Open Access
ARTICLE
Research on Driver’s Fatigue Detection Based on Information Fusion
1 Harbin Institute of Technology, School of Instrument Science and Engineering, Harbin, 150036, China
2 Department of Industrial and Systems Engineering, The Hong Kong Polytechnic University, Hong Kong, 100872, China
* Corresponding Authors: Qisong Wang. Email: ; Jingxiao Liao. Email:
(This article belongs to the Special Issue: Industrial Big Data and Artificial Intelligence-Driven Intelligent Perception, Maintenance, and Decision Optimization in Industrial Systems)
Computers, Materials & Continua 2024, 79(1), 1039-1061. https://doi.org/10.32604/cmc.2024.048643
Received 13 December 2023; Accepted 04 March 2024; Issue published 25 April 2024
Abstract
Driving fatigue is a physiological phenomenon that often occurs during driving. After the driver enters a fatigued state, the attention is lax, the response is slow, and the ability to deal with emergencies is significantly reduced, which can easily cause traffic accidents. Therefore, studying driver fatigue detection methods is significant in ensuring safe driving. However, the fatigue state of actual drivers is easily interfered with by the external environment (glasses and light), which leads to many problems, such as weak reliability of fatigue driving detection. Moreover, fatigue is a slow process, first manifested in physiological signals and then reflected in human face images. To improve the accuracy and stability of fatigue detection, this paper proposed a driver fatigue detection method based on image information and physiological information, designed a fatigue driving detection device, built a simulation driving experiment platform, and collected facial as well as physiological information of drivers during driving. Finally, the effectiveness of the fatigue detection method was evaluated. Eye movement feature parameters and physiological signal features of drivers’ fatigue levels were extracted. The driver fatigue detection model was trained to classify fatigue and non-fatigue states based on the extracted features. Accuracy rates of the image, electroencephalogram (EEG), and blood oxygen signals were 86%, 82%, and 71%, separately. Information fusion theory was presented to facilitate the fatigue detection effect; the fatigue features were fused using multiple kernel learning and typical correlation analysis methods to increase the detection accuracy to 94%. It can be seen that the fatigue driving detection method based on multi-source feature fusion effectively detected driver fatigue state, and the accuracy rate was higher than that of a single information source. In summary, fatigue driving monitoring has broad development prospects and can be used in traffic accident prevention and wearable driver fatigue recognition.Keywords
Cite This Article
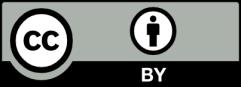