Open Access
ARTICLE
Artificial Neural Network Methods for the Solution of Second Order Boundary Value Problems
Institute of Structural Mechanics, Bauhaus-Universität Weimar, 99423, Weimar, Germany.
School of Civil & Environmental Engineering, University of New South Wales, Sydney, Australia.
Department of Computer Engineering, College of Computer and Information Sciences, King Saud University, Riyadh, Saudi Arabia.
* Corresponding Author: Timon Rabczuk. Email: .
Computers, Materials & Continua 2019, 59(1), 345-359. https://doi.org/10.32604/cmc.2019.06641
Abstract
We present a method for solving partial differential equations using artificial neural networks and an adaptive collocation strategy. In this procedure, a coarse grid of training points is used at the initial training stages, while more points are added at later stages based on the value of the residual at a larger set of evaluation points. This method increases the robustness of the neural network approximation and can result in significant computational savings, particularly when the solution is non-smooth. Numerical results are presented for benchmark problems for scalar-valued PDEs, namely Poisson and Helmholtz equations, as well as for an inverse acoustics problem.Keywords
Cite This Article
Citations
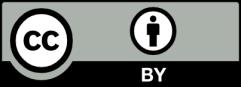