Open Access
ARTICLE
Comparative Analysis of Machine Learning Models for PDF Malware Detection: Evaluating Different Training and Testing Criteria
1 Department of Computer Science, City University of Science and Information Technology, Peshawar, Pakistan
2 Department of Computer Software Engineering, University of Engineering and Technology, Mardan, Pakistan
3 Department of Computer and Software Technology, University of Swat, Swat, Pakistan
4 Department of Computer Science, IQRA National University, Swat, Pakistan
* Corresponding Author: Sarwar Shah Khan. Email:
Journal of Cyber Security 2023, 5, 1-11. https://doi.org/10.32604/jcs.2023.042501
Received 01 June 2023; Accepted 03 August 2023; Issue published 21 August 2023
Abstract
The proliferation of maliciously coded documents as file transfers increase has led to a rise in sophisticated attacks. Portable Document Format (PDF) files have emerged as a major attack vector for malware due to their adaptability and wide usage. Detecting malware in PDF files is challenging due to its ability to include various harmful elements such as embedded scripts, exploits, and malicious URLs. This paper presents a comparative analysis of machine learning (ML) techniques, including Naive Bayes (NB), K-Nearest Neighbor (KNN), Average One Dependency Estimator (A1DE), Random Forest (RF), and Support Vector Machine (SVM) for PDF malware detection. The study utilizes a dataset obtained from the Canadian Institute for Cyber-security and employs different testing criteria, namely percentage splitting and 10-fold cross-validation. The performance of the techniques is evaluated using F1-score, precision, recall, and accuracy measures. The results indicate that KNN outperforms other models, achieving an accuracy of 99.8599% using 10-fold cross-validation. The findings highlight the effectiveness of ML models in accurately detecting PDF malware and provide insights for developing robust systems to protect against malicious activities.Keywords
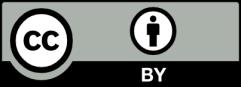