Open Access
ARTICLE
Outlier Detection of Mixed Data Based on Neighborhood Combinatorial Entropy
1 School of Artificial Intelligence, Nanjing University of Information Science and Technology, Nanjing, 210044, China
2 Southern Marine Science and Engineering Guangdong Laboratory (Zhuhai), Zhuhai, 519080, China
3 School of Computer and Software, Nanjing University of Information Science and Technology, Nanjing, 210044, China
4 International Business Machines Corporation (IBM), New York, 10504, USA
* Corresponding Author: Lina Wang. Email:
Computers, Materials & Continua 2021, 69(2), 1765-1781. https://doi.org/10.32604/cmc.2021.017516
Received 02 February 2021; Accepted 10 April 2021; Issue published 21 July 2021
Abstract
Outlier detection is a key research area in data mining technologies, as outlier detection can identify data inconsistent within a data set. Outlier detection aims to find an abnormal data size from a large data size and has been applied in many fields including fraud detection, network intrusion detection, disaster prediction, medical diagnosis, public security, and image processing. While outlier detection has been widely applied in real systems, its effectiveness is challenged by higher dimensions and redundant data attributes, leading to detection errors and complicated calculations. The prevalence of mixed data is a current issue for outlier detection algorithms. An outlier detection method of mixed data based on neighborhood combinatorial entropy is studied to improve outlier detection performance by reducing data dimension using an attribute reduction algorithm. The significance of attributes is determined, and fewer influencing attributes are removed based on neighborhood combinatorial entropy. Outlier detection is conducted using the algorithm of local outlier factor. The proposed outlier detection method can be applied effectively in numerical and mixed multidimensional data using neighborhood combinatorial entropy. In the experimental part of this paper, we give a comparison on outlier detection before and after attribute reduction. In a comparative analysis, we give results of the enhanced outlier detection accuracy by removing the fewer influencing attributes in numerical and mixed multidimensional data.Keywords
Cite This Article
Citations
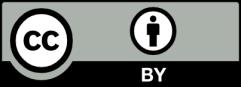