Open Access
ARTICLE
DCFNet: An Effective Dual-Branch Cross-Attention Fusion Network for Medical Image Segmentation
1 School of Computer Science and Engineering, Central South University, Changsha, 410083, China
2 School of Humanities, Central South University, Changsha, 410083, China
3 Xiangya Hospital Central South University, Changsha, 410008, China
4 Changsha Aier Eye Hospital, Changsha, 410015, China
* Corresponding Author: Yalong Xiao. Email:
(This article belongs to the Special Issue: Intelligent Medical Decision Support Systems: Methods and Applications)
Computer Modeling in Engineering & Sciences 2024, 140(1), 1103-1128. https://doi.org/10.32604/cmes.2024.048453
Received 08 December 2023; Accepted 16 January 2024; Issue published 16 April 2024
Abstract
Automatic segmentation of medical images provides a reliable scientific basis for disease diagnosis and analysis. Notably, most existing methods that combine the strengths of convolutional neural networks (CNNs) and Transformers have made significant progress. However, there are some limitations in the current integration of CNN and Transformer technology in two key aspects. Firstly, most methods either overlook or fail to fully incorporate the complementary nature between local and global features. Secondly, the significance of integrating the multi-scale encoder features from the dual-branch network to enhance the decoding features is often disregarded in methods that combine CNN and Transformer. To address this issue, we present a groundbreaking dual-branch cross-attention fusion network (DCFNet), which efficiently combines the power of Swin Transformer and CNN to generate complementary global and local features. We then designed the Feature Cross-Fusion (FCF) module to efficiently fuse local and global features. In the FCF, the utilization of the Channel-wise Cross-fusion Transformer (CCT) serves the purpose of aggregating multi-scale features, and the Feature Fusion Module (FFM) is employed to effectively aggregate dual-branch prominent feature regions from the spatial perspective. Furthermore, within the decoding phase of the dual-branch network, our proposed Channel Attention Block (CAB) aims to emphasize the significance of the channel features between the up-sampled features and the features generated by the FCF module to enhance the details of the decoding. Experimental results demonstrate that DCFNet exhibits enhanced accuracy in segmentation performance. Compared to other state-of-the-art (SOTA) methods, our segmentation framework exhibits a superior level of competitiveness. DCFNet’s accurate segmentation of medical images can greatly assist medical professionals in making crucial diagnoses of lesion areas in advance.Keywords
Cite This Article
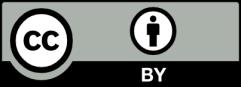