Open Access
ARTICLE
Enhanced Deep Autoencoder Based Feature Representation Learning for Intelligent Intrusion Detection System
College of Computer Engineering and Sciences, Prince Sattam Bin Abdulaziz University, AlKharj, Saudi Arabia
* Corresponding Author: Thavavel Vaiyapuri. Email:
(This article belongs to the Special Issue: Machine Learning-based Intelligent Systems: Theories, Algorithms, and Applications)
Computers, Materials & Continua 2021, 68(3), 3271-3288. https://doi.org/10.32604/cmc.2021.017665
Received 02 February 2021; Accepted 06 March 2021; Issue published 06 May 2021
Abstract
In the era of Big data, learning discriminant feature representation from network traffic is identified has as an invariably essential task for improving the detection ability of an intrusion detection system (IDS). Owing to the lack of accurately labeled network traffic data, many unsupervised feature representation learning models have been proposed with state-of-the-art performance. Yet, these models fail to consider the classification error while learning the feature representation. Intuitively, the learnt feature representation may degrade the performance of the classification task. For the first time in the field of intrusion detection, this paper proposes an unsupervised IDS model leveraging the benefits of deep autoencoder (DAE) for learning the robust feature representation and one-class support vector machine (OCSVM) for finding the more compact decision hyperplane for intrusion detection. Specially, the proposed model defines a new unified objective function to minimize the reconstruction and classification error simultaneously. This unique contribution not only enables the model to support joint learning for feature representation and classifier training but also guides to learn the robust feature representation which can improve the discrimination ability of the classifier for intrusion detection. Three set of evaluation experiments are conducted to demonstrate the potential of the proposed model. First, the ablation evaluation on benchmark dataset, NSL-KDD validates the design decision of the proposed model. Next, the performance evaluation on recent intrusion dataset, UNSW-NB15 signifies the stable performance of the proposed model. Finally, the comparative evaluation verifies the efficacy of the proposed model against recently published state-of-the-art methods.Keywords
Cite This Article
Citations
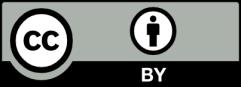