Open Access
ARTICLE
Ensemble Machine Learning Based Identification of Pediatric Epilepsy
1 Department of Computer Science, College of Computer Science and Information Technology, Imam Abdulrahman Bin Faisal University, Dammam, 31441, Saudi Arabia
2 Faculty of Computing, Riphah School of Computing & Innovation, Riphah International University, Lahore, Pakistan
* Corresponding Author: Muhammad Adnan Khan. Email:
Computers, Materials & Continua 2021, 68(1), 149-165. https://doi.org/10.32604/cmc.2021.015976
Received 16 December 2020; Accepted 18 January 2021; Issue published 22 March 2021
Abstract
Epilepsy is a type of brain disorder that causes recurrent seizures. It is the second most common neurological disease after Alzheimer’s. The effects of epilepsy in children are serious, since it causes a slower growth rate and a failure to develop certain skills. In the medical field, specialists record brain activity using an Electroencephalogram (EEG) to observe the epileptic seizures. The detection of these seizures is performed by specialists, but the results might not be accurate due to human errors; therefore, automated detection of epileptic pediatric seizures might be the optimal solution. This paper investigates the detection of epileptic seizures by applying supervised machine learning techniques. The techniques applied on the data of patients with ages seven years and below from children’s hospital boston massachusetts institute of technology (CHB-MIT) scalp EEG database of epileptic pediatric signals. A group of Naïve Bayes (NB), Support vector machine (SVM), Logistic regression (LR), k-nearest neighbor (KNN), Linear discernment (LD), Decision tree (DT), and ensemble learning methods were applied to the classification process. The results demonstrated the outperformance of the present study by achieving 100% for all parameters using the Ensemble learning model in contrast to state-of-the-art studies in the literature. Similarly, the SVM model achieved performance with 98.3% for sensitivity, 97.7% for specificity, and 98% for accuracy. The results of the LD and LR models reveal the lower performance i.e., the sensitivity at 66.9%–68.9%, specificity at 73.5%–77.1%, and accuracy at 70.2%–73%.Keywords
Cite This Article
S. Majed Alotaibi, A. , M. Imran Basheer and M. Adnan Khan, "Ensemble machine learning based identification of pediatric epilepsy," Computers, Materials & Continua, vol. 68, no.1, pp. 149–165, 2021. https://doi.org/10.32604/cmc.2021.015976Citations
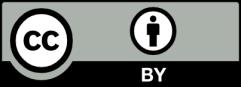