Open Access
ARTICLE
Knowledge Graph Representation Reasoning for Recommendation System
Department of Software, Yunnan University, Kunming, 650500, China
* Corresponding Author: Hao Li. Email:
Journal of New Media 2020, 2(1), 21-30. https://doi.org/10.32604/jnm.2020.09767
Received 17 January 2020; Accepted 31 January 2020; Issue published 14 August 2020
Abstract
In view of the low interpretability of existing collaborative filtering recommendation algorithms and the difficulty of extracting information from content-based recommendation algorithms, we propose an efficient KGRS model. KGRS first obtains reasoning paths of knowledge graph and embeds the entities of paths into vectors based on knowledge representation learning TransD algorithm, then uses LSTM and soft attention mechanism to capture the semantic of each path reasoning, then uses convolution operation and pooling operation to distinguish the importance of different paths reasoning. Finally, through the full connection layer and sigmoid function to get the prediction ratings, and the items are sorted according to the prediction ratings to get the user’s recommendation list. KGRS is tested on the movielens-100k dataset. Compared with the related representative algorithm, including the state-of-the-art interpretable recommendation models RKGE and RippleNet, the experimental results show that KGRS has good recommendation interpretation and higher recommendation accuracy.Keywords
Cite This Article
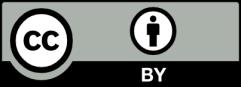