Open Access
ARTICLE
Perpendicular-Cutdepth: Perpendicular Direction Depth Cutting Data Augmentation Method
1 Anhui Provincial Engineering Laboratory of Big Data Technology Application for Urban Infrastructure, School of Artificial Intelligence and Big Data, Hefei University, Hefei, 230601, China
2 Institute of Applied Optimization, School of Artificial Intelligence and Big Data, Hefei University, Hefei, 230601, China
* Corresponding Author: Xiaofeng Wang. Email:
Computers, Materials & Continua 2024, 79(1), 927-941. https://doi.org/10.32604/cmc.2024.048889
Received 21 December 2023; Accepted 28 February 2024; Issue published 25 April 2024
Abstract
Depth estimation is an important task in computer vision. Collecting data at scale for monocular depth estimation is challenging, as this task requires simultaneously capturing RGB images and depth information. Therefore, data augmentation is crucial for this task. Existing data augmentation methods often employ pixel-wise transformations, which may inadvertently disrupt edge features. In this paper, we propose a data augmentation method for monocular depth estimation, which we refer to as the Perpendicular-Cutdepth method. This method involves cutting real-world depth maps along perpendicular directions and pasting them onto input images, thereby diversifying the data without compromising edge features. To validate the effectiveness of the algorithm, we compared it with existing convolutional neural network (CNN) against the current mainstream data augmentation algorithms. Additionally, to verify the algorithm’s applicability to Transformer networks, we designed an encoder-decoder network structure based on Transformer to assess the generalization of our proposed algorithm. Experimental results demonstrate that, in the field of monocular depth estimation, our proposed method, Perpendicular-Cutdepth, outperforms traditional data augmentation methods. On the indoor dataset NYU, our method increases accuracy from 0.900 to 0.907 and reduces the error rate from 0.357 to 0.351. On the outdoor dataset KITTI, our method improves accuracy from 0.9638 to 0.9642 and decreases the error rate from 0.060 to 0.0598.Keywords
Cite This Article
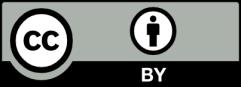