Open Access
ARTICLE
Leveraging Vision-Language Pre-Trained Model and Contrastive Learning for Enhanced Multimodal Sentiment Analysis
1 School of Computer Sciences, Universiti Sains Malaysia, Penang, 11800, Malaysia
2 Jiangxi University of Applied Science, Nanchang, 330000, Jiangxi, China
* Corresponding Author: Jieyu An. Email:
Intelligent Automation & Soft Computing 2023, 37(2), 1673-1689. https://doi.org/10.32604/iasc.2023.039763
Received 15 February 2023; Accepted 13 April 2023; Issue published 21 June 2023
Abstract
Multimodal sentiment analysis is an essential area of research in artificial intelligence that combines multiple modes, such as text and image, to accurately assess sentiment. However, conventional approaches that rely on unimodal pre-trained models for feature extraction from each modality often overlook the intrinsic connections of semantic information between modalities. This limitation is attributed to their training on unimodal data, and necessitates the use of complex fusion mechanisms for sentiment analysis. In this study, we present a novel approach that combines a vision-language pre-trained model with a proposed multimodal contrastive learning method. Our approach harnesses the power of transfer learning by utilizing a vision-language pre-trained model to extract both visual and textual representations in a unified framework. We employ a Transformer architecture to integrate these representations, thereby enabling the capture of rich semantic information in image-text pairs. To further enhance the representation learning of these pairs, we introduce our proposed multimodal contrastive learning method, which leads to improved performance in sentiment analysis tasks. Our approach is evaluated through extensive experiments on two publicly accessible datasets, where we demonstrate its effectiveness. We achieve a significant improvement in sentiment analysis accuracy, indicating the superiority of our approach over existing techniques. These results highlight the potential of multimodal sentiment analysis and underscore the importance of considering the intrinsic semantic connections between modalities for accurate sentiment assessment.Keywords
Cite This Article
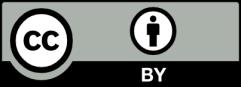