensure accuracy and reliability, To ensure accuracy and reliability, we conducted a 2-phase test. An HTTP HEAD query is employed first for the accessibility measurement of the official website quickly. If the response code is Non-200, an HTTP GET query will be performed again (e.g., Some Non-200 response codes that do not mean the website is down. For example, The HTTP 301 Moved Permanently redirect status response code indicates that the requested resource has been definitively moved to the URL given by the Location headers and browser redirects to the new URL). If the response code remains Non-200, it is marked to be inaccessible. 2) The Discord community address is also a HTTP URL address, but the URL host address is Discord server. So we tested the accessibility of the Discord community address based on the HTTP response content. First use HTTP GET query to get the response content of the Discord server, then according to the response content to determine whether the Discord community address is valid or not, if the response content can get the information Discord community name, the number of online users and the size of the group and other information, it means that the Discord community address is accessible, otherwise it will be marked as inaccessible. 3) In terms of the accessibility measurement of Twitter accounts, Tweepy [60], a third-party development library provided by Twitter, is employed to acquire information regarding the NFT issuers’ Twitter accounts. Besides marking accounts as inaccessible if they no longer exist, accounts that have privacy settings blocking access or accounts suspended for violating Twitter rules [61] are marked to be inaccessible.
Inactivity. The inactivity level of NFT issuers on social networks is measured by measuring the time elapsed since their last tweet on their Twitter accounts. The inactivity duration is obtained using Tweepy to retrieve the timestamp of the latest tweet posted by accessible NFT issuer’ Twitter accounts and by determining the difference between the above timestamp and the current UTC timestamp. That is, the timestamp of the moment of collection minus the timestamp of the last tweet is the duration of inactivity. Subsequently, the inactivity duration is converted to days by rounding down to measure the level of inactivity.
NFT Counterfeiting. First, the names of the 24,259 Twitter accounts collected from the previous data are treated as strings. The Ratcliff-Obershelp similarity algorithm [62] is then applied to calculate the pairwise similarity between the strings, (Ratcliff/Obershelp pattern recognition algorithm, is a string-matching algorithm for determining the similarity of two strings. It was developed in 1983 by John W. Ratcliff and John A. Obershelp and published in the Dr. Dobb’s Journal in July 1988 [63]. The main idea of the algorithm is to quantify the similarity between two strings using the Longest Common Subsequence (LCS). The similarity is usually calculated by dividing the length of the LCS by the length of the longer string.) thereby measuring the counterfeiting behavior of NFT issuers on Twitter.
Accessibility. As depicted in Fig. 9, out of the 25,050 official website URLs, 7,643 (30.51%) were inaccessible. Out of the 24,259 Twitter accounts, 3,833 (15.8%) were inaccessible. And out of the 15,364 Discord community addresses, 8,704 (56.65%) were inaccessible.
Figure 9: Accessibility
Inactivity. Fig. 10 presents the cumulative distribution of the inactivity duration of NFT issuers’ Twitter accounts, with the X-axis representing the number of inactive days. Based on the observation from the graph, a conclusion is drawn that 35.17% of Twitter accounts have been inactive for over 90 days, 25.21% have been inactive for over 180 days, and 11.99% have been inactive for over 360 days, approaching a year of inactivity. In terms of the above-described NFT projects, the result suggested that the NFT issuers have already exited the project building and are rug-pull.
Figure 10: Inactivity
NFT Counterfeiting. As indicated by the 24,259 Twitter accounts, 261 pairs of Twitter accounts had a similarity of over 0.92. Next, a random sample of 10 pairs of similar Twitter accounts is selected to verify the similarity between their Twitter homepages and NFT images. The result suggests that seven pairs exhibited a certain visual similarity between their Twitter social network accounts and NFT images. For instance, “0xInvisibleFriends” counterfeiting “InvisibleFriends” had Twitter accounts named “InvsbleFrens” and “InvisibleFriends” with a similarity score of 0.9231. As depicted in Fig. 11, their Twitter homepages appeared similarly, especially since their Twitter avatars are identical. Subsequently, by obtaining the NFT image URI information for their respective smart contract addresses on EtherScan, a comparison suggests that their NFT dynamic images were nearly identical, except for differences in the background color. Fig. 12 depicts an example of a sampled NFT.
Figure 11: Twitter homepage of InvisibleFriends and 0xInvisibleFriends
Figure 12: NFT images from InvisibleFriends and 0xInvisibleFriends
In the above-mentioned section, several key NFT security issues at release, deployment, minting, and circulation stages are measured based on the quantitative and qualitative analysis methods. The above-mentioned stages represent the majority of the lifecycle of NFTs. Only a few blue-chip NFTs will be subjected to the derivative stage, since current NFT decentralized finance platforms only support blue-chip NFTs with relatively high and stable prices to lower financial risks in collateralized lending services. Moreover, the details of staking and lending services in a wide variety of NFT decentralized finance platforms differ. Thus, security issues at this stage are primarily discussed through case analysis, as elaborated in the previous sections.
Since NFT holders are downstream in the entire NFT industry chain and are consumers, NFT holders are in a relatively weak position in this ecosystem and are exposed to a variety of scams and traps, putting their digital assets at risk of loss. In this section, we look at the mitigation of security risks to NFT holders’ digital assets from an NFT lifecycle perspective.
In response to the malicious activities of NFT issuers (e.g., fraud, rug-pull, and backdoors), we offer the following recommendations to mitigate the risks. 1) Social Networking Accounts (e.g., Twitter) NFT Fraud Detection. Assesses the credibility of the social network account behavior of NFT issuers and provides early warning of suspected fraudulent activity. 2) Distributed Storage NFT Assets. It is recommended that NFT issuers add some cost to put NFT metadata and NFT digital artworks into distributed storage to prevent the loss of NFT assets. 3) NFT Smart Contract code backdoor auditing. Current research on smart contract auditing focuses mainly on vulnerabilities, with less research on the logical backdoor of the NFT business intentionally reserved by the NFT issuer. 4) NFT issuers Withdrawal Limitations. Enhancement of ERC721 and other mainstream NFT smart contract standard protocols, which can be gradually unlocked according to the NFT issuer’s whitepaper program to sell NFT for profit, to prevent a one-time withdrawal of all the money and rug-pull.
In response to the malicious activities of NFT hackers (e.g.,Counterfeit, Phishing and Exploit) we offer the following recommendations to mitigate the risks. 1) Counterfeit Detection and Alert. As the NFT industry attracts a large amount of capital investment, some startups in the Web3 field are beginning to pay attention to NFT counterfeiting and provide related detection services, such as Tovera [64], Yakoa [65], Optic [66] and CheckNFT [67], etc. They perform counterfeit detection by collecting NFT images and metadata information from the blockchain. Meanwhile, Opensea, the largest NFT markplaces, also provides real-time online counterfeit detection services [68]. 2) Phishing Attack Prevention. Currently, the industry’s mainstream solution is a browser plug-in for real-time detection of NFT phishing sites, such as PeckShieldAlert [64], Pocket Universe [69], Scam Sniffer [70], etc. 3) Exploit Prevention. In addition to performing smart code audits in advance, another option is to add gas front-running hacker’s transaction in real time. For instance, BlockSec prevented a hacker from stealing $5 million from NFT’s Paraspace lending project. After the hacker was unable to execute the exploit transaction due to low gas,BlockSec detected a hack in real time and executed the front-running as a white hat and took control of the assets [71].
In this study, the NFT ecosystem is analyzed in depth from a holistic perspective. On that basis, the NFT ecological processes are comprehensively overviewed. The NFT lifecycle falls into five stages, i.e., release, deployment, minting, circulation, and derivatives. The respective stage is thoroughly discussed. The matrix addressing NFT security issues is introduced. In this matrix, the issues are categorized based on malicious behavior by project owners and attackers. Furthermore, the security issues in each stage of the NFT lifecycle, as described above, are elucidated. Diverse types of data are collected from various sources, including Social Networks, the Ethereum blockchain and NFT marketplaces, which are then integrated and processed. Subsequently, nine critical NFT security issues are selected from the NFT security issues matrix model for qualitative and quantitative analysis. Next, the severity of the above-mentioned security concerns is confirmed.
Acknowledgement: None.
Funding Statement: The authors received no specific funding for this study.
Author Contributions: The authors confirm contribution to the paper as follows: study conception and design, P.L., X.C. and C.L.; data collection: P.L., C.L. and Z.W.; analysis and interpretation of results, P.L., J.Y. and Z.W.; draft manuscript preparation: P.L., C.L., J.Y. and Z.W. All authors reviewed the results and approved the final version of the manuscript.
Availability of Data and Materials: All data are available from the authors upon request.
Conflicts of Interest: The authors declare that they have no conflicts of interest to report regarding the present study.
References
1. Raj, A., Prakash, S. (2022). A privacy-preserving authentic healthcare monitoring system using blockchain. International Journal of Software Science and Computational Intelligence, 14(1), 1–23. [Google Scholar]
2. Gupta, B. B., Li, K. C., Leung, V. C., Psannis, K. E., Yamaguchi, S. et al. (2021). Blockchain-assisted secure fine-grained searchable encryption for a cloud-based healthcare cyber-physical system. IEEE/CAA Journal of Automatica Sinica, 8(12), 1877–1890. [Google Scholar]
3. Far, S. B., Rad, A. I., Bamakan, S. M. H., Asaar, M. R. (2023). Toward metaverse of everything: Opportunities, challenges, and future directions of the next generation of visual/virtual communications. Journal of Network and Computer Applications, 217, 103675. https://doi.org/10.1016/j.jnca.2023.103675 [Google Scholar] [CrossRef]
4. Das, D., Bose, P., Ruaro, N., Kruegel, C., Vigna, G. (2022). Understanding security issues in the nft ecosystem. In: Yin, H., Stavrou, A., Cremers, C., Shi, E. Proceedings of the 2022 ACM SIGSAC Conference on Computer and Communications Security, Los Angeles, CA, USA. https://doi.org/10.1145/3548606.3559342 [Google Scholar] [CrossRef]
5. Weyl, E. G., Ohlhaver, P., Buterin, V. (2022). Decentralized society: Finding web3’s soul. https://ssrn.com/abstract=4105763 (accessed on 30/06/2023). [Google Scholar]
6. Lian, A. (2022). 27 stats about NFTs in 2022: Who are the big winners. https://cryptoslate.com/27-stats-about-nfts-in-2022-who-are-the-big-winners (accessed on 30/06/2023). [Google Scholar]
7. Craig, T. (2022). Hype, bad art, deception: Pixelmon’s $70m NFT rug unpacked. https://cryptobriefing.com/hype-bad-art-deception-pixelmons-70m-rug-unpacked (accessed on 30/06/2023). [Google Scholar]
8. BAYC (2023). https://boredapeyachtclub.com/ (accessed on 13/07/2023). [Google Scholar]
9. _chopper_ (2021). Cryptophunks. https://opensea.io/collection/crypto-phunks (accessed on 30/06/2023). [Google Scholar]
10. Chen, A. (2022). Asian pop superstar jay chou loses over $450,000 worth of NFTs to scammer. https://en.pingwest.com/a/10019 (accessed on 30/06/2023). [Google Scholar]
11. Castro, D. (2023). NFTs: Us policies and priorities in 2023. https://itif.org/publications/2023/04/24/nfts-us-policies-and-priorities-in-2023/ (accessed on 30/06/2023). [Google Scholar]
12. Ethereum (2023). ERC-721 non-fungible token standard. https://ethereum.org/en/developers/docs/standards/tokens/erc-721/ (accessed on 23/06/2023). [Google Scholar]
13. Ethereum (2023). ERC-1155 multi-token standard. https://ethereum.org/en/developers/docs/standards/tokens/erc-1155/ (accessed on 23/06/2023). [Google Scholar]
14. Wang, Q., Li, R., Wang, Q., Chen, S. (2021). Non-fungible token (NFTOverview, evaluation, opportunities and challenges. arXiv preprint arXiv:2105.07447. [Google Scholar]
15. Gupta, Y., Kumar, J., Reifers, D. A. (2022). Identifying security risks in NFT platforms. arXiv preprint arXiv:2204.01487. [Google Scholar]
16. Wang, Z., Gao, J., Wei, X. (2023). Do NFTs’ owners really possess their assets? A first look at the NFT-to-asset connection fragility. Proceedings of the ACM Web Conference 2023, Austin, TX, USA. https://doi.org/10.1145/3543507.3583281 [Google Scholar] [CrossRef]
17. Wen, X., Wang, Y., Yue, X., Zhu, F., Zhu, M. (2023). Nftdisk: Visual detection of wash trading in NFT markets. Proceedings of the 2023 CHI Conference on Human Factors in Computing Systems, Hamburg, Germany. https://doi.org/10.1145/3544548.3581466 [Google Scholar] [CrossRef]
18. Song, M., Liu, Y., Shah, A., Chava, S. (2023). Abnormal trading detection in the NFT market. arXiv preprint arXiv:2306.04643. [Google Scholar]
19. Pelechrinis, K., Liu, X., Krishnamurthy, P., Babay, A. (2023). Spotting anomalous trades in NFT markets: The case of NBA topshot. PLoS One, 18(6), e0287262. [Google Scholar] [PubMed]
20. Chan, V., Choi, E. (2023). NFT fraud detection system. https://wp.cs.hku.hk/2022/fyp22012 (accessed on 30/06/2023). [Google Scholar]
21. DTTD (2023). DTTD: A mobile-first social wallet enabling web3 for everyone. https://www.dttd.io/ (accessed on 11/07/2023). [Google Scholar]
22. Roy, S. S., Das, D., Bose, P., Kruegel, C., Vigna, G. et al. (2023). Demystifying NFT promotion and phishing scams.arXiv preprint arXiv:2301.09806. [Google Scholar]
23. Li, S., Gou, G., Liu, C., Hou, C., Li, Z. et al. (2022). TTAGN: Temporal transaction aggregation graph network for ethereum phishing scams detection. WWW ’22: The ACM Web Conference 2022, Lyon,France. https://doi.org/10.1145/3485447.3512226 [Google Scholar] [CrossRef]
24. Wu, J., Yuan, Q., Lin, D., You, W., Chen, W. et al. (2020). Who are the phishers? Phishing scam detection on ethereum via network embedding. IEEE Transactions on Systems, Man, and Cybernetics: Systems, 52(2), 1156–1166. [Google Scholar]
25. Kim, H., Cui, J., Jang, E., Lee, C., Lee, Y. et al. (2023). Drainclog: Detecting rogue accounts with illegally-obtained NFTs using classifiers learned on graphs. arXiv preprint arXiv:2301.13577. [Google Scholar]
26. Azuki (2023). https://www.azuki.com/ (accessed on 13/07/2023). [Google Scholar]
27. RTFKT (2023). RTFKT: Clonex NFT avatars. https://clonex.rtfkt.com/ (accessed on 13/07/2023). [Google Scholar]
28. CoolCats (2023). Cool cats NFT. https://coolcatsnft.com/ (accessed on 13/07/2023). [Google Scholar]
29. IPFS (2023). IPFS powers the distributed web. https://ipfs.tech/ (accessed on 11/07/2023). [Google Scholar]
30. OpenSea (2023). https://opensea.io (accessed on 11/07/2023). [Google Scholar]
31. Blur (2023). Blur. https://blur.io/airdrop (accessed on 11/07/2023). [Google Scholar]
32. SuperRare (2023). Superrare. https://superrare.com/ (accessed on 13/07/2023). [Google Scholar]
33. ApeStake (2023). Apecoin staking. https://docs.apestake.io/#/ (accessed on 13/07/2023). [Google Scholar]
34. ParaSpace (2023). https://parax.ai/ (accessed on 13/07/2023). [Google Scholar]
35. NFTX (2023). Buy, sell, and swap NFTs instantly. https://nftx.io/ (accessed on 13/07/2023). [Google Scholar]
36. BendDAO (2023). BendDAO: Web3 data liquidity. https://www.benddao.xyz/ (accessed on 13/07/2023). [Google Scholar]
37. Zaslowsky, D. (2023). Judge hands victory to yuga labs in its battle over trademark for bored ape yacht club NFTs. https://tinyurl.com/2yumdfjz (accessed on 30/06/2023). [Google Scholar]
38. Impossiblefinance (2023). NFT lending aggregated dash. https://dune.com/impossiblefinance/nft-lending-aggregated-dash (accessed on 11/07/2023). [Google Scholar]
39. MITRE (2023). ATT&CK®. https://attack.mitre.org/ (accessed on 11/07/2023). [Google Scholar]
40. Kapoor, A., Guhathakurta, D., Mathur, M., Yadav, R., Gupta, M. et al. (2022). Tweetboost: Influence of social media on NFT valuation. Companion Proceedings of the Web Conference 2022, Lyon, France. https://doi.org/10.1145/3487553.3524642 [Google Scholar] [CrossRef]
41. Casale-Brunet, S., Zichichi, M., Hutchinson, L., Mattavelli, M., Ferretti, S. (2022). The impact of NFT profile pictures within social network communities. GoodIT 2022: ACM International Conference on Information Technology for Social Good, Limassol, Cyprus. https://doi.org/10.1145/3524458.3547230 [Google Scholar] [CrossRef]
42. Zagabond (2022). A builder’s journey. https://mirror.xyz/0x1Cb8332607fba6A780DdE78584AD3BFD1eEB1E40/yG8rI1lpQGLPhZch0kjxYRjKTtA9rAL51zg-ZrURyAc (accessed on 30/06/2023). [Google Scholar]
43. Certik (2022). Bored ape yacht club discord hit with phishing attack. https://www.certik.com/resources/blog/5zQ2MkBTCn6dHd6wl9ZJ7i-bored-ape-yacht-club-discord-hit-with-phishing-attack (accessed on 30/06/2023). [Google Scholar]
44. Chen, Z., Omote, K. (2022). Toward achieving anonymous NFT trading. IEEE Access, 10, 130166–130176. [Google Scholar]
45. Foobar (2022). Tweet. https://twitter.com/0xfoobar/status/1533530083603927040 (accessed on 30/06/2023). [Google Scholar]
46. Victor, F., Weintraud, A. M. (2021). Detecting and quantifying wash trading on decentralized cryptocurrency exchanges. WWW ’21: The WebConference 2021, Ljubljana, Slovenia. https://doi.org/10.1145/3442381.3449824 [Google Scholar] [CrossRef]
47. LooksRare (2023). Rewards hub. https://looksrare.org/rewards (accessed on 11/07/2023). [Google Scholar]
48. A., S. (2022). Bored bunny NFT project exposed as a slow rug, $21m stolen from investors. https://www.bitdegree.org/crypto/news/bored-bunny-nft-creators-snatch-21-million-from-slow-rug-pull (accessed on 30/06/2023). [Google Scholar]
49. Pimentel, B. (2022). Anatomy of an NFT art scam: How the frosties rug pull went down. https://www.protocol.com/fintech/frosties-nft-rug-pull (accessed on 30/06/2023). [Google Scholar]
50. Kripa, M., Nidhin Mahesh, A., Ramaguru, R., Amritha, P. P. (2021). Blockchain framework for social media drm based on secret sharing. In: Senjyu, T., Mahalle, P. N., Perumal, T., Joshi, A. Information andcommunication technology for intelligent systems. Singapore: Springer Singapore. [Google Scholar]
51. García, R., Cediel, A., Teixidó, M., Gil, R. (2022). Semantics and non-fungible tokens for copyright management on the metaverse and beyond. https://doi.org/10.48550/arXiv.2208.14174.2208.14174 [Google Scholar]
52. Cybabo (2022). Bayc apecoin suffers $800k flash loan “attack” during air-drop. https://www.cybavo.com/blog/apecoin-bayc-airdrop-flash-loan-exploit/ (accessed on 30/06/2023). [Google Scholar]
53. Lunaray (2022). Xcarnival attack analysis. https://medium.com/coinmonks/xcarnival-attack-analysis-1e5722f03a09 (accessed on 30/06/2023). [Google Scholar]
54. Nansen (2022). NFT statistics 2023: Sales, trends, market cap and more. https://www.nansen.ai/guides/nft-statistics-2022 (accessed on 30/06/2023). [Google Scholar]
55. Boredapeyachtclub (2023). Opensea homepage of boredapeyachtclub. https://opensea.io/collection/boredapeyachtclub (accessed on 13/07/2023). [Google Scholar]
56. Opensea API overview (2023). https://docs.opensea.io/reference/api-overview (accessed on 13/07/2023). [Google Scholar]
57. OSoMe (2023). Botometer. https://botometer.osome.iu.edu/ (accessed on 30/06/2023). [Google Scholar]
58. Randbotometer (2023). Botometer. https://www.rand.org/research/projects/truth-decay/fighting-disinformation/search/items/botometer.html (accessed on 13/07/2023). [Google Scholar]
59. RFC2616 (2023). https://www.w3.org/Protocols/rfc2616/rfc2616-sec9.html (accessed on 13/07/2023). [Google Scholar]
60. Tweepy (2023). https://www.tweepy.org/ (accessed on 11/07/2023). [Google Scholar]
61. Twitter (2023). The Twitter rules. https://help.twitter.com/en/rules-and-policies/twitter-rules (accessed on 14/07/2023). [Google Scholar]
62. Black, P. E. (2021). Ratcliff/obershelp pattern recognition in dictionary of algorithms and data structures. https://www.nist.gov/dads/HTML/ratcliffObershelp.html (accessed on 30/06/2023). [Google Scholar]
63. Ratcliff, J. W., Metzener, D. E. (1988). Pattern matching: The gestalt approach. Dr. Dobb’s Journal, 13(7), 46. [Google Scholar]
64. Tovera (2023). Fight NFT fraud. https://fnftf.io/ (accessed on 13/07/2023). [Google Scholar]
65. Yakoa (2023). AI-powered ip protection for the blockchain. https://www.yakoa.io/ (accessed on 13/07/2023). [Google Scholar]
66. Optic (2023). Ai safety and authenticity: Defend authenticity and keep humanity safe from misinformation in the age of gen-ai. https://www.optic.xyz/ (accessed on 13/07/2023). [Google Scholar]
67. CheckNFT (2023). The smartest way to analyze collectibles and NFTs to make decisions and earn more. https://checknft.io/ (accessed on 13/07/2023). [Google Scholar]
68. OpenSeaCopymint (2023). Anne fauvre-willis. authenticity on opensea: Updates to verification and copymint prevention. https://opensea.io/blog/announcements/improving-authenticity-on-opensea-updates-to-verification-and-copymint-prevention/ (accessed on 13/07/2023). [Google Scholar]
69. Pocketuniverse (2023). Protect your web3 assets. https://www.pocketuniverse.app/ (accessed on 13/07/2023). [Google Scholar]
70. Scamsniffer (2023). All-in-one web3 anti-scam solution. https://www.scamsniffer.io/ (accessed on 13/07/2023). [Google Scholar]
71. Blocksec-prevents-5-million hack (2023). blocksec-prevents-5-million hack. https://insidebitcoins.com/news/blocksec-prevents-5-million-hack (accessed on 13/07/2023). [Google Scholar]
Cite This Article
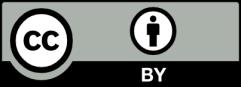