Open Access
ARTICLE
Sentiment Analysis of Short Texts Based on Parallel DenseNet
1 Nanjing University of Information Science & Technology, Nanjing, 210044, China
2 State Grid Hunan Electric Power Company Limited Research Institute, Changsha, 410007, China
3 Waterford Institute of Technology, Waterford, X91 K0EK, Ireland
* Corresponding Author: Jin Han. Email:
Computers, Materials & Continua 2021, 69(1), 51-65. https://doi.org/10.32604/cmc.2021.016920
Received 15 January 2021; Accepted 04 March 2021; Issue published 04 June 2021
Abstract
Text sentiment analysis is a common problem in the field of natural language processing that is often resolved by using convolutional neural networks (CNNs). However, most of these CNN models focus only on learning local features while ignoring global features. In this paper, based on traditional densely connected convolutional networks (DenseNet), a parallel DenseNet is proposed to realize sentiment analysis of short texts. First, this paper proposes two novel feature extraction blocks that are based on DenseNet and a multi-scale convolutional neural network. Second, this paper solves the problem of ignoring global features in traditional CNN models by combining the original features with features extracted by the parallel feature extraction block, and then sending the combined features into the final classifier. Last, a model based on parallel DenseNet that is capable of simultaneously learning both local and global features of short texts and shows better performance on six different databases compared to other basic models is proposed.Keywords
Cite This Article
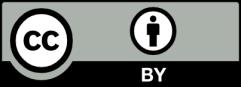