Open Access
ARTICLE
Forecasting Damage Mechanics By Deep Learning
1 CIRTech Institute, Ho Chi Minh City University of Technology (HUTECH), Ho Chi Minh City, Vietnam.
2 Department of Architectural Engineering, Sejong University, 209 Neungdong-ro, Gwangjin-gu, Seoul, Korea.
3 Institute of Structural Mechanics, Bauhaus-Universität Weimar, 99423, Weimar, Germany.
4 Department of Physical Therapy, Graduate Institute of Rehabilitation Science, China Medical University, Taichung, 40402, Taiwan.
* Corresponding Author: Hung Nguyen-Xuan. Email:
Computers, Materials & Continua 2019, 61(3), 951-977. https://doi.org/10.32604/cmc.2019.08001
Abstract
We in this paper exploit time series algorithm based deep learning in forecasting damage mechanics problems. The methodologies that are able to work accurately for less computational and resolving attempts are a significant demand nowadays. Relied on learning an amount of information from given data, the long short-term memory (LSTM) method and multi-layer neural networks (MNN) method are applied to predict solutions. Numerical examples are implemented for predicting fracture growth rates of L-shape concrete specimen under load ratio, single-edge-notched beam forced by 4-point shear and hydraulic fracturing in permeable porous media problems such as storage-toughness fracture regime and fracture-height growth in Marcellus shale. The predicted results by deep learning algorithms are well-agreed with experimental data.Keywords
Cite This Article
Citations
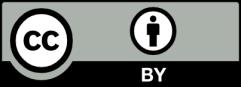