Open Access
ARTICLE
TMCA-Net: A Compact Convolution Network for Monitoring Upper Limb Rehabilitation
1 The School of Computer Science, Nanjing University of Information Science and Technology, Nanjing, 210044, China
2 The School of Computing, Edinburgh Napier University, Edinburgh, EH10 5DT, UK
* Corresponding Author: Zihao Wu. Email:
Journal on Internet of Things 2022, 4(3), 169-181. https://doi.org/10.32604/jiot.2022.040368
Received 15 February 2023; Accepted 24 March 2023; Issue published 12 June 2023
Abstract
This study proposed a lightweight but high-performance convolution network for accurately classifying five upper limb movements of arm, involving forearm flexion and rotation, arm extension, lumbar touch and no reaction state, aiming to monitoring patient’s rehabilitation process and assist the therapist in elevating patient compliance with treatment. To achieve this goal, a lightweight convolution neural network TMCA-Net (Time Multiscale Channel Attention Convolutional Neural Network) is designed, which combines attention mechanism, uses multi-branched convolution structure to automatically extract feature information at different scales from sensor data, and filters feature information based on attention mechanism. In particular, channel separation convolution is used to replace traditional convolution. This reduces the computational complexity of the model, decouples the convolution operation of the time dimension and the cross-channel feature interaction, which is helpful to the target optimization of feature extraction. TMCA-Net shows excellent performance in the upper limb rehabilitation gesture data, achieves 99.11% accuracy and 99.16% F1-score for the classification and recognition of five gestures. Compared with CNN and LSTM network, it achieves 65.62% and 89.98% accuracy in the same task. In addition, on the UCI smartphone public dataset, with the network parameters of one tenth of the current advanced model, the recognition accuracy rate of 95.21% has been achieved, which further proves the light weight and performance characteristics of the model. The clinical significance of this study is to accurately monitor patients’ upper limb rehabilitation gesture by an affordable intelligent model as an auxiliary support for therapists’ decision-making.Keywords
Cite This Article
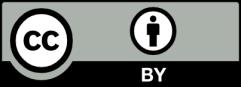