Open Access
ARTICLE
A Real Time Vision-Based Smoking Detection Framework on Edge
1 College of Computer, National University of Defense Technology, Changsha, 410073, China
2 College of Computer, Guizhou University of Finance and Economics, Guiyang, 550025, China
* Corresponding Author: Lei Luo. Email:
Journal on Internet of Things 2020, 2(2), 55-64. https://doi.org/10.32604/jiot.2020.09814
Received 22 January 2020; Accepted 05 June 2020; Issue published 14 September 2020
Abstract
Smoking is the main reason for fire disaster and pollution in petrol station, construction site and warehouse. Existing solutions based on wearable devices and smoking sensors were costly and hard to obtain evidence of smoking in unmanned scenarios. With the developments of closed circuit television (CCTV) system, vision-based methods for object detection, mostly driven by deep learning techniques, were introduced recently. However, the massive GPU computing hardware required by the deep learning algorithm made these methods hard to be deployed. This paper aims at solving the smoking detection problem on edge and proposes the solution that has fast detection speed, high accuracy on micro-objects and low computing budget, i.e., it could be deployed on the edge device such as NVIDIA JETSON TX2. We designed a new framework named RTVBS based on yolov3 and made a smoking dataset to train our model. We raised several methods to improve detection accuracy during the training step. The validation results show our model has excellent performance in smoking detection.Keywords
Cite This Article
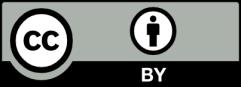