Open Access
ARTICLE
Synergistic Swarm Optimization Algorithm
1 Faculty of Computer Sciences and Informatics, Amman Arab University, Amman, 11953, Jordan
2 Computer Science Department, Al Al-Bayt University, Mafraq, 25113, Jordan
3 Department of Electrical and Computer Engineering, Lebanese American University, Byblos, 13-5053, Lebanon
4 Hourani Center for Applied Scientific Research, Al-Ahliyya Amman University, Amman, 19328, Jordan
5 MEU Research Unit, Middle East University, Amman, 11831, Jordan
6 Applied Science Research Center, Applied Science Private University, Amman, 11931, Jordan
7 School of Computer Sciences, Universiti Sains Malaysia, Pulau Pinang, 11800, Malaysia
8 School of Engineering and Technology, Sunway University Malaysia, Petaling Jaya, 27500, Malaysia
9 Department of Industrial Engineering, College of Engineering, King Saud University, P.O. Box 800, Riyadh, 11421, Saudi Arabia
10 College of Engineering, Al Ain University, Abu Dhabi, 112612, United Arab Emirates
11 Department of Civil and Architectural Engineering, University of Miami, Coral Gables, 1251, USA
12 School of Information Engineering, Sanming University, Sanming, 365004, China
* Corresponding Authors: Laith Abualigah. Email: ,
Computer Modeling in Engineering & Sciences 2024, 139(3), 2557-2604. https://doi.org/10.32604/cmes.2023.045170
Received 19 August 2023; Accepted 17 November 2023; Issue published 11 March 2024
Abstract
This research paper presents a novel optimization method called the Synergistic Swarm Optimization Algorithm (SSOA). The SSOA combines the principles of swarm intelligence and synergistic cooperation to search for optimal solutions efficiently. A synergistic cooperation mechanism is employed, where particles exchange information and learn from each other to improve their search behaviors. This cooperation enhances the exploitation of promising regions in the search space while maintaining exploration capabilities. Furthermore, adaptive mechanisms, such as dynamic parameter adjustment and diversification strategies, are incorporated to balance exploration and exploitation. By leveraging the collaborative nature of swarm intelligence and integrating synergistic cooperation, the SSOA method aims to achieve superior convergence speed and solution quality performance compared to other optimization algorithms. The effectiveness of the proposed SSOA is investigated in solving the 23 benchmark functions and various engineering design problems. The experimental results highlight the effectiveness and potential of the SSOA method in addressing challenging optimization problems, making it a promising tool for a wide range of applications in engineering and beyond. Matlab codes of SSOA are available at: .Keywords
Cite This Article
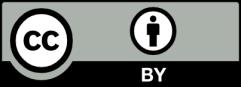