Open Access
ARTICLE
RoGRUT: A Hybrid Deep Learning Model for Detecting Power Trapping in Smart Grids
1 Center of Excellence in Information Assurance (CoEIA), King Saud University, Riyadh, 11543, Saudi Arabia
2 College of Computer & Information Sciences, King Saud University, Riyadh, 11543, Saudi Arabia
* Corresponding Author: Farah Mohammad. Email:
(This article belongs to the Special Issue: Trends in Machine Learning and Internet of Things for Industrial Applications)
Computers, Materials & Continua 2024, 79(2), 3175-3192. https://doi.org/10.32604/cmc.2023.042873
Received 15 June 2023; Accepted 21 October 2023; Issue published 15 May 2024
Abstract
Electricity theft is a widespread non-technical issue that has a negative impact on both power grids and electricity users. It hinders the economic growth of utility companies, poses electrical risks, and impacts the high energy costs borne by consumers. The development of smart grids is crucial for the identification of power theft since these systems create enormous amounts of data, including information on client consumption, which may be used to identify electricity theft using machine learning and deep learning techniques. Moreover, there also exist different solutions such as hardware-based solutions to detect electricity theft that may require human resources and expensive hardware. Computer-based solutions are presented in the literature to identify electricity theft but due to the dimensionality curse, class imbalance issue and improper hyper-parameter tuning of such models lead to poor performance. In this research, a hybrid deep learning model abbreviated as RoGRUT is proposed to detect electricity theft as a malicious and non-malicious activity. The key steps of the RoGRUT are data preprocessing that covers the problem of class imbalance, feature extraction and final theft detection. Different advanced-level models like RoBERTa is used to address the curse of dimensionality issue, the near miss for class imbalance, and transfer learning for classification. The effectiveness of the RoGRUT is evaluated using the dataset from actual smart meters. A significant number of simulations demonstrate that, when compared to its competitors, the RoGRUT achieves the best classification results. The performance evaluation of the proposed model revealed exemplary results across various metrics. The accuracy achieved was 88%, with precision at an impressive 86% and recall reaching 84%. The F1-Score, a measure of overall performance, stood at 85%. Furthermore, the model exhibited a noteworthy Matthew correlation coefficient of 78% and excelled with an area under the curve of 91%.Keywords
Cite This Article
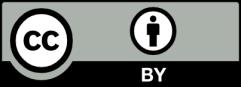