Open Access
ARTICLE
HLR-Net: A Hybrid Lip-Reading Model Based on Deep Convolutional Neural Networks
1 Department of Computers and Control Engineering, Faculty of Engineering, Tanta University, Tanta, 37133, Egypt
2 Department of Information Technology, College of Computer, Qassim University, Buraydah, 51452, Saudi Arabia
* Corresponding Author: Dina M. Ibrahim. Email:
Computers, Materials & Continua 2021, 68(2), 1531-1549. https://doi.org/10.32604/cmc.2021.016509
Received 04 January 2021; Accepted 17 February 2021; Issue published 13 April 2021
Abstract
Lip reading is typically regarded as visually interpreting the speaker’s lip movements during the speaking. This is a task of decoding the text from the speaker’s mouth movement. This paper proposes a lip-reading model that helps deaf people and persons with hearing problems to understand a speaker by capturing a video of the speaker and inputting it into the proposed model to obtain the corresponding subtitles. Using deep learning technologies makes it easier for users to extract a large number of different features, which can then be converted to probabilities of letters to obtain accurate results. Recently proposed methods for lip reading are based on sequence-to-sequence architectures that are designed for natural machine translation and audio speech recognition. However, in this paper, a deep convolutional neural network model called the hybrid lip-reading (HLR-Net) model is developed for lip reading from a video. The proposed model includes three stages, namely, pre-processing, encoder, and decoder stages, which produce the output subtitle. The inception, gradient, and bidirectional GRU layers are used to build the encoder, and the attention, fully-connected, activation function layers are used to build the decoder, which performs the connectionist temporal classification (CTC). In comparison with the three recent models, namely, the LipNet model, the lip-reading model with cascaded attention (LCANet), and attention-CTC (A-ACA) model, on the GRID corpus dataset, the proposed HLR-Net model can achieve significant improvements, achieving the CER of 4.9%, WER of 9.7%, and Bleu score of 92% in the case of unseen speakers, and the CER of 1.4%, WER of 3.3%, and Bleu score of 99% in the case of overlapped speakers.
Keywords
Cite This Article
Citations
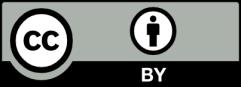