Open Access
ARTICLE
A Robust Framework for Multimodal Sentiment Analysis with Noisy Labels Generated from Distributed Data Annotation
School of Computer Science and Technology, Zhejiang University of Technology, Hangzhou, 310023, China
* Corresponding Author: Bin Cao. Email:
(This article belongs to the Special Issue: Machine Learning Empowered Distributed Computing: Advance in Architecture, Theory and Practice)
Computer Modeling in Engineering & Sciences 2024, 139(3), 2965-2984. https://doi.org/10.32604/cmes.2023.046348
Received 27 September 2023; Accepted 06 December 2023; Issue published 11 March 2024
Abstract
Multimodal sentiment analysis utilizes multimodal data such as text, facial expressions and voice to detect people’s attitudes. With the advent of distributed data collection and annotation, we can easily obtain and share such multimodal data. However, due to professional discrepancies among annotators and lax quality control, noisy labels might be introduced. Recent research suggests that deep neural networks (DNNs) will overfit noisy labels, leading to the poor performance of the DNNs. To address this challenging problem, we present a Multimodal Robust Meta Learning framework (MRML) for multimodal sentiment analysis to resist noisy labels and correlate distinct modalities simultaneously. Specifically, we propose a two-layer fusion net to deeply fuse different modalities and improve the quality of the multimodal data features for label correction and network training. Besides, a multiple meta-learner (label corrector) strategy is proposed to enhance the label correction approach and prevent models from overfitting to noisy labels. We conducted experiments on three popular multimodal datasets to verify the superiority of our method by comparing it with four baselines.Keywords
Cite This Article
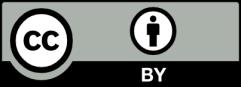