Open Access
ARTICLE
MSC-YOLO: Improved YOLOv7 Based on Multi-Scale Spatial Context for Small Object Detection in UAV-View
1 School of Computer Science and Technology, Hainan University, Haikou, 570228, China
2 Hainan Blockchain Technology Engineering Research Center, Hainan University, Haikou, 570228, China
3 School of Cyberspace Security (School of Cryptology), Hainan University, Haikou, 570228, China
* Corresponding Author: Chengchun Ruan. Email:
Computers, Materials & Continua 2024, 79(1), 983-1003. https://doi.org/10.32604/cmc.2024.047541
Received 08 November 2023; Accepted 27 February 2024; Issue published 25 April 2024
Abstract
Accurately identifying small objects in high-resolution aerial images presents a complex and crucial task in the field of small object detection on unmanned aerial vehicles (UAVs). This task is challenging due to variations in UAV flight altitude, differences in object scales, as well as factors like flight speed and motion blur. To enhance the detection efficacy of small targets in drone aerial imagery, we propose an enhanced You Only Look Once version 7 (YOLOv7) algorithm based on multi-scale spatial context. We build the MSC-YOLO model, which incorporates an additional prediction head, denoted as P2, to improve adaptability for small objects. We replace conventional downsampling with a Spatial-to-Depth Convolutional Combination (CSPDC) module to mitigate the loss of intricate feature details related to small objects. Furthermore, we propose a Spatial Context Pyramid with Multi-Scale Attention (SCPMA) module, which captures spatial and channel-dependent features of small targets across multiple scales. This module enhances the perception of spatial contextual features and the utilization of multiscale feature information. On the Visdrone2023 and UAVDT datasets, MSC-YOLO achieves remarkable results, outperforming the baseline method YOLOv7 by 3.0% in terms of mean average precision (mAP). The MSC-YOLO algorithm proposed in this paper has demonstrated satisfactory performance in detecting small targets in UAV aerial photography, providing strong support for practical applications.Keywords
Cite This Article
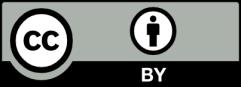