Open Access
ARTICLE
UFC-Net with Fully-Connected Layers and Hadamard Identity Skip Connection for Image Inpainting
1 Korea Electronics Technology Institute, Seongnam, 13488, Korea
2 School of Electrical Engineering, Korea University, Seoul, 02841, Korea
* Corresponding Author: Eenjun Hwang. Email:
(This article belongs to the Special Issue: Machine Learning-based Intelligent Systems: Theories, Algorithms, and Applications)
Computers, Materials & Continua 2021, 68(3), 3447-3463. https://doi.org/10.32604/cmc.2021.017633
Received 01 February 2021; Accepted 05 March 2021; Issue published 06 May 2021
Abstract
Image inpainting is an interesting technique in computer vision and artificial intelligence for plausibly filling in blank areas of an image by referring to their surrounding areas. Although its performance has been improved significantly using diverse convolutional neural network (CNN)-based models, these models have difficulty filling in some erased areas due to the kernel size of the CNN. If the kernel size is too narrow for the blank area, the models cannot consider the entire surrounding area, only partial areas or none at all. This issue leads to typical problems of inpainting, such as pixel reconstruction failure and unintended filling. To alleviate this, in this paper, we propose a novel inpainting model called UFC-net that reinforces two components in U-net. The first component is the latent networks in the middle of U-net to consider the entire surrounding area. The second component is the Hadamard identity skip connection to improve the attention of the inpainting model on the blank areas and reduce computational cost. We performed extensive comparisons with other inpainting models using the Places2 dataset to evaluate the effectiveness of the proposed scheme. We report some of the results.Keywords
Cite This Article
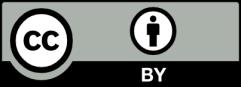