Open Access
ARTICLE
A Random Fusion of Mix3D and PolarMix to Improve Semantic Segmentation Performance in 3D Lidar Point Cloud
1 School of Computer Science and Engineering, Macao University of Science and Technology, Macao, 999078, China
2 School of Computer Science and Artificial Intelligence, Chaohu University, Chaohu, 238000, China
3 Institute of Vehicle Information Control and Network Technology, Hubei University of Automotive Technology, Shiyan, 442002, China
* Corresponding Author: Li Feng. Email:
(This article belongs to the Special Issue: Structural Design and Optimization)
Computer Modeling in Engineering & Sciences 2024, 140(1), 845-862. https://doi.org/10.32604/cmes.2024.047695
Received 14 November 2023; Accepted 08 January 2024; Issue published 16 April 2024
Abstract
This paper focuses on the effective utilization of data augmentation techniques for 3D lidar point clouds to enhance the performance of neural network models. These point clouds, which represent spatial information through a collection of 3D coordinates, have found wide-ranging applications. Data augmentation has emerged as a potent solution to the challenges posed by limited labeled data and the need to enhance model generalization capabilities. Much of the existing research is devoted to crafting novel data augmentation methods specifically for 3D lidar point clouds. However, there has been a lack of focus on making the most of the numerous existing augmentation techniques. Addressing this deficiency, this research investigates the possibility of combining two fundamental data augmentation strategies. The paper introduces PolarMix and Mix3D, two commonly employed augmentation techniques, and presents a new approach, named RandomFusion. Instead of using a fixed or predetermined combination of augmentation methods, RandomFusion randomly chooses one method from a pool of options for each instance or sample. This innovative data augmentation technique randomly augments each point in the point cloud with either PolarMix or Mix3D. The crux of this strategy is the random choice between PolarMix and Mix3D for the augmentation of each point within the point cloud data set. The results of the experiments conducted validate the efficacy of the RandomFusion strategy in enhancing the performance of neural network models for 3D lidar point cloud semantic segmentation tasks. This is achieved without compromising computational efficiency. By examining the potential of merging different augmentation techniques, the research contributes significantly to a more comprehensive understanding of how to utilize existing augmentation methods for 3D lidar point clouds. RandomFusion data augmentation technique offers a simple yet effective method to leverage the diversity of augmentation techniques and boost the robustness of models. The insights gained from this research can pave the way for future work aimed at developing more advanced and efficient data augmentation strategies for 3D lidar point cloud analysis.Keywords
Cite This Article
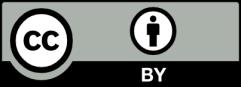